Exploiting Big Data for Experiment Reporting: the Hi-Drive Collaborative Research Project Case
Sensors(2023)
Univ Genoa | Univ Warwick | Rhein Westfal TH Aachen
Abstract
As timely information about a project’s state is key for management, we developed a data toolchain to support the monitoring of a project’s progress. By extending the Measurify framework, which is dedicated to efficiently building measurement-rich applications on MongoDB, we were able to make the process of setting up the reporting tool just a matter of editing a couple of .json configuration files that specify the names and data format of the project’s progress/performance indicators. Since the quantity of data to be provided at each reporting period is potentially overwhelming, some level of automation in the extraction of the indicator values is essential. To this end, it is important to make sure that most, if not all, of the quantities to be reported can be automatically extracted from the experiment data files actually used in the project. The originating use case for the toolchain is a collaborative research project on driving automation. As data representing the project’s state, 330+ numerical indicators were identified. According to the project’s pre-test experience, the tool is effective in supporting the preparation of periodic progress reports that extensively exploit the actual project data (i.e., obtained from the sensors—real or virtual—deployed for the project). While the presented use case concerns the automotive industry, we have taken care that the design choices (particularly, the definition of the resources exposed by the Application Programming Interfaces, APIs) abstract the requirements, with an aim to guarantee effectiveness in virtually any application context.
MoreTranslated text
Key words
big data architecture,project monitoring and reporting,non-relational DB,RESTful APIs,field operational tests,automated driving
求助PDF
上传PDF
View via Publisher
AI Read Science
AI Summary
AI Summary is the key point extracted automatically understanding the full text of the paper, including the background, methods, results, conclusions, icons and other key content, so that you can get the outline of the paper at a glance.
Example
Background
Key content
Introduction
Methods
Results
Related work
Fund
Key content
- Pretraining has recently greatly promoted the development of natural language processing (NLP)
- We show that M6 outperforms the baselines in multimodal downstream tasks, and the large M6 with 10 parameters can reach a better performance
- We propose a method called M6 that is able to process information of multiple modalities and perform both single-modal and cross-modal understanding and generation
- The model is scaled to large model with 10 billion parameters with sophisticated deployment, and the 10 -parameter M6-large is the largest pretrained model in Chinese
- Experimental results show that our proposed M6 outperforms the baseline in a number of downstream tasks concerning both single modality and multiple modalities We will continue the pretraining of extremely large models by increasing data to explore the limit of its performance
Upload PDF to Generate Summary
Must-Reading Tree
Example
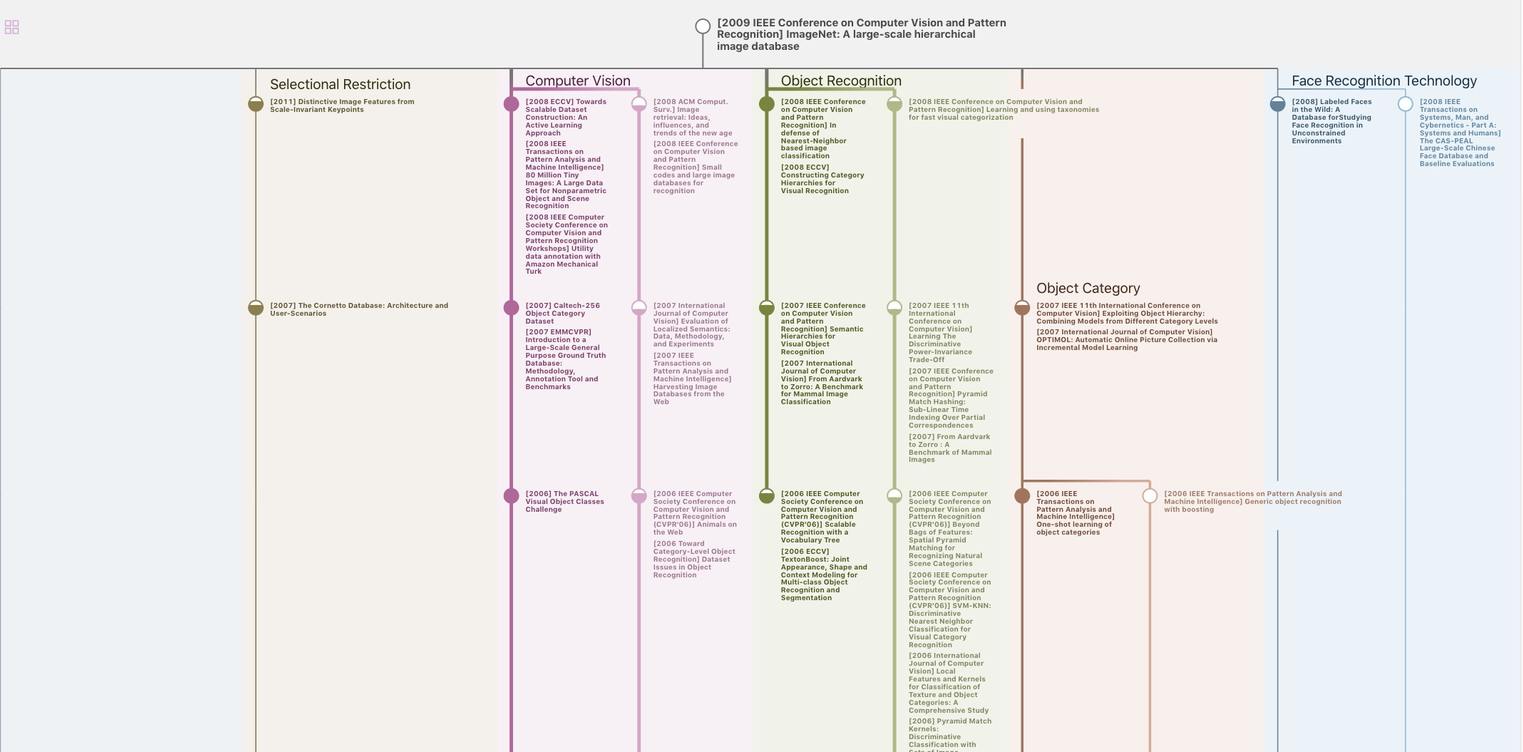
Generate MRT to find the research sequence of this paper
Related Papers
Data Disclaimer
The page data are from open Internet sources, cooperative publishers and automatic analysis results through AI technology. We do not make any commitments and guarantees for the validity, accuracy, correctness, reliability, completeness and timeliness of the page data. If you have any questions, please contact us by email: report@aminer.cn
Chat Paper