Fake News Detectors are Biased against Texts Generated by Large Language Models
CoRR(2023)
摘要
The spread of fake news has emerged as a critical challenge, undermining trust and posing threats to society. In the era of Large Language Models (LLMs), the capability to generate believable fake content has intensified these concerns. In this study, we present a novel paradigm to evaluate fake news detectors in scenarios involving both human-written and LLM-generated misinformation. Intriguingly, our findings reveal a significant bias in many existing detectors: they are more prone to flagging LLM-generated content as fake news while often misclassifying human-written fake news as genuine. This unexpected bias appears to arise from distinct linguistic patterns inherent to LLM outputs. To address this, we introduce a mitigation strategy that leverages adversarial training with LLM-paraphrased genuine news. The resulting model yielded marked improvements in detection accuracy for both human and LLM-generated news. To further catalyze research in this domain, we release two comprehensive datasets, \texttt{GossipCop++} and \texttt{PolitiFact++}, thus amalgamating human-validated articles with LLM-generated fake and real news.
更多查看译文
关键词
fake news detectors,large language
AI 理解论文
溯源树
样例
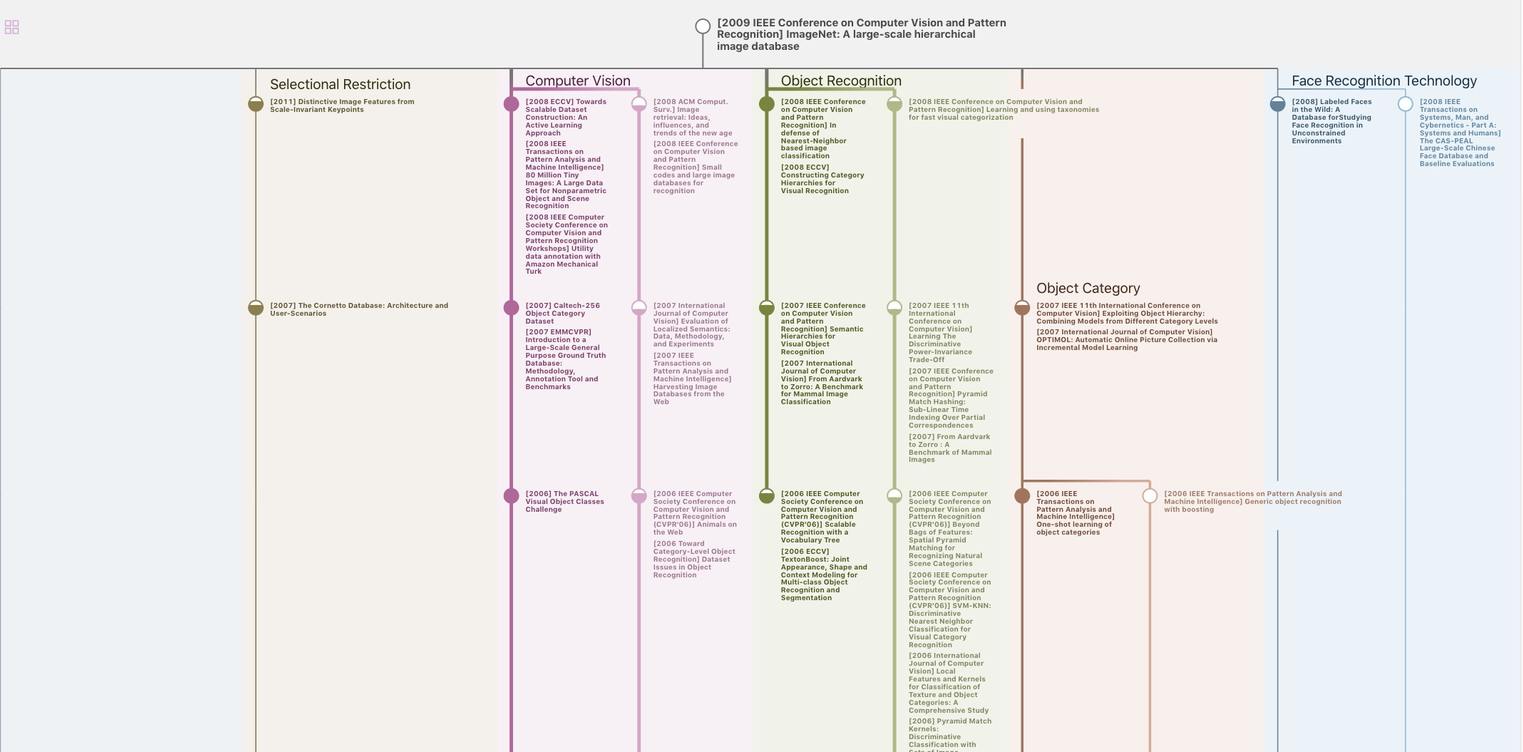
生成溯源树,研究论文发展脉络
Chat Paper
正在生成论文摘要