RadarFormer: End-to-End Human Perception With Through-Wall Radar and Transformers
IEEE transactions on neural networks and learning systems(2023)
摘要
For fine-grained human perception tasks such as pose estimation and activity recognition, radar-based sensors show advantages over optical cameras in low-visibility, privacy-aware, and wall-occlusive environments. Radar transmits radio frequency signals to irradiate the target of interest and store the target information in the echo signals. One common approach is to transform the echoes into radar images and extract the features with convolutional neural networks. This article introduces RadarFormer, the first method that introduces the self-attention (SA) mechanism to perform human perception tasks directly from radar echoes. It bypasses the imaging algorithm and realizes end-to-end signal processing. Specifically, we give constructive proof that processing radar echoes using the SA mechanism is at least as expressive as processing radar images using the convolutional layer. On this foundation, we design RadarFormer, which is a Transformer-like model to process radar signals. It benefits from the fast-/slow-time SA mechanism considering the physical characteristics of radar signals. RadarFormer extracts human representations from radar echoes and handles various downstream human perception tasks. The experimental results demonstrate that our method outperforms the state-of-the-art radar-based methods both in performance and computational cost and obtains accurate human perception results even in dark and occlusive environments.
更多查看译文
关键词
End-to-end signal processing,fine-grained human perception,radio frequency (RF) signal,self-attention (SA) mechanism
AI 理解论文
溯源树
样例
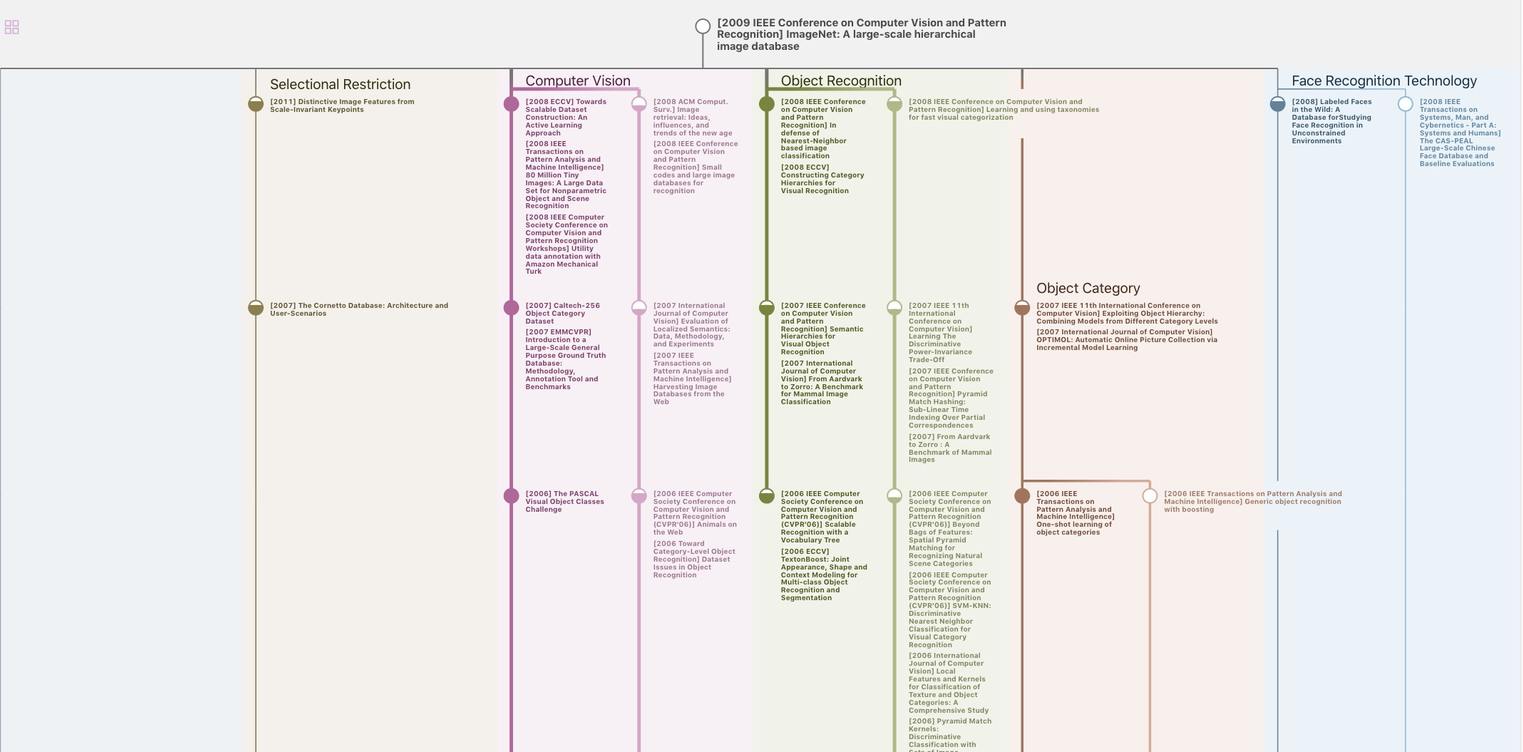
生成溯源树,研究论文发展脉络
Chat Paper
正在生成论文摘要