Recurrent Hypernetworks are Surprisingly Strong in Meta-RL
NeurIPS(2023)
摘要
Deep reinforcement learning (RL) is notoriously impractical to deploy due to sample inefficiency. Meta-RL directly addresses this sample inefficiency by learning to perform few-shot learning when a distribution of related tasks is available for meta-training. While many specialized meta-RL methods have been proposed, recent work suggests that end-to-end learning in conjunction with an off-the-shelf sequential model, such as a recurrent network, is a surprisingly strong baseline. However, such claims have been controversial due to limited supporting evidence, particularly in the face of prior work establishing precisely the opposite. In this paper, we conduct an empirical investigation. While we likewise find that a recurrent network can achieve strong performance, we demonstrate that the use of hypernetworks is crucial to maximizing their potential. Surprisingly, when combined with hypernetworks, the recurrent baselines that are far simpler than existing specialized methods actually achieve the strongest performance of all methods evaluated.
更多查看译文
关键词
recurrent hypernetworks
AI 理解论文
溯源树
样例
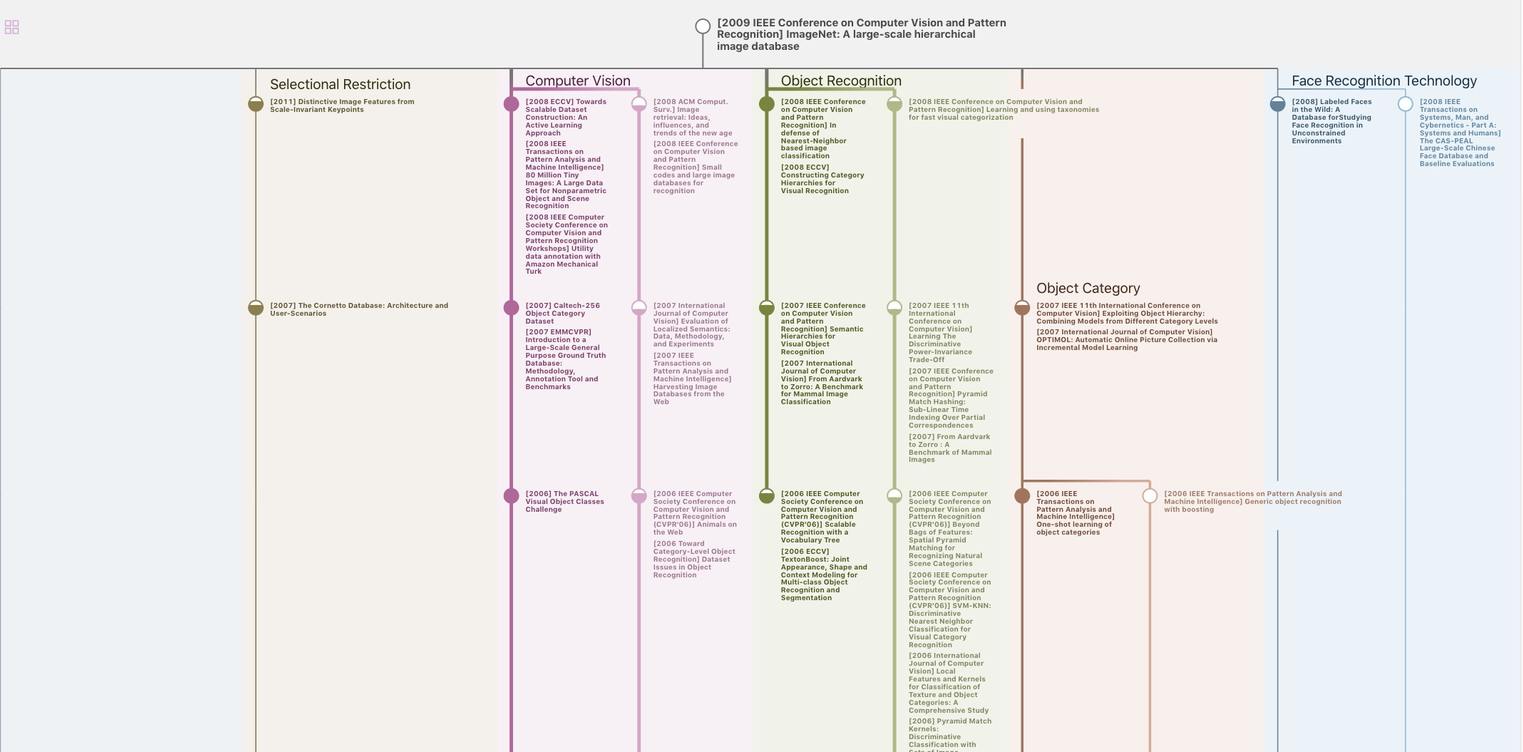
生成溯源树,研究论文发展脉络
Chat Paper
正在生成论文摘要