Automatic vocalisation detection delivers reliable, multi-faceted, and global avian biodiversity monitoring
biorxiv(2023)
摘要
Tracking biodiversity and its dynamics at scale is essential if we are to solve global environmental challenges. Detecting animal vocalisations in passively recorded audio data offers a highly automatable, inexpensive, and taxonomically broad way to monitor biodiversity. However, uptake is slow due to the expertise and labour required to label new data and fine-tune algorithms for each deployment. In this study, we applied an off-the-shelf bird vocalisation detection model, BirdNET, to 152,376 hours of audio comprising of datasets from Norway, Taiwan, Costa Rica, and Brazil. We manually listened to a subset of detections for each species in each dataset and found precisions of over 80% for 89 of the 139 species (100% for 57 species). Whilst some species were reliably detected across multiple datasets, the performance of others was dataset specific. By filtering out unreliable detections, we could extract species and community level insight on diel (Brazil) and seasonal (Taiwan) temporal scales, as well as landscape (Costa Rica) and national (Norway) spatial scales. Our findings demonstrate that, with a relatively fast validation step, a single vocalisation detection model can deliver multi-faceted community and species level insight across highly diverse datasets; unlocking the scale at which acoustic monitoring can deliver immediate applied impact.
### Competing Interest Statement
The authors have declared no competing interest.
更多查看译文
AI 理解论文
溯源树
样例
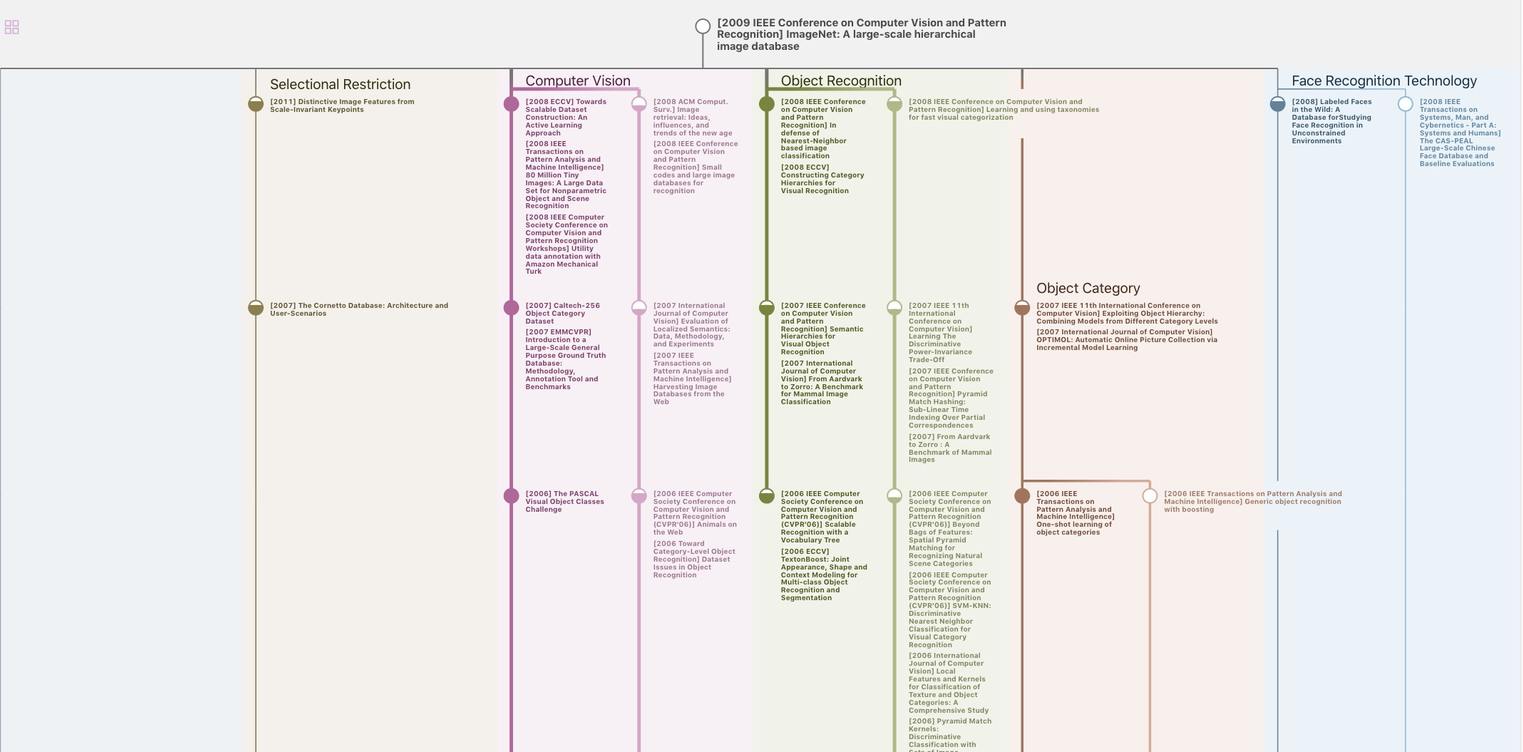
生成溯源树,研究论文发展脉络
Chat Paper
正在生成论文摘要