ReConcile: Round-Table Conference Improves Reasoning via Consensus among Diverse LLMs
CoRR(2023)
摘要
Large Language Models (LLMs) still struggle with natural language reasoning
tasks. Motivated by the society of minds (Minsky, 1988), we propose ReConcile,
a multi-model multiagent framework designed as a round table conference among
diverse LLM agents. ReConcile enhances collaborative reasoning between LLM
agents via multiple rounds of discussion, learning to convince other agents to
improve their answers, and employing a confidence-weighted voting mechanism
that leads to a better consensus. In each round, ReConcile initiates discussion
between agents via a 'discussion prompt' that consists of (a) grouped answers
and explanations generated by each agent in the previous round, (b) their
confidence scores, and (c) demonstrations of answer-rectifying human
explanations, used for convincing other agents. Experiments on seven benchmarks
demonstrate that ReConcile significantly improves LLMs' reasoning – both
individually and as a team – surpassing prior single-agent and multi-agent
baselines by up to 11.4
ReConcile also flexibly incorporates different combinations of agents,
including API-based, open-source, and domain-specific models, leading to an 8
improvement on MATH. Finally, we analyze the individual components of
ReConcile, demonstrating that the diversity originating from different models
is critical to its superior performance. Code:
https://github.com/dinobby/ReConcile
更多查看译文
关键词
diverse llms,conference,consensus,reasoning,round-table
AI 理解论文
溯源树
样例
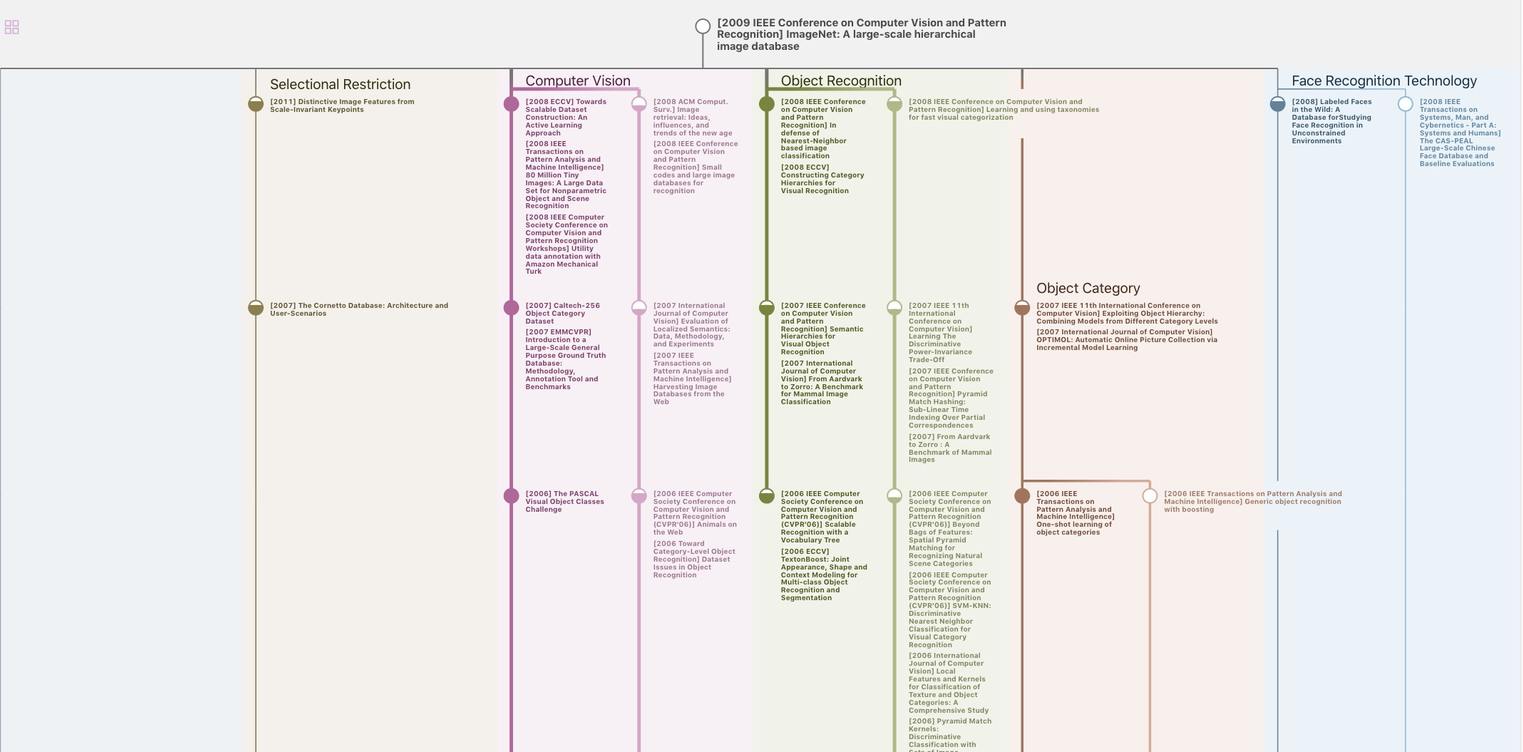
生成溯源树,研究论文发展脉络
Chat Paper
正在生成论文摘要