Patient-Specific Analysis of Co-expression Networks for Predicting Clinical Outcomes in Breast Cancer
bioRxiv (Cold Spring Harbor Laboratory)(2023)
Abstract
To effectively understand the underlying mechanisms of disease and inform the development of personalized therapies, it is critical to harness the power of differential co-expression network analysis. Despite the promise of differential co-expression network analysis in precision medicine, current approaches have a major limitation: they measure an average differential network across multiple samples, which means the specific etiology of individual patients is often overlooked. To address this, we present Cosinet, a differential co-expression-based single-sample network rewiring degree quantification tool. By analyzing two breast cancer datasets, we demonstrate that Cosinet can identify important differences in gene co-expression patterns between individual patients and generate scores for each individual that are significantly associated with overall survival, recurrence-free interval, and other clinical outcomes, even after adjusting for risk factors such as age, tumor size, HER2 status and PAM50 subtypes. Cosinet represents a remarkable development toward unlocking the potential of differential co-expression analysis in the context of precision medicine.
### Competing Interest Statement
The authors have declared no competing interest.
MoreTranslated text
Key words
breast cancer,networks,clinical outcomes,patient-specific,co-expression
AI Read Science
Must-Reading Tree
Example
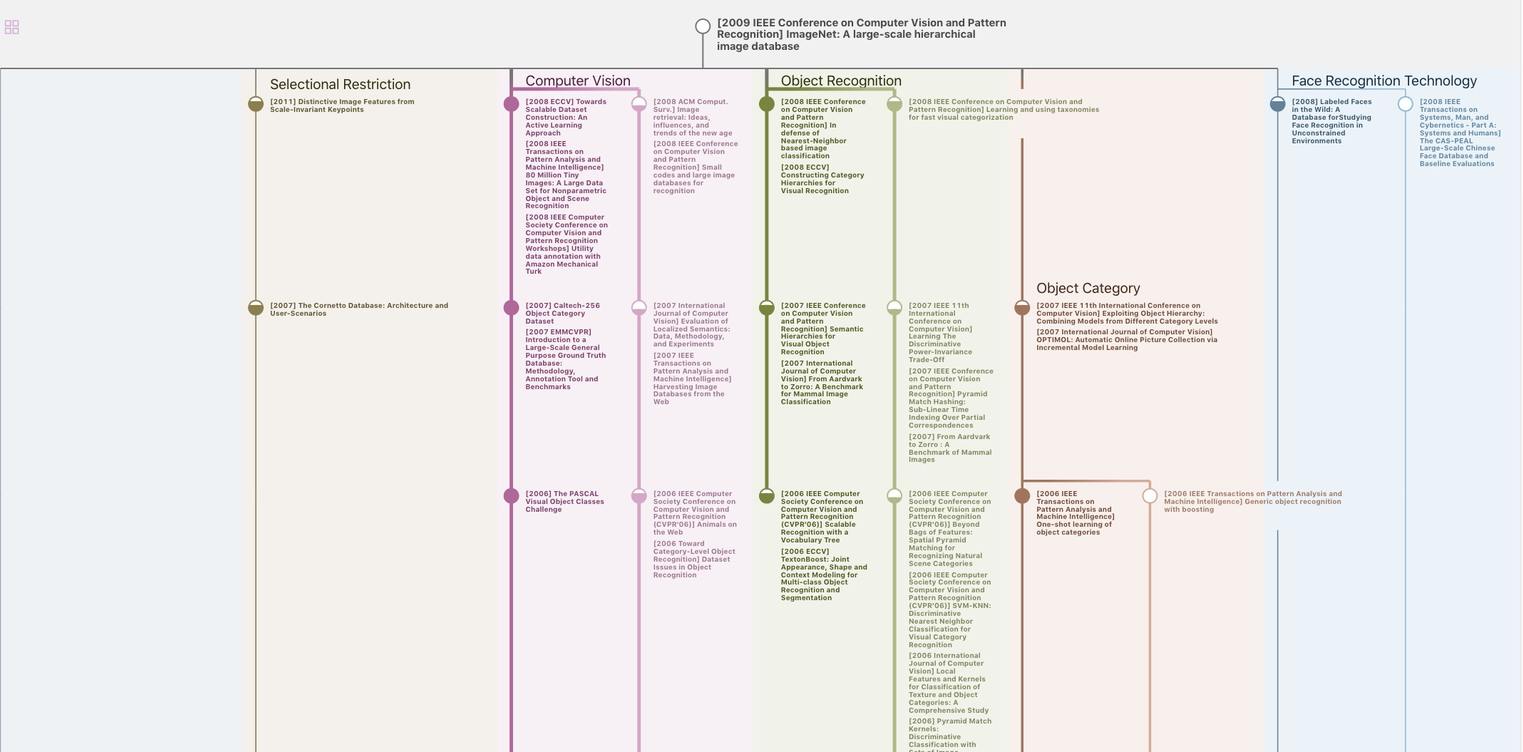
Generate MRT to find the research sequence of this paper
Chat Paper
Summary is being generated by the instructions you defined