Tiny Machine Learning for Real-time Postural Stability Analysis
2023 IEEE Sensors Applications Symposium (SAS)(2023)
摘要
Postural sway is a critical measure for evaluating postural control, and its analysis plays a vital role in preventing falls among the elderly. Typically, physiotherapists assess an individual's postural control using tests such as the Berg Balance Scale, Tinetti Test, and time up-and-go test. Sensor-based analysis is available based on devices such as force plates or inertial measurement units. Recently, machine learning methods have demonstrated promising results in the sensor-based analysis of postural control. However, these models are often complex, slow, and energy-intensive. To address these limitations, this study explores the design space of lightweight machine learning models deployable to microcontrollers to assess postural stability. We developed an artificial neural network (ANN) model and compare its performance to that of random forests, gaussian naive bayes, and extra tree classifiers. The models are trained using a sway dataset with varying input sizes and signal-to-noise ratios. The dataset comprises two feature vectors extracted from raw accelerometer data. The developed models are deployed to an ARM Cortex M4-based microcontroller, and their performance is evaluated and compared. We show that the ANN model has 99.03% accuracy, higher noise immunity, and the model performs better with a window size of one second with 590.96 us inference time.
更多查看译文
关键词
TinyML,postural sway,fall prevention,real-time postural assessment,embedded systems,machine learning
AI 理解论文
溯源树
样例
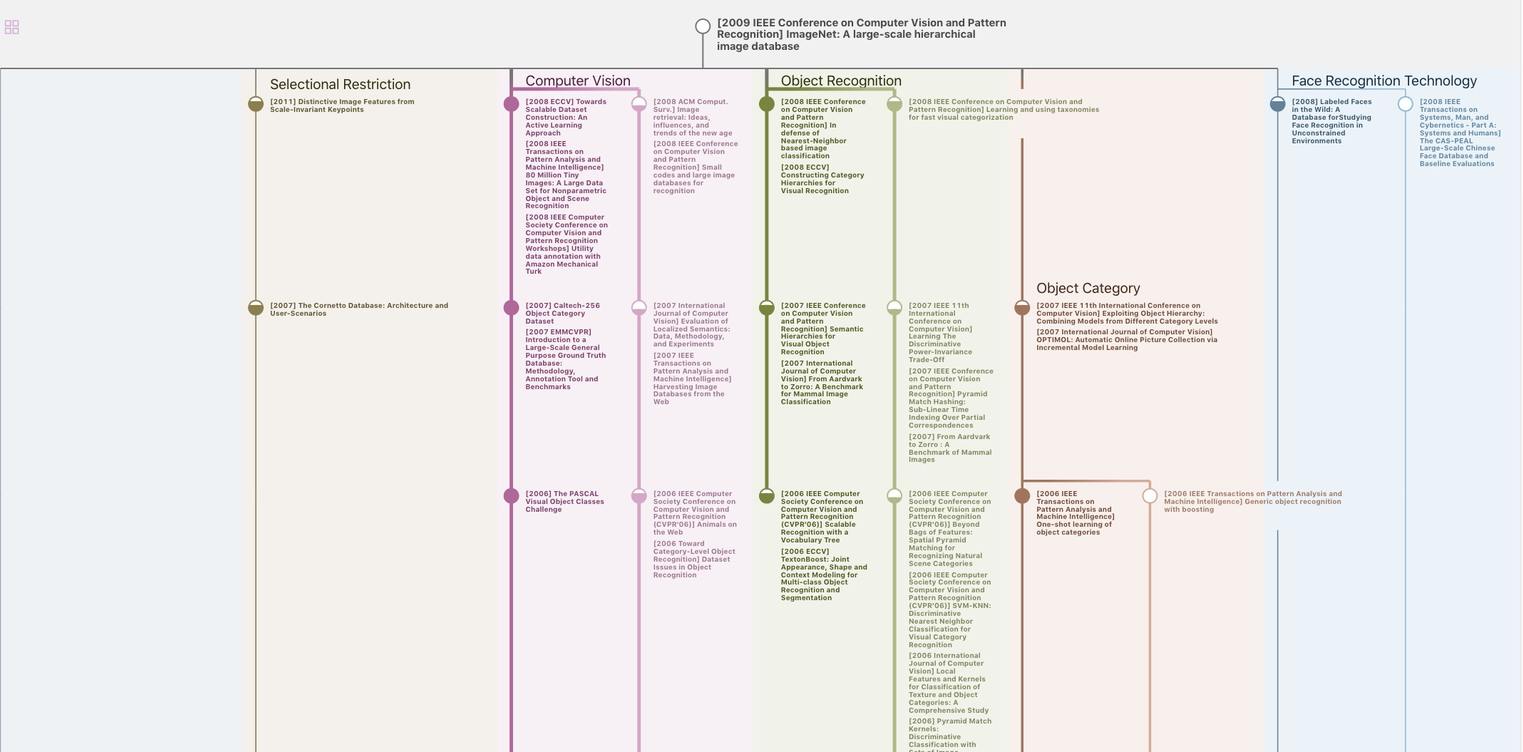
生成溯源树,研究论文发展脉络
Chat Paper
正在生成论文摘要