Diagnosis of suspicious pigmented lesions in specialist settings with artificial intelligence
The Lancet: Digital Health(2023)
摘要
The evidence base for the accuracy of artificial intelligence (AI) algorithms in dermatology is growing exponentially, but it is limited by methodological shortcomings in algorithm development and a lack of external validation.1Nagendran M Chen Y Lovejoy CA et al.Artificial intelligence versus clinicians: systematic review of design, reporting standards, and claims of deep learning studies.BMJ. 2020; 368: m689Crossref PubMed Scopus (412) Google Scholar, 2Haggenmüller S Maron RC Hekler A et al.Skin cancer classification via convolutional neural networks: systematic review of studies involving human experts.Eur J Cancer. 2021; 156: 202-216Summary Full Text Full Text PDF PubMed Scopus (75) Google Scholar, 3Liu X Faes L Kale AU et al.A comparison of deep learning performance against health-care professionals in detecting diseases from medical imaging: a systematic review and meta-analysis.Lancet Digit Health. 2019; 1: e271-e297Summary Full Text Full Text PDF PubMed Scopus (710) Google Scholar Where AI algorithm performance has been evaluated in different populations or settings, results are frequently reported in terms of the discriminative capacity of the tool (eg, area under the receiver operating characteristic curve or accuracy), with little or no attention to model calibrationa. Although there is an increasing focus on the comparative accuracy of AI algorithms versus clinicians, many studies are based on retrospectively collected data and built in artificial conditions, thus not adequately reflecting real-life clinical settings,1Nagendran M Chen Y Lovejoy CA et al.Artificial intelligence versus clinicians: systematic review of design, reporting standards, and claims of deep learning studies.BMJ. 2020; 368: m689Crossref PubMed Scopus (412) Google Scholar with results often favouring the AI algorithm over clinical diagnosis.2Haggenmüller S Maron RC Hekler A et al.Skin cancer classification via convolutional neural networks: systematic review of studies involving human experts.Eur J Cancer. 2021; 156: 202-216Summary Full Text Full Text PDF PubMed Scopus (75) Google Scholar Evidence suggests that when these comparisons are made using out-of-sample external validation data, diagnostic performance of AI algorithms is more likely to be equivalent to clinicians.3Liu X Faes L Kale AU et al.A comparison of deep learning performance against health-care professionals in detecting diseases from medical imaging: a systematic review and meta-analysis.Lancet Digit Health. 2019; 1: e271-e297Summary Full Text Full Text PDF PubMed Scopus (710) Google Scholar Moreover, there is legitimate concern that despite these findings, regulatory approvals have been issued without a requirement for prospective data.4Wu E Wu K Daneshjou R Ouyang D Ho DE Zou J How medical AI devices are evaluated: limitations and recommendations from an analysis of FDA approvals.Nat Med. 2021; 27: 582-584Crossref PubMed Scopus (158) Google Scholar Scott W Menzies and colleagues5Menzies SW Sinz C Menzies M et al.Comparison of humans versus mobile phone-powered artificial intelligence for the diagnosis and management of pigmented skin cancer in secondary care: a multicentre, prospective, diagnostic, clinical trial.Lancet Digit Health. 2023; 5: e679-e691Summary Full Text Full Text PDF Scopus (1) Google Scholar have made a welcome attempt to address this real-life clinical practice evidence gap by prospectively comparing in-person clinical decision making with AI algorithms for the diagnosis of suspicious pigmented skin lesions selected for biopsy or excision in a specialist setting and for the management of individuals at high risk with multiple naevi. In their diagnostic clinical trial, Menzies and colleagues compared their own 7-class AI algorithm and the winning AI diagnostic algorithm of the International Skin Imaging Collaboration (ISIC) 2018 Challenge with the diagnostic and management decisions of specialist (ie, those with a medical qualification related to diagnosing and managing pigmented skin lesions) and novice (ie, unaccredited or accredited trainees) clinicians. The results showed that the diagnostic accuracy of the 7-class AI algorithm (ie, the correct classification of lesion types into seven categories [melanoma, melanocytic naevus, basal cell carcinoma, pigmented actinic keratosis or intraepithelial carcinoma, benign keratotic lesion, benign vascular lesion, and dermatofibroma]; 127 [74%] of 172 lesions correctly classified) was equivalent to that of specialists (125 [73%] lesions correctly classified) and superior to that of novices (90 [52%] lesions correctly classified). The diagnostic accuracy of the ISIC algorithm (105 [61%] lesions correctly classified) was significantly inferior to that of specialists, despite previously showing superiority in a retrospective expert readers study.6Tschandl P Codella N Akay BN et al.Comparison of the accuracy of human readers versus machine-learning algorithms for pigmented skin lesion classification: an open, web-based, international, diagnostic study.Lancet Oncol. 2019; 20: 938-947Summary Full Text Full Text PDF PubMed Scopus (262) Google Scholar Specialists outperformed the 7-class AI algorithm for melanomas (34 [62%] vs 28 [51%] of 55), basal cell carcinomas (27 [100%] vs 25 [93%] of 27), and pigmented actinic keratosis or intraepithelial carcinomas (one [50%] vs none of two), whereas their diagnostic accuracy was inferior to the 7-class AI algorithm for melanocytic naevi (54 [74%] vs 64 [88%] of 73) and benign keratotic lesions (eight [57%] vs nine [64%] of 14). The potential downstream effect of these misclassifications (ie, effect on management decisions) was not evaluated. For the management study, new management algorithms were developed using outputs of the original AI algorithms with different threshold combinations to create a single decision of “dismiss”, “monitor”, or “biopsy”, so that comparison with the clinical decisions could be made. With the exception of two of five algorithms, the AI correct management decision algorithms were inferior to both specialists and novices.5Menzies SW Sinz C Menzies M et al.Comparison of humans versus mobile phone-powered artificial intelligence for the diagnosis and management of pigmented skin cancer in secondary care: a multicentre, prospective, diagnostic, clinical trial.Lancet Digit Health. 2023; 5: e679-e691Summary Full Text Full Text PDF Scopus (1) Google Scholar The authors suggested that a more optimal conversion from the 7-class diagnosis to the management decision might be achievable. Menzies and colleagues are to be commended for doing a robust, prospective study in a real-world environment. Some concerns about data representativeness remain; small lesions (≤3 mm) and non-pigmented lesions were excluded and, importantly, participants were restricted to those with Fitzpatrick I–III skin types. Although these inclusion criteria allow a comparison of results with those from the ISIC datasets, the performance of the AI algorithms to diagnose and manage individuals with Fitzpatrick type IV–V skin types remains unknown and their applicability to a more broadly defined population is unclear. Studies limited to some skin types are a recognised concern for dermatology datasets, because they do not adequately represent minority ethnic groups.7Wen D Khan SM Ji Xu A et al.Characteristics of publicly available skin cancer image datasets: a systematic review.Lancet Digit Health. 2022; 4: e64-e74Summary Full Text Full Text PDF PubMed Scopus (37) Google Scholar, 8Daneshjou R Vodrahalli K Novoa RA et al.Disparities in dermatology AI performance on a diverse, curated clinical image set.Sci Adv. 2022; 8eabq6147Crossref PubMed Scopus (21) Google Scholar The Standing Together group emphasises the importance of inclusivity and fairness in dataset creation and has defined essential criteria, with regard to dataset composition and dataset reporting. This guidance should inform future studies to consider inclusivity and diversity of individuals for whom an AI tool could be used. We highlight concerns about lesion selection, including the fact that all lesions had already been scheduled for biopsy or excision; the potential role of a standalone AI algorithm in such a population is unclear. However, considering the promising results observed, future studies should evaluate interactions between clinicians and AI algorithms in the proposed setting and the resulting effect on clinical decisions. For example, the additional benefit from the AI algorithm used in a more broadly defined population (eg, all lesions referred to secondary care), under the care of novice clinicians, remains uncertain. Regulatory bodies, including the UK Medicines and Healthcare Products Regulatory Agency and the US Food and Drug Administration, highlight the requirement of very specific intended uses for AI technologies, including the population and setting in which the test will be used.9UK GovernmentCrafting an intended purpose in the context of Software as a Medical Device (SaMD).https://www.gov.uk/government/publications/crafting-an-intended-purpose-in-the-context-of-software-as-a-medical-device-samdDate: 2023Date accessed: September 12, 2023Google Scholar Prospective real-life data acquired for the intended use and clinical setting in which AI skin cancer technologies will be deployed are still needed to show effectiveness and safety. Clinicians must engage with AI developers to support these development and validation studies to facilitate greater progress in this field. We declare no competing interests. Comparison of humans versus mobile phone-powered artificial intelligence for the diagnosis and management of pigmented skin cancer in secondary care: a multicentre, prospective, diagnostic, clinical trialThe mobile phone-powered AI technology is simple, practical, and accurate for the diagnosis of suspicious pigmented skin cancer in patients presenting to a specialist setting, although its usage for management decisions requires more careful execution. An AI algorithm that was superior in experimental studies was significantly inferior to specialists in a real-world scenario, suggesting that caution is needed when extrapolating results of experimental studies to clinical practice. Full-Text PDF Open Access
更多查看译文
关键词
lesions,diagnosis,artificial intelligence,specialist settings
AI 理解论文
溯源树
样例
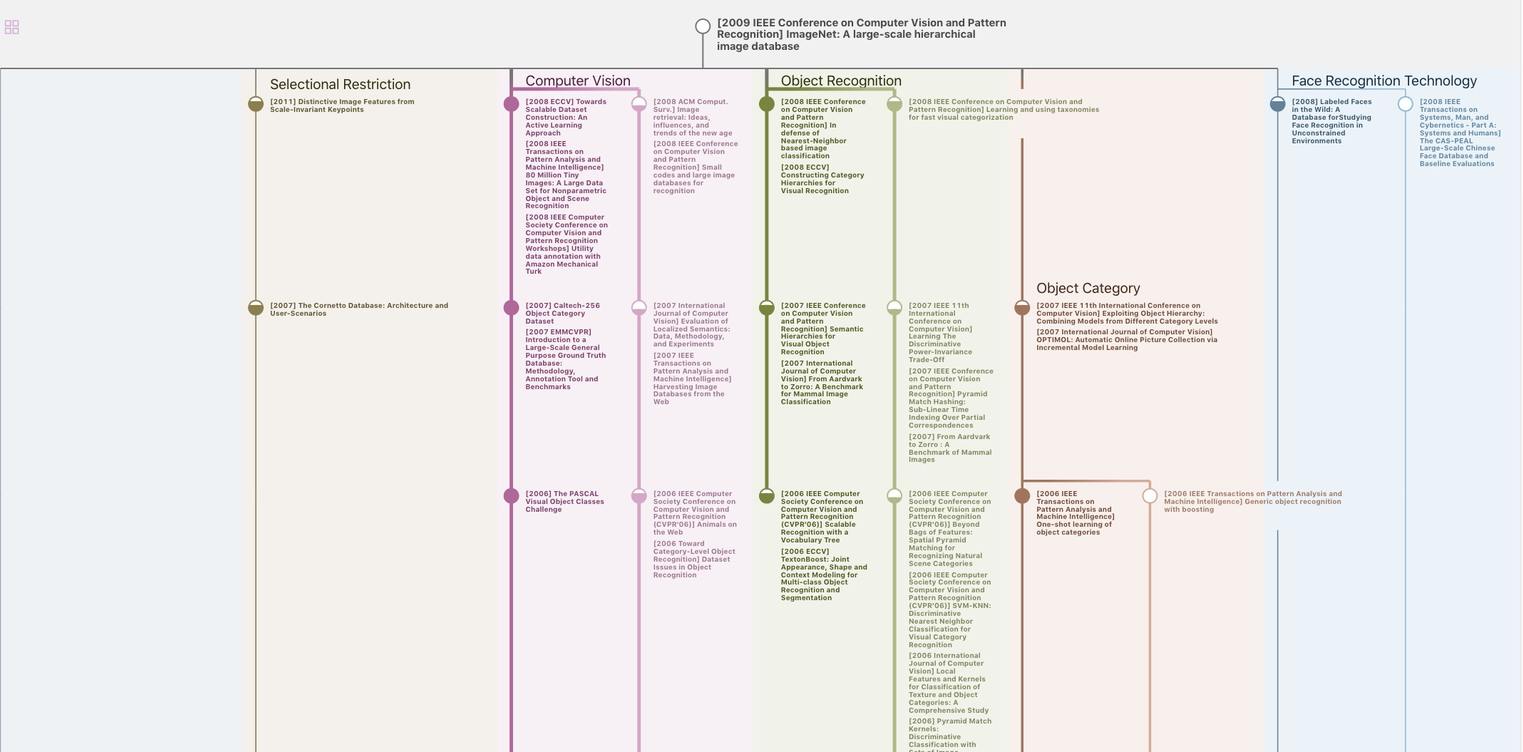
生成溯源树,研究论文发展脉络
Chat Paper
正在生成论文摘要