When Automated Assessment Meets Automated Content Generation: Examining Text Quality in the Era of GPTs
CoRR(2023)
摘要
The use of machine learning (ML) models to assess and score textual data has become increasingly pervasive in an array of contexts including natural language processing, information retrieval, search and recommendation, and credibility assessment of online content. A significant disruption at the intersection of ML and text are text-generating large-language models such as generative pre-trained transformers (GPTs). We empirically assess the differences in how ML-based scoring models trained on human content assess the quality of content generated by humans versus GPTs. To do so, we propose an analysis framework that encompasses essay scoring ML-models, human and ML-generated essays, and a statistical model that parsimoniously considers the impact of type of respondent, prompt genre, and the ML model used for assessment model. A rich testbed is utilized that encompasses 18,460 human-generated and GPT-based essays. Results of our benchmark analysis reveal that transformer pretrained language models (PLMs) more accurately score human essay quality as compared to CNN/RNN and feature-based ML methods. Interestingly, we find that the transformer PLMs tend to score GPT-generated text 10-15\% higher on average, relative to human-authored documents. Conversely, traditional deep learning and feature-based ML models score human text considerably higher. Further analysis reveals that although the transformer PLMs are exclusively fine-tuned on human text, they more prominently attend to certain tokens appearing only in GPT-generated text, possibly due to familiarity/overlap in pre-training. Our framework and results have implications for text classification settings where automated scoring of text is likely to be disrupted by generative AI.
更多查看译文
关键词
automated content generation,text quality,automated assessment
AI 理解论文
溯源树
样例
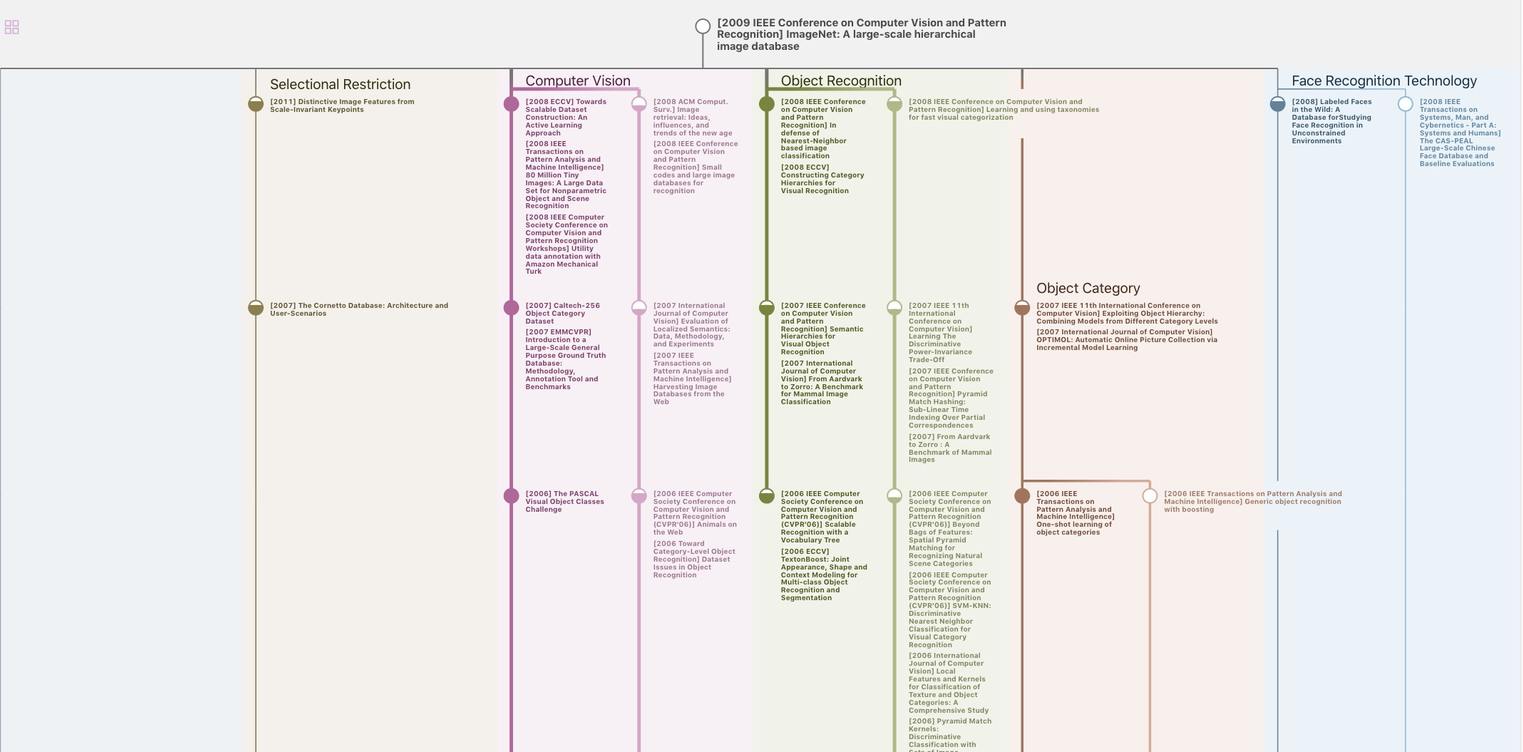
生成溯源树,研究论文发展脉络
Chat Paper
正在生成论文摘要