Digital twin-assisted imbalanced fault diagnosis framework using subdomain adaptive mechanism and margin-aware regularization
RELIABILITY ENGINEERING & SYSTEM SAFETY(2023)
摘要
The current data-level and algorithm-level based imbalanced fault diagnosis methods have respective limitations such as uneven data generation quality and excessive reliance on minority class information. In response to these limitations, this study proposes a novel digital twin-assisted framework for imbalanced fault diagnosis. The framework begins by analyzing the nonlinear kinetic characteristics of the gearbox and establishing a dynamic simulation model assisted by digital twin technology to generate high-fidelity simulated fault data. Subsequently, a subdomain adaptive mechanism is employed to align the conditional distribution of the subdomains by minimizing the dissimilarity of fine-grained features between the simulated and real-world fault data. To improve the fault tolerance of the model's diagnosis, margin-aware regularization is designed by applying significant regularization penalties to the fault data margins. Experimental results from two gearboxes demonstrate that, compared to the recent data-level and algorithm-level based imbalanced fault diagnosis methods, the proposed framework holds distinct advantages under the influence of highly imbalanced data, offering a fresh perspective for addressing this challenging scenario. In addition, the effectiveness of subdomain adaptive mechanism and margin-aware regularization is verified through the ablation experiment.
更多查看译文
关键词
Digital twin,Imbalanced fault diagnosis,Subdomain adaptive mechanism,Margin-aware regularization,Gearbox
AI 理解论文
溯源树
样例
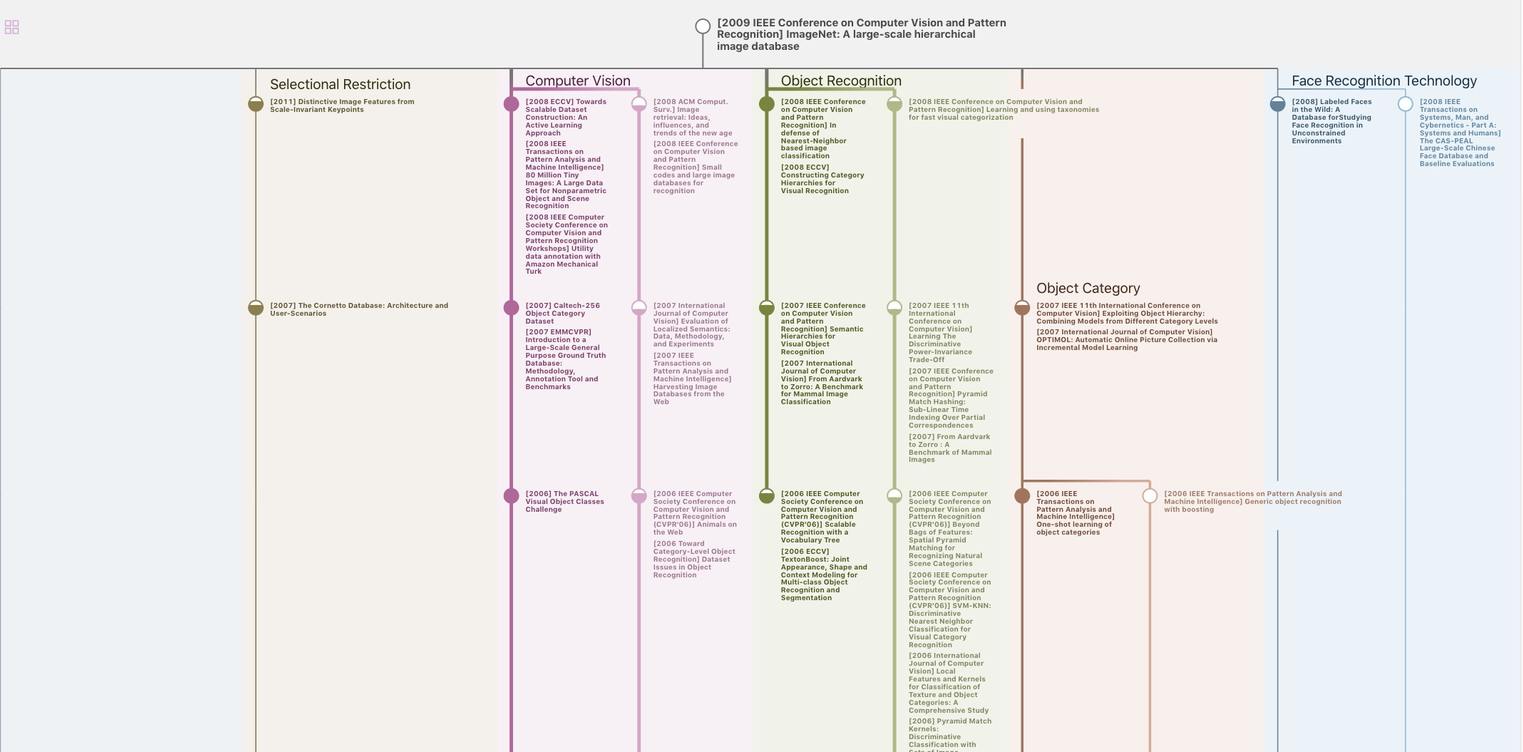
生成溯源树,研究论文发展脉络
Chat Paper
正在生成论文摘要