Development of Monthly Scale Precipitation-Forecasting Model for Indian Subcontinent Using Wavelet-Based Deep Learning Approach
doaj(2023)
摘要
In the present work, a wavelet-based multiscale deep learning approach is developed to forecast precipitation using the lagged monthly rainfall, local climate variables, and global teleconnections such as IOD, PDO, NAO, and Nino 3.4 as predictors. The conventional methods are limited by their inability to capture the high precipitation variability in time and space. The proposed multiscale method was tested and validated over the Krishna River basin in India. The results from the proposed methods were compared with contemporary models based on Multiple Linear Regression and Neural Networks. Overall, the forecasting accuracy was higher using the wavelet-based hybrid models than the single-scale models. The wavelet-based methods yielded results with 13–34% reduced error when compared with the best single-scale models. The proposed multi-scale model was then applied to the different climatic regions of the country, and it was shown that the model could forecast rainfall with reasonable accuracy for different climate zones of the country.
更多查看译文
关键词
monthly precipitation forecast,wavelet-based machine learning,teleconnections
AI 理解论文
溯源树
样例
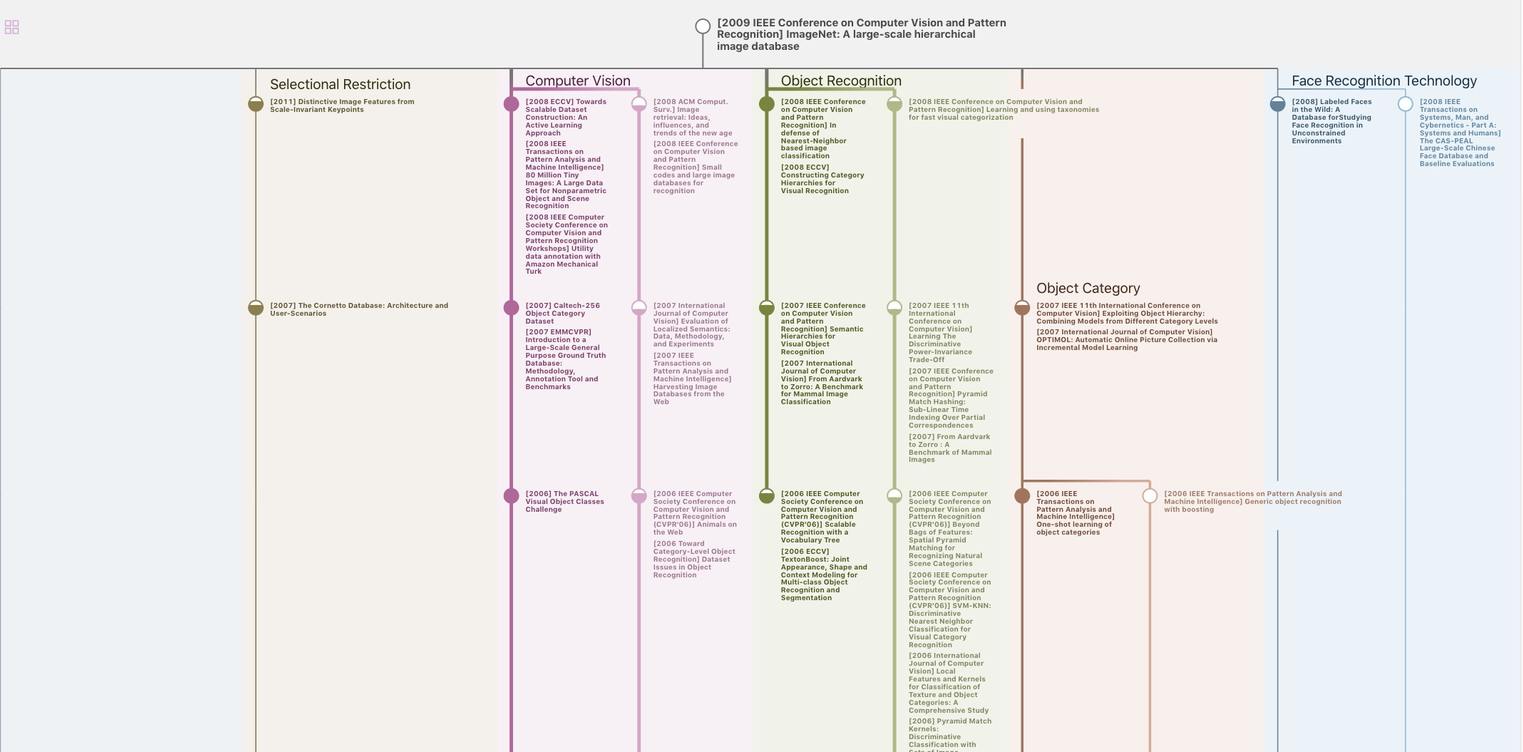
生成溯源树,研究论文发展脉络
Chat Paper
正在生成论文摘要