Cyberbullying detection framework for short and imbalanced Arabic datasets
JOURNAL OF KING SAUD UNIVERSITY-COMPUTER AND INFORMATION SCIENCES(2023)
摘要
Cyberbullying detection has attracted many researchers to detect negative comments deployed on communication platforms as cyberbullying can take many forms: verbal, implicit, explicit, or even nonverbal. The successful growth of social media in recent years has opened new perspectives on the detection of cyberbullying, although related research still encounters several challenges, such as data imbalance and expression implicitness. In this paper, we propose an automated cyberbullying detection framework designed to produce satisfactory results, especially when imbalanced short text and different dialects exist in the Arabic text data. In the proposed framework a new method to solve the imbalance problem is suggested, where the modified simulated annealing optimization algorithm is used to find the optimal set of samples from the majority class to balance the training set. This method has been evaluated using traditional machine learning algorithms including support vector machine, and deep learning algorithms including Long Short-Term Memory (LSTM) and Bidirectional LSTM (Bi-LSTM). To generate a framework that can detect Arabic written cyberbullying on communication platforms, the accuracy, recall, specificity, sensitivity and mean squared error are used as the main performance indicators. The results indicate that the proposed framework can improve the performance of the tested algorithms, and Bi-LSTM outperforms other methods for cyberbullying classification.(c) 2023 The Author(s). Published by Elsevier B.V. on behalf of King Saud University. This is an open access article under the CC BY-NC-ND license (http://creativecommons.org/licenses/by-nc-nd/4.0/).
更多查看译文
关键词
Arabic cyberbullying detection,Imbalance data,Machine learning,Deep learning,Optimization,Sample selection
AI 理解论文
溯源树
样例
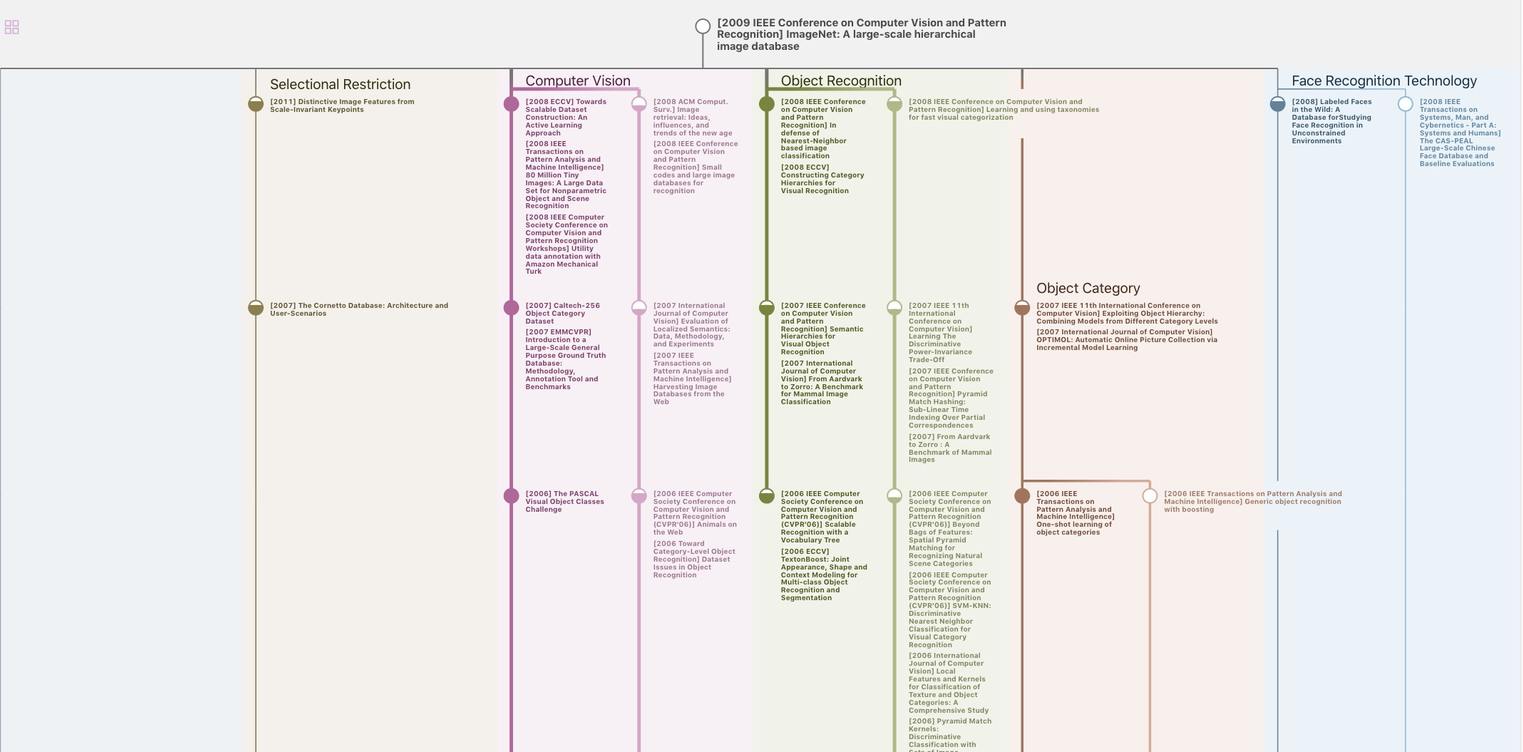
生成溯源树,研究论文发展脉络
Chat Paper
正在生成论文摘要