SPOT: Scalable 3D Pre-training via Occupancy Prediction for Learning Transferable 3D Representations
arxiv(2023)
摘要
Annotating 3D LiDAR point clouds for perception tasks is fundamental for many
applications e.g., autonomous driving, yet it still remains notoriously
labor-intensive. Pretraining-finetuning approach can alleviate the labeling
burden by fine-tuning a pre-trained backbone across various downstream datasets
as well as tasks. In this paper, we propose SPOT, namely Scalable Pre-training
via Occupancy prediction for learning Transferable 3D representations under
such a label-efficient fine-tuning paradigm. SPOT achieves effectiveness on
various public datasets with different downstream tasks, showcasing its general
representation power, cross-domain robustness and data scalability which are
three key factors for real-world application. Specifically, we both
theoretically and empirically show, for the first time, that general
representations learning can be achieved through the task of occupancy
prediction. Then, to address the domain gap caused by different LiDAR sensors
and annotation methods, we develop a beam re-sampling technique for point cloud
augmentation combined with class-balancing strategy. Furthermore, scalable
pre-training is observed, that is, the downstream performance across all the
experiments gets better with more pre-training data. Additionally, such
pre-training strategy also remains compatible with unlabeled data. The hope is
that our findings will facilitate the understanding of LiDAR points and pave
the way for future advancements in LiDAR pre-training.
更多查看译文
AI 理解论文
溯源树
样例
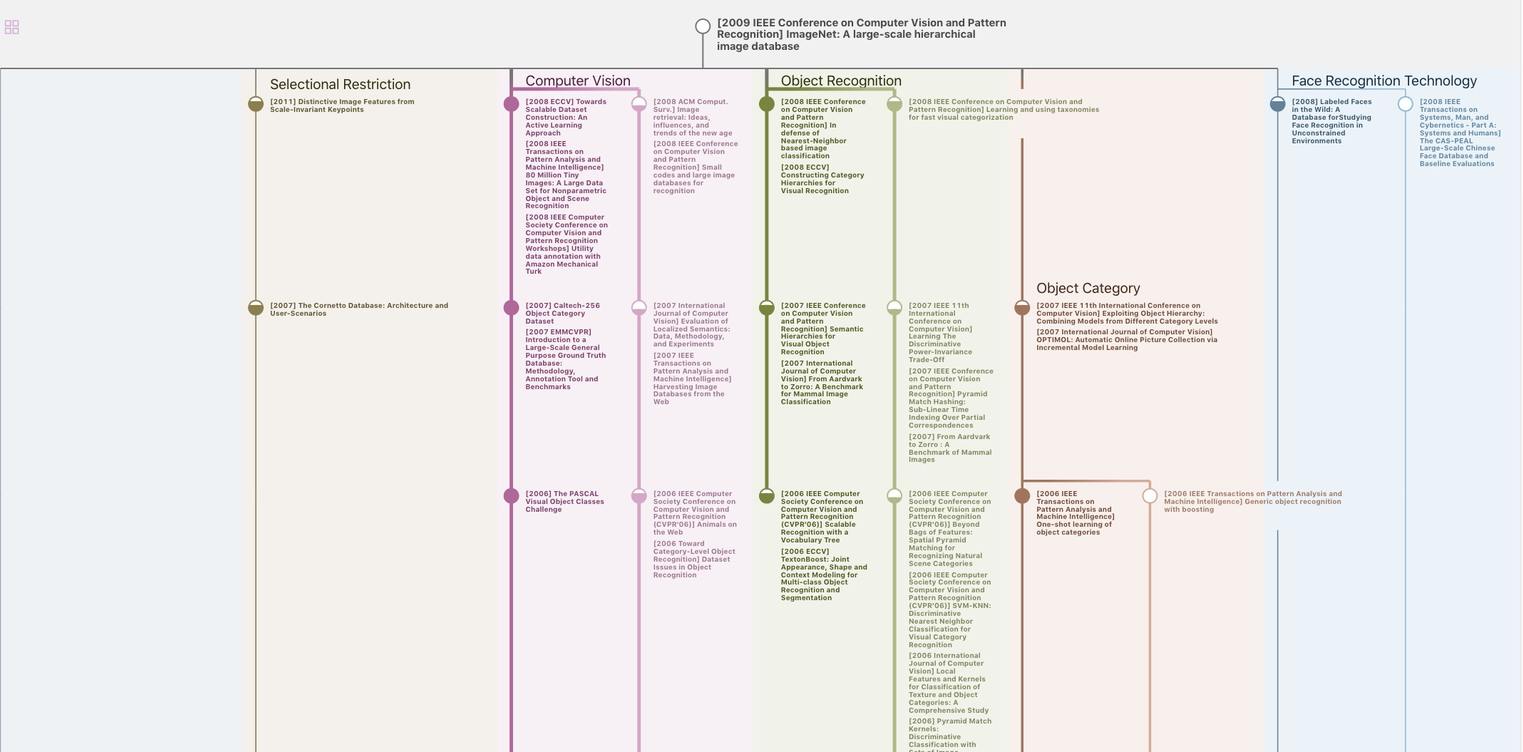
生成溯源树,研究论文发展脉络
Chat Paper
正在生成论文摘要