Measuring Catastrophic Forgetting in Cross-Lingual Transfer Paradigms: Exploring Tuning Strategies
arxiv(2023)
摘要
The cross-lingual transfer is a promising technique to solve tasks in
less-resourced languages. In this empirical study, we compare two fine-tuning
approaches combined with zero-shot and full-shot learning approaches for large
language models in a cross-lingual setting. As fine-tuning strategies, we
compare parameter-efficient adapter methods with fine-tuning of all parameters.
As cross-lingual transfer strategies, we compare the intermediate-training
(IT) that uses each language sequentially and cross-lingual validation
(CLV) that uses a target language already in the validation phase of
fine-tuning. We assess the success of transfer and the extent of catastrophic
forgetting in a source language due to cross-lingual transfer, i.e., how much
previously acquired knowledge is lost when we learn new information in a
different language. The results on two different classification problems, hate
speech detection and product reviews, each containing datasets in several
languages, show that the IT cross-lingual strategy outperforms
CLV for the target language. Our findings indicate that, in the
majority of cases, the CLV strategy demonstrates superior retention of
knowledge in the base language (English) compared to the IT strategy,
when evaluating catastrophic forgetting in multiple cross-lingual transfers.
更多查看译文
AI 理解论文
溯源树
样例
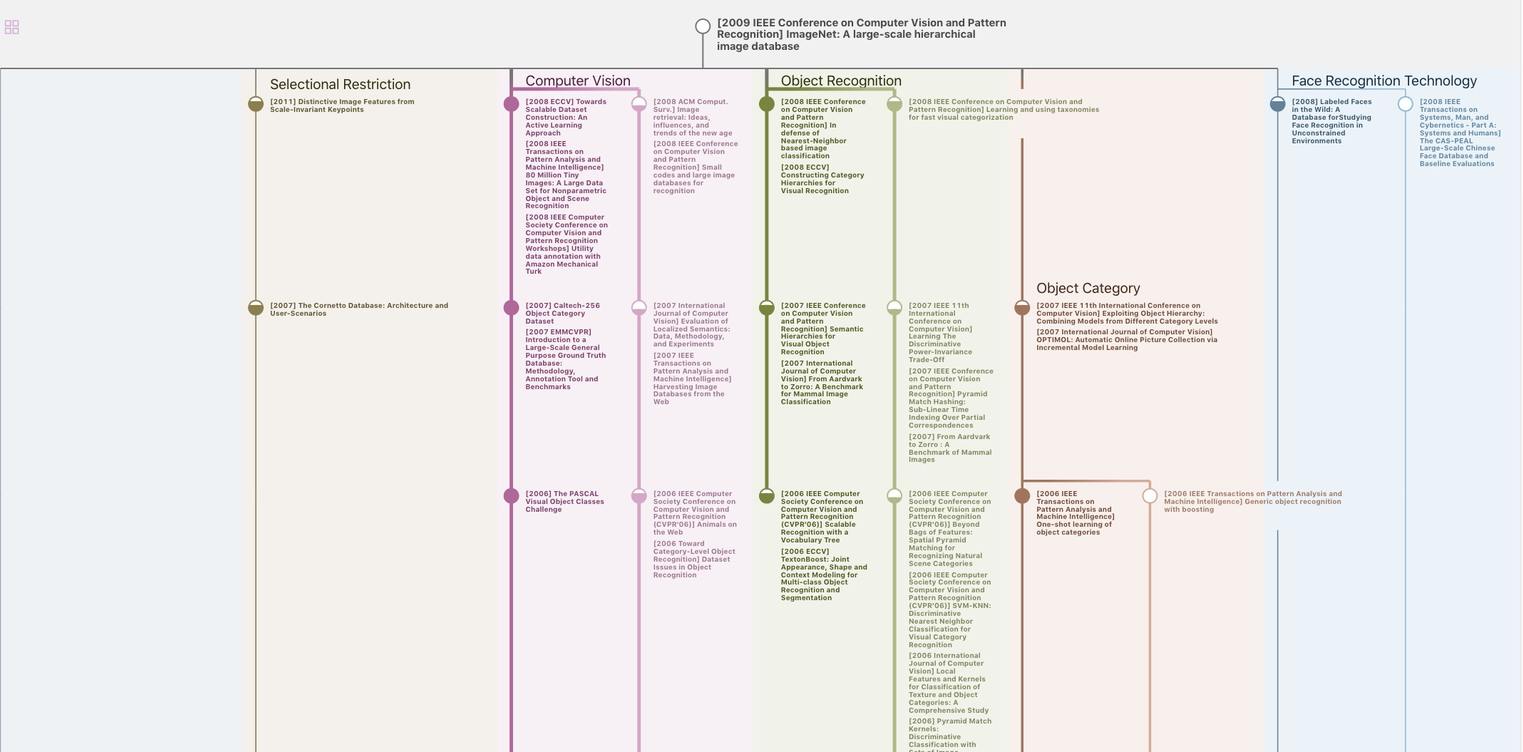
生成溯源树,研究论文发展脉络
Chat Paper
正在生成论文摘要