Determinants of Coronary Artery Calcium Score in Patients with Multifactorial Chylomicronemia Syndrome
Atherosclerosis(2023)
Hosp Civils Lyon | Hospices Civils Lyon | Carmen Laboratory
Abstract
Background and Aims: Coronary artery calcium score (CAC) improves cardiovascular (CV) risk prediction and is validated in many high risk populations such as type 1/type 2 diabetes and familial hypercholesterolemia. We aimed to characterize CAC distribution and determinants in patients with multifactorial hyperchylomicronemia syndrome (MCS) in primary CV prevention. Methods: Retrospective monocentric study, 164 MCS patients in primary prevention with a CAC assessment from 01/01/2011 to 31/08/2022. Results: The cohort had 79.3% of men. Mean age was 51.1±10.9 years. 48.2% of patients had type 2 diabetes, 45.1% hypertension (HTA), 34.7% had a history of acute pancreatitis. CAC median value was 1.0 (IQT 165) with the following distribution: CAC=0 47.1%, CAC 1- 100 23.2%, CAC 101-300 11.0% and CAC >300 18.9%. 48.2% of patients had CAC below the 25th percentile for age and sex and 34.1 % over the 75th. In univariate analysis, age, HTA, diabetes and smoking were significantly associated with a CAC >300 vs CAC=0 and with CAC >75th percentile vs <25th percentile. Sex and lipid parameters at the time of CAC assessment were not associated with the CAC score. In multivariate analysis (binary logistic regression), age, HTA, diabetes and smoking, remained significantly associated with CAC >300 vs CAC=0 and age, HTA, and smoking with CAC >75th percentile vs < 25th percentile. Conclusions: Traditional CV rick factors such as age, diabetes, HTA and smoking are the major determinants of CAC score. CAC may contribute to identify a subgroup of MCS patients at very high cardiovascular risk which might benefit for treatment intensification with new therapies.
MoreTranslated text
求助PDF
上传PDF
View via Publisher
AI Read Science
AI Summary
AI Summary is the key point extracted automatically understanding the full text of the paper, including the background, methods, results, conclusions, icons and other key content, so that you can get the outline of the paper at a glance.
Example
Background
Key content
Introduction
Methods
Results
Related work
Fund
Key content
- Pretraining has recently greatly promoted the development of natural language processing (NLP)
- We show that M6 outperforms the baselines in multimodal downstream tasks, and the large M6 with 10 parameters can reach a better performance
- We propose a method called M6 that is able to process information of multiple modalities and perform both single-modal and cross-modal understanding and generation
- The model is scaled to large model with 10 billion parameters with sophisticated deployment, and the 10 -parameter M6-large is the largest pretrained model in Chinese
- Experimental results show that our proposed M6 outperforms the baseline in a number of downstream tasks concerning both single modality and multiple modalities We will continue the pretraining of extremely large models by increasing data to explore the limit of its performance
Upload PDF to Generate Summary
Must-Reading Tree
Example
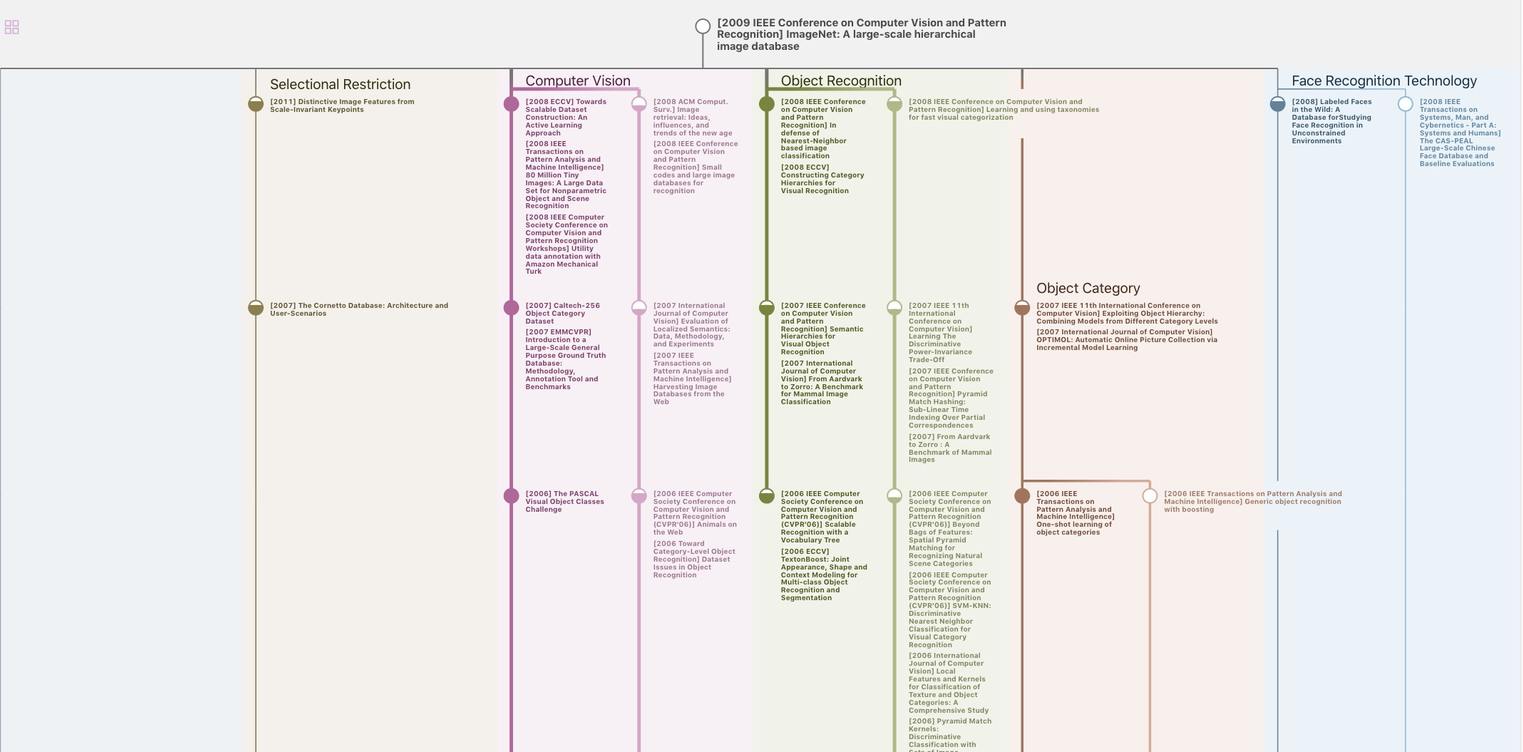
Generate MRT to find the research sequence of this paper
Data Disclaimer
The page data are from open Internet sources, cooperative publishers and automatic analysis results through AI technology. We do not make any commitments and guarantees for the validity, accuracy, correctness, reliability, completeness and timeliness of the page data. If you have any questions, please contact us by email: report@aminer.cn
Chat Paper
GPU is busy, summary generation fails
Rerequest