Service Matching Based on Group Preference and Service Representation Learning for Edge Caching
2023 IEEE International Conference on Web Services (ICWS)(2023)
摘要
With the widespread application of edge computing, service providers cache resources on edge servers to meet users’ low latency requirements. Due to resource constraints, how to match candidate service sets deployed on edge servers has become a research hotspot. Most traditional service matching methods ignore group preferences in edge scenarios, which leads to poor service matching performance. Besides, these methods use simple embedding vectors or service usage frequencies to represent service, which lack deep mining of service representation. To improve the performance of service matching, this paper proposes a Service Matching method based on Group Preference and Service Representation Learning (SM-GPSRL). Group preference representation is obtained through deep embedding clustering and recurrent neural network. Graph attention network is adopted to compute the interaction between services for learning representation of services. And service matching is implemented based on the obtained representation. Experimental results on real-world datasets demonstrate that SM-GPSRL has a better performance compared with baseline methods.
更多查看译文
关键词
Edge Computing,Service Matching,Group Preference,Service Representation Learning
AI 理解论文
溯源树
样例
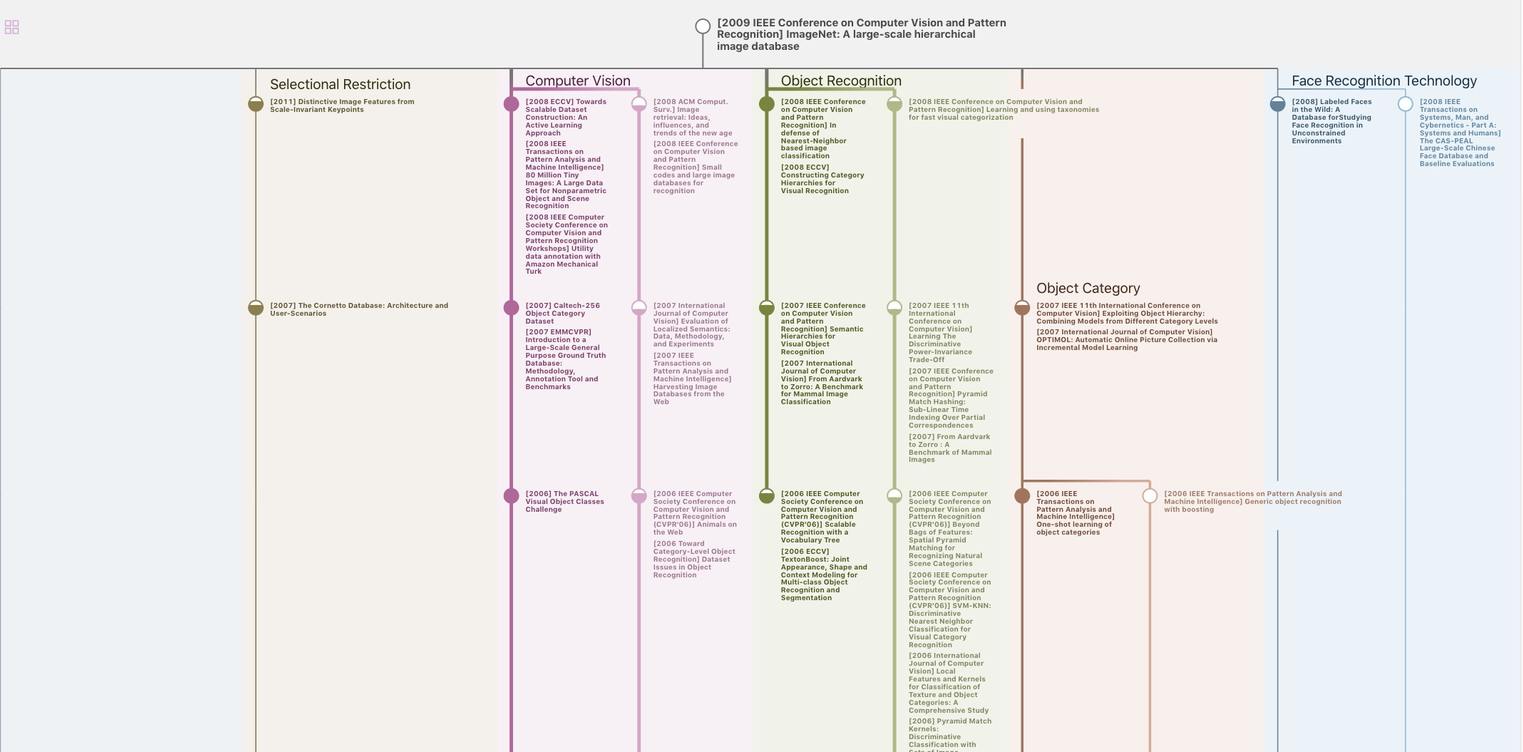
生成溯源树,研究论文发展脉络
Chat Paper
正在生成论文摘要