Real-Time Object Detection Performance of YOLOv8 Models for Self-Driving Cars in a Mixed Traffic Environment
2023 2nd International Conference on Computer System, Information Technology, and Electrical Engineering (COSITE)(2023)
Key words
Real-Time,Object Detection,YOLOv8,Self-Driving Car,Mixed-Traffic Environments
AI Read Science
Must-Reading Tree
Example
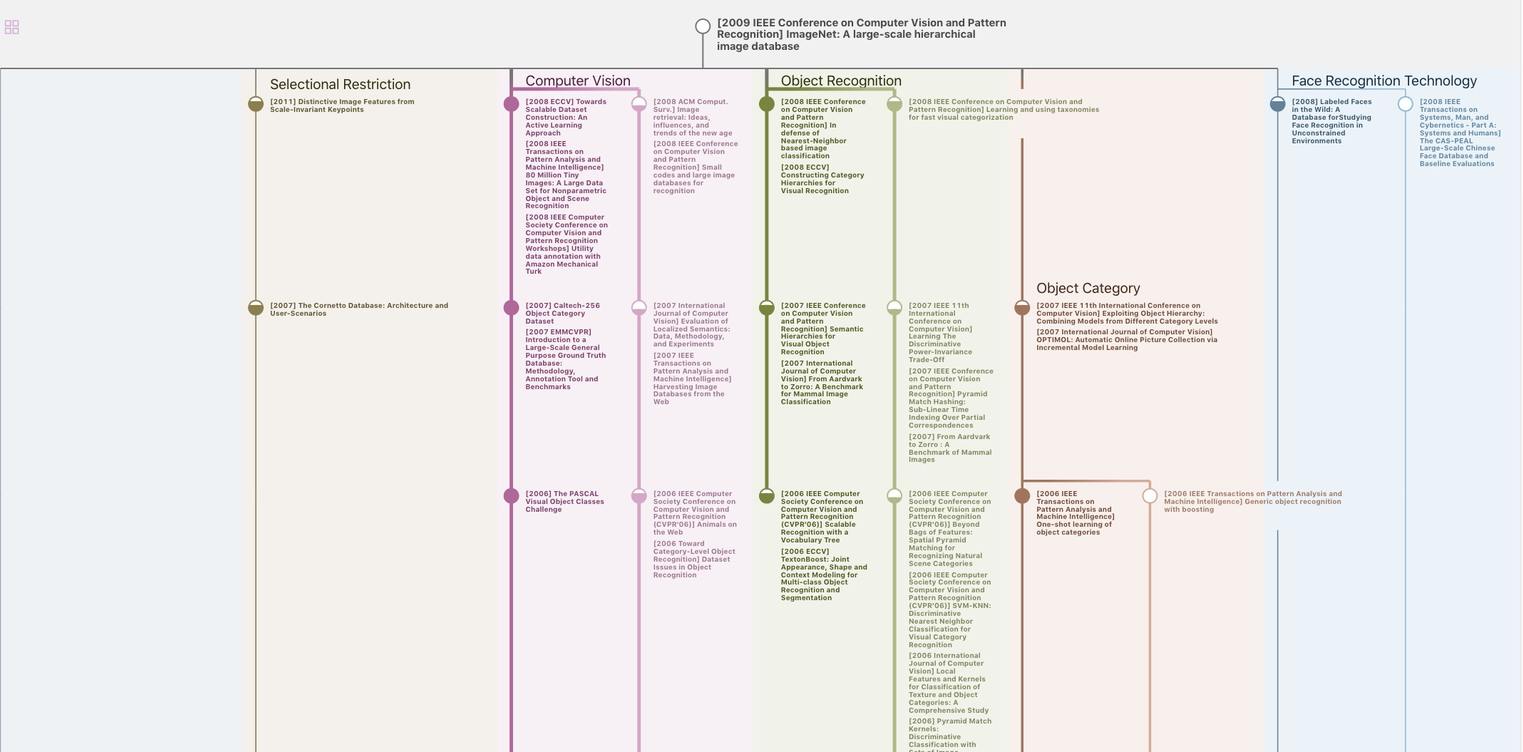
Generate MRT to find the research sequence of this paper
Chat Paper
Summary is being generated by the instructions you defined