Unsupervised Green Object Tracker (GOT) without Offline Pre-training
CoRR(2023)
摘要
Supervised trackers trained on labeled data dominate the single object tracking field for superior tracking accuracy. The labeling cost and the huge computational complexity hinder their applications on edge devices. Unsupervised learning methods have also been investigated to reduce the labeling cost but their complexity remains high. Aiming at lightweight high-performance tracking, feasibility without offline pre-training, and algorithmic transparency, we propose a new single object tracking method, called the green object tracker (GOT), in this work. GOT conducts an ensemble of three prediction branches for robust box tracking: 1) a global object-based correlator to predict the object location roughly, 2) a local patch-based correlator to build temporal correlations of small spatial units, and 3) a superpixel-based segmentator to exploit the spatial information of the target frame. GOT offers competitive tracking accuracy with state-of-the-art unsupervised trackers, which demand heavy offline pre-training, at a lower computation cost. GOT has a tiny model size (<3k parameters) and low inference complexity (around 58M FLOPs per frame). Since its inference complexity is between 0.1%-10% of DL trackers, it can be easily deployed on mobile and edge devices.
更多查看译文
关键词
green,pre-training
AI 理解论文
溯源树
样例
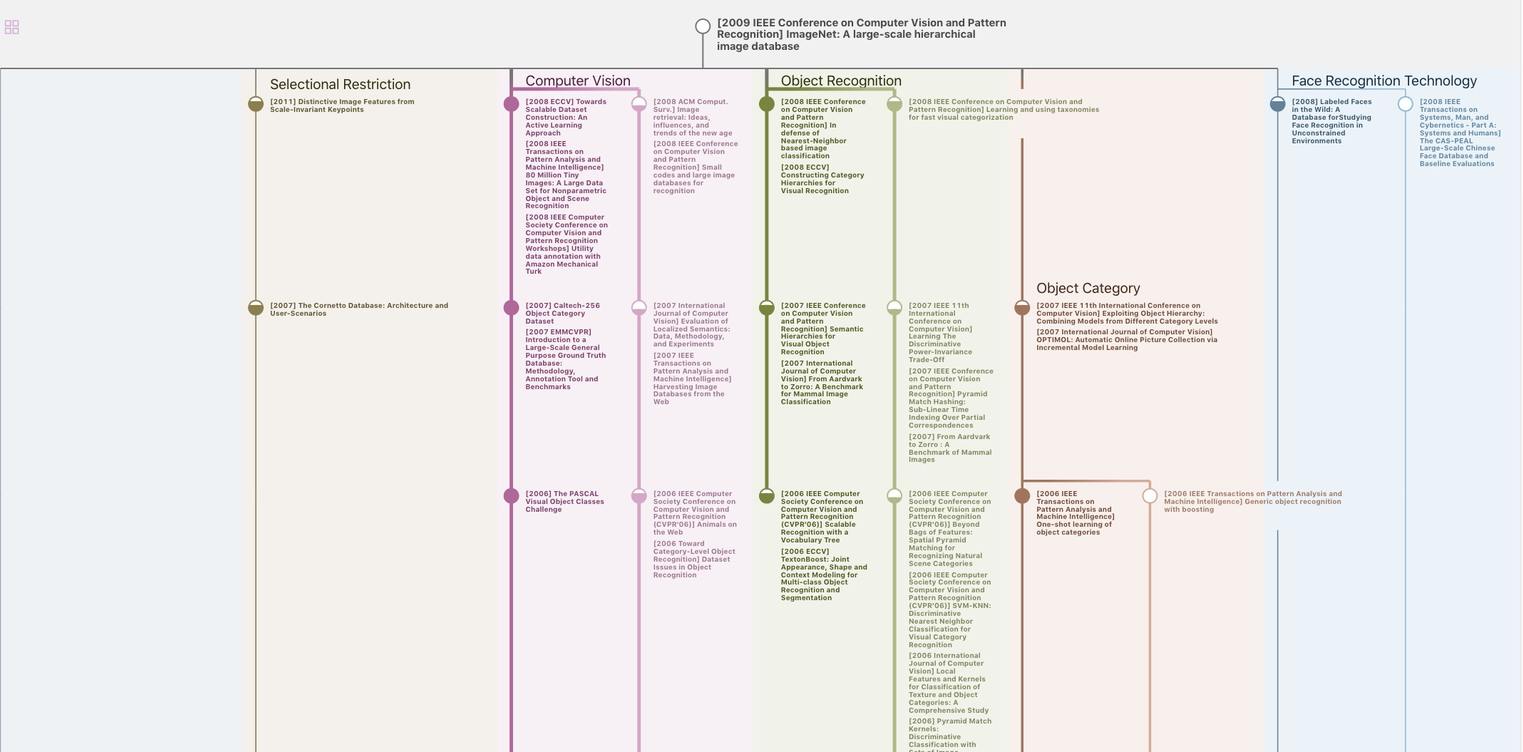
生成溯源树,研究论文发展脉络
Chat Paper
正在生成论文摘要