DINA Model with Entropy Penalization
Mathematics(2023)
摘要
The cognitive diagnosis model (CDM) is an effective statistical tool for extracting the discrete attributes of individuals based on their responses to diagnostic tests. When dealing with cases that involve small sample sizes or highly correlated attributes, not all attribute profiles may be present. The standard method, which accounts for all attribute profiles, not only increases the complexity of the model but also complicates the calculation. Thus, it is important to identify the empty attribute profiles. This paper proposes an entropy-penalized likelihood method to eliminate the empty attribute profiles. In addition, the relation between attribute profiles and the parameter space of item parameters is discussed, and two modified expectation–maximization (EM) algorithms are designed to estimate the model parameters. Simulations are conducted to demonstrate the performance of the proposed method, and a real data application based on the fraction–subtraction data is presented to showcase the practical implications of the proposed method.
更多查看译文
关键词
cognitive diagnosis model,DINA model,penalized likelihood,Shannon entropy,EM algorithm
AI 理解论文
溯源树
样例
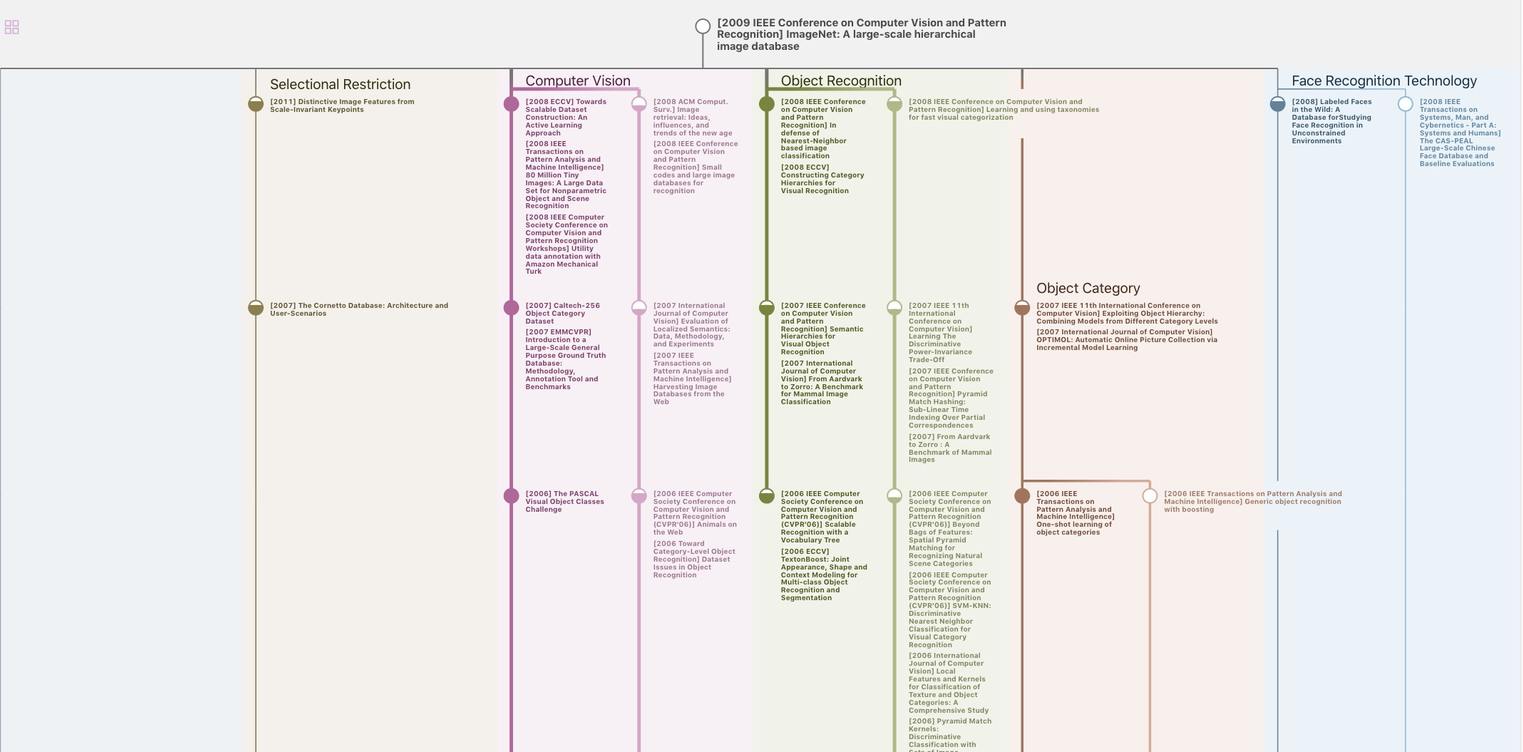
生成溯源树,研究论文发展脉络
Chat Paper
正在生成论文摘要