End-to-End Speech Recognition and Disfluency Removal with Acoustic Language Model Pretraining
CoRR(2023)
摘要
The SOTA in transcription of disfluent and conversational speech has in recent years favored two-stage models, with separate transcription and cleaning stages. We believe that previous attempts at end-to-end disfluency removal have fallen short because of the representational advantage that large-scale language model pretraining has given to lexical models. Until recently, the high dimensionality and limited availability of large audio datasets inhibited the development of large-scale self-supervised pretraining objectives for learning effective audio representations, giving a relative advantage to the two-stage approach, which utilises pretrained representations for lexical tokens. In light of recent successes in large scale audio pretraining, we revisit the performance comparison between two-stage and end-to-end model and find that audio based language models pretrained using weak self-supervised objectives match or exceed the performance of similarly trained two-stage models, and further, that the choice of pretraining objective substantially effects a model's ability to be adapted to the disfluency removal task.
更多查看译文
关键词
disfluency removal,acoustic
AI 理解论文
溯源树
样例
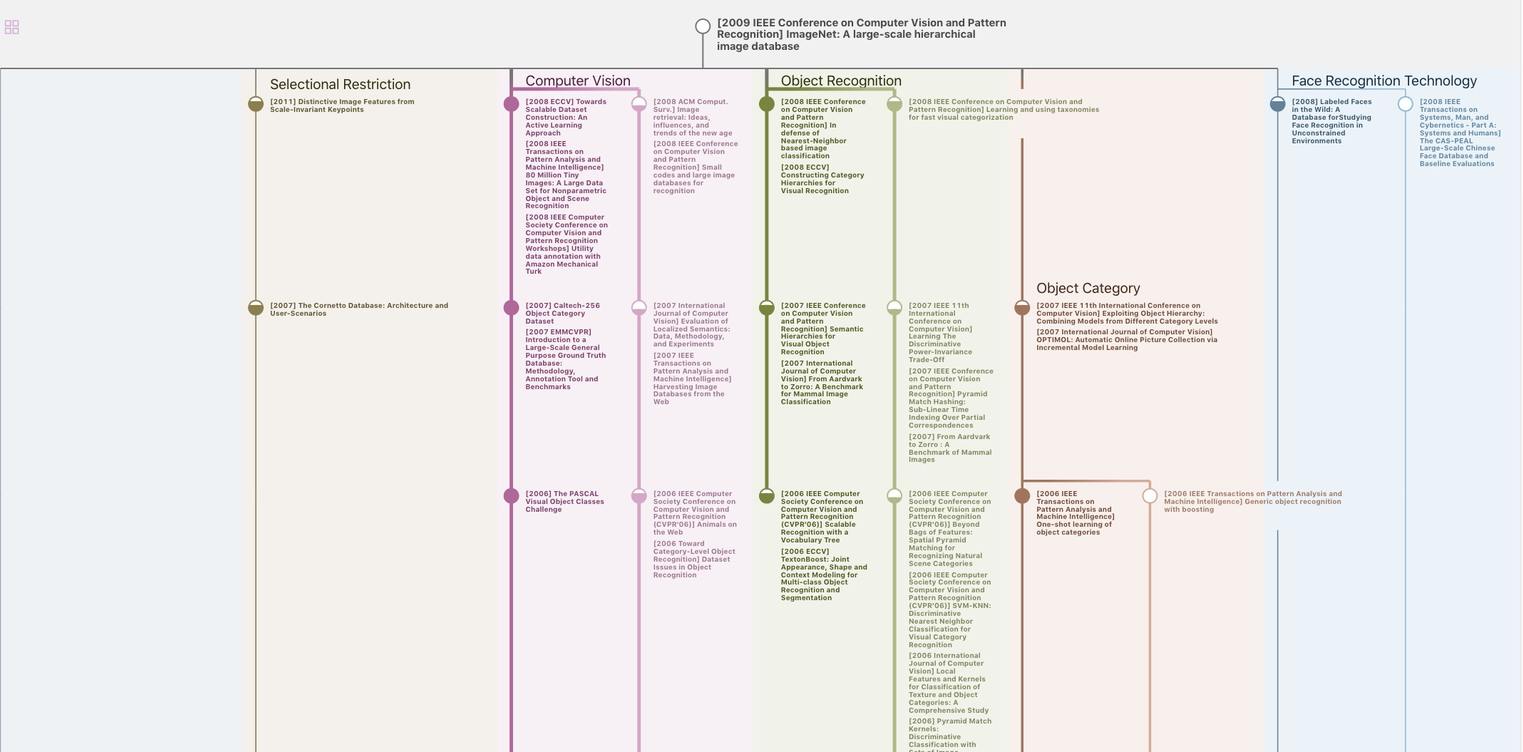
生成溯源树,研究论文发展脉络
Chat Paper
正在生成论文摘要