Performance Analysis of Six Semi-Automated Tumour Delineation Methods on [18F] Fluorodeoxyglucose Positron Emission Tomography/Computed Tomography (FDG PET/CT) in Patients with Head and Neck Cancer
Sensors(2023)
摘要
Background. Head and neck cancer (HNC) is the seventh most common neoplastic disorder at the global level. Contouring HNC lesions on [F-18] Fluorodeoxyglucose positron emission tomography/computed tomography (FDG PET/CT) scans plays a fundamental role for diagnosis, risk assessment, radiotherapy planning and post-treatment evaluation. However, manual contouring is a lengthy and tedious procedure which requires significant effort from the clinician. Methods. We evaluated the performance of six hand-crafted, training-free methods (four threshold-based, two algorithm-based) for the semi-automated delineation of HNC lesions on FDG PET/CT. This study was carried out on a single-centre population of n = 103 subjects, and the standard of reference was manual segmentation generated by nuclear medicine specialists. Figures of merit were the Sorensen-Dice coefficient (DSC) and relative volume difference (RVD). Results. Median DSC ranged between 0.595 and 0.792, median RVD between -22.0% and 87.4%. Click and draw and Nestle's methods achieved the best segmentation accuracy (median DSC, respectively, 0.792 +/- 0.178 and 0.762 +/- 0.107; median RVD, respectively, -21.6% +/- 1270.8% and -32.7% +/- 40.0%) and outperformed the other methods by a significant margin. Nestle's method also resulted in a lower dispersion of the data, hence showing stronger inter-patient stability. The accuracy of the two best methods was in agreement with the most recent state-of-the art results. Conclusions. Semi-automated PET delineation methods show potential to assist clinicians in the segmentation of HNC lesions on FDG PET/CT images, although manual refinement may sometimes be needed to obtain clinically acceptable ROIs.
更多查看译文
关键词
head and neck cancer,positron emission tomography,segmentation,region of interest,radiomics
AI 理解论文
溯源树
样例
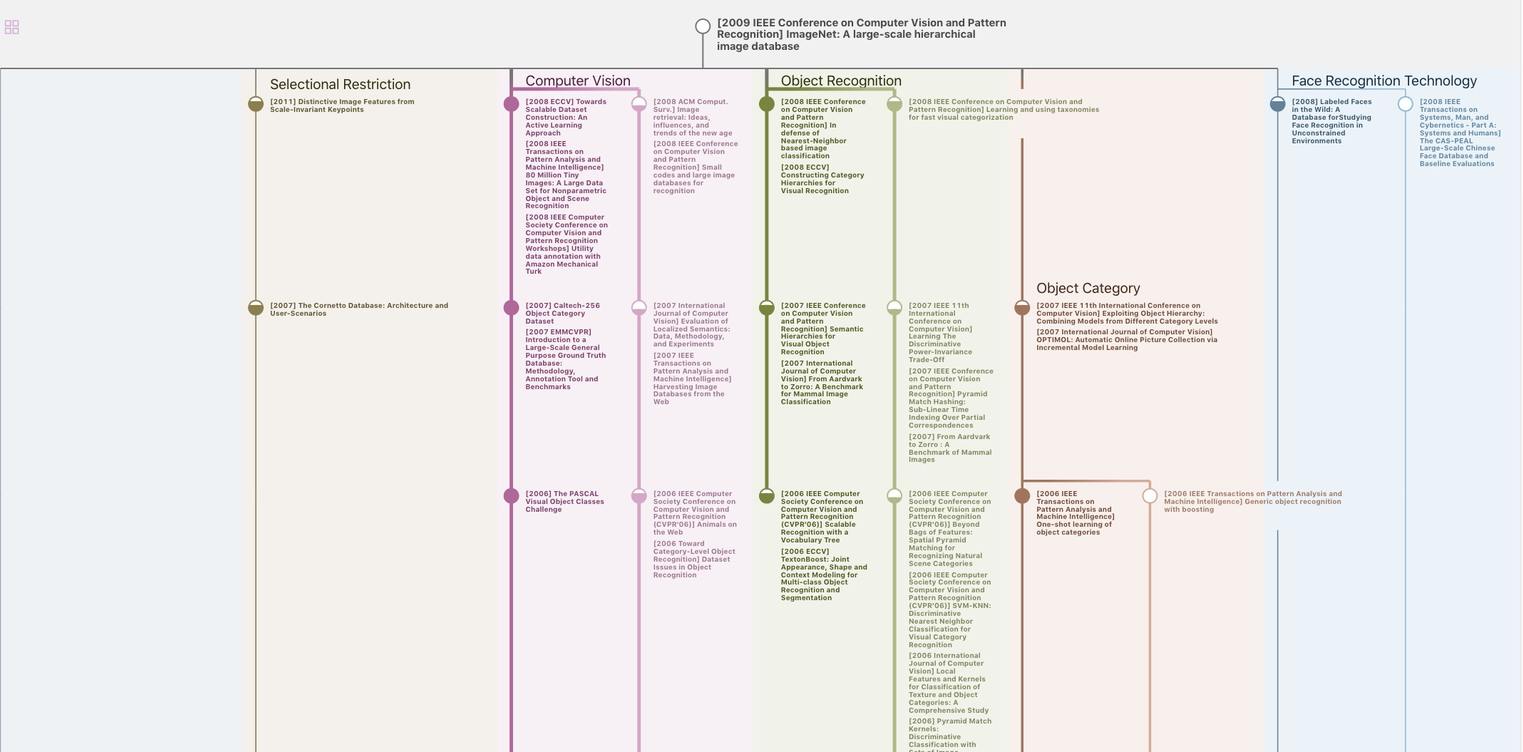
生成溯源树,研究论文发展脉络
Chat Paper
正在生成论文摘要