Is Solving Graph Neural Tangent Kernel Equivalent to Training Graph Neural Network?
CoRR(2023)
摘要
A rising trend in theoretical deep learning is to understand why deep learning works through Neural Tangent Kernel (NTK) [jgh18], a kernel method that is equivalent to using gradient descent to train a multi-layer infinitely-wide neural network. NTK is a major step forward in the theoretical deep learning because it allows researchers to use traditional mathematical tools to analyze properties of deep neural networks and to explain various neural network techniques from a theoretical view. A natural extension of NTK on graph learning is \textit{Graph Neural Tangent Kernel (GNTK)}, and researchers have already provide GNTK formulation for graph-level regression and show empirically that this kernel method can achieve similar accuracy as GNNs on various bioinformatics datasets [dhs+19]. The remaining question now is whether solving GNTK regression is equivalent to training an infinite-wide multi-layer GNN using gradient descent. In this paper, we provide three new theoretical results. First, we formally prove this equivalence for graph-level regression. Second, we present the first GNTK formulation for node-level regression. Finally, we prove the equivalence for node-level regression.
更多查看译文
关键词
training graph,neural network
AI 理解论文
溯源树
样例
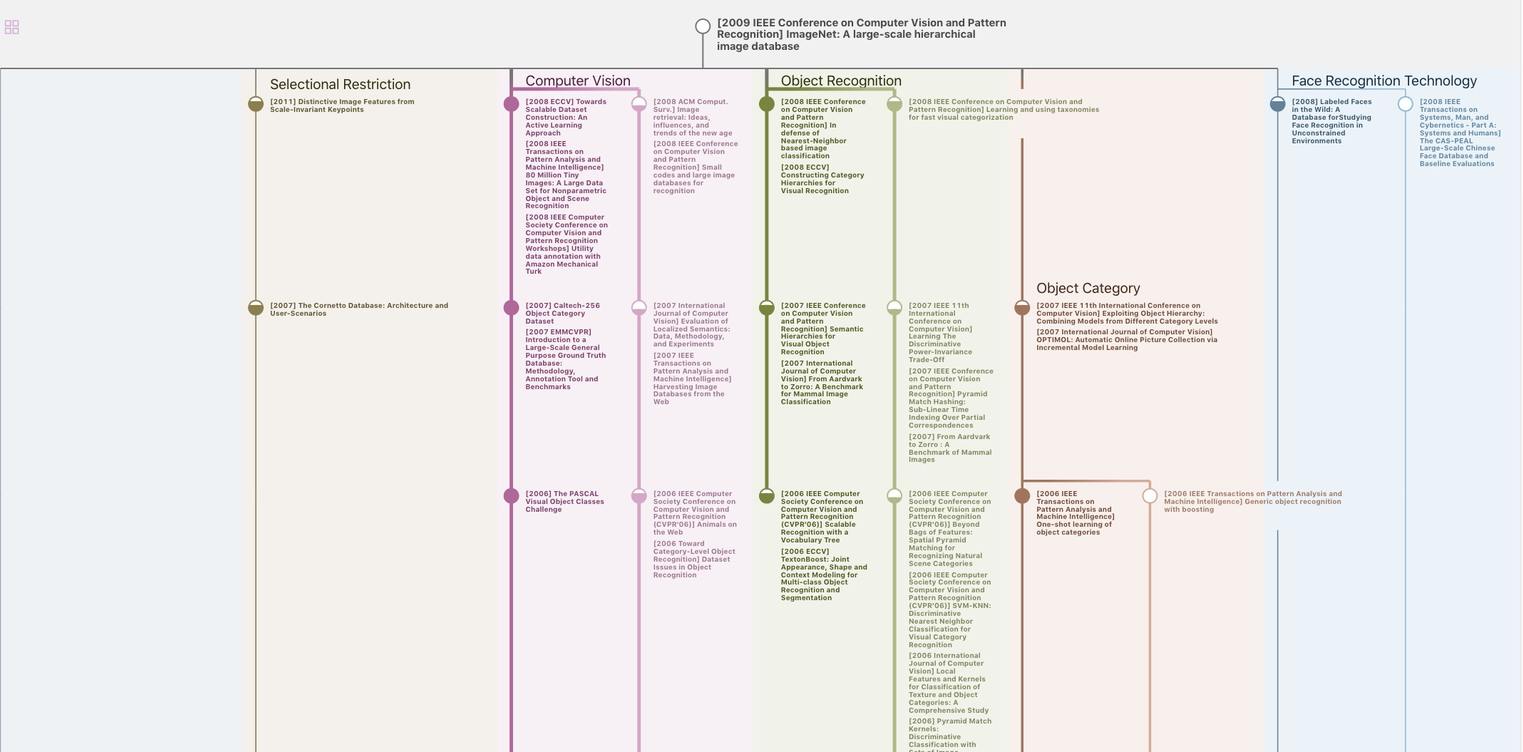
生成溯源树,研究论文发展脉络
Chat Paper
正在生成论文摘要