Cytosolic RGG RNA-binding Proteins Are Temperature Sensitive Flowering Time Regulators in Arabidopsis
Biological Chemistry(2023)SCI 3区SCI 4区
Abstract
mRNA translation is tightly regulated by various classes of RNA-binding proteins (RBPs) during development and in response to changing environmental conditions. In this study, we characterize the arginine-glycine-glycine (RGG) motif containing RBP family of Arabidopsis thaliana representing homologues of the multifunctional translation regulators and ribosomal preservation factors Stm1 from yeast (ScStm1) and human SERBP1 (HsSERBP1). The Arabidopsis genome encodes three RGG proteins named AtRGGA, AtRGGB and AtRGGC. While AtRGGA is ubiquitously expressed, AtRGGB and AtRGGC are enriched in dividing cells. All AtRGGs localize almost exclusively to the cytoplasm and bind with high affinity to ssRNA, while being capable to interact with most nucleic acids, except dsRNA. A protein-interactome study shows that AtRGGs interact with ribosomal proteins and proteins involved in RNA processing and transport. In contrast to ScStm1, AtRGGs are enriched in ribosome-free fractions in polysome profiles, suggesting additional plant-specific functions. Mutant studies show that AtRGG proteins differentially regulate flowering time, with a distinct and complex temperature dependency for each AtRGG protein. In conclusion, we suggest that AtRGGs function in fine-tuning translation efficiency to control flowering time and potentially other developmental processes in response to environmental changes.
MoreTranslated text
Key words
mRNA binding,RBP,RGG motif,ribosome,SERBP1,Stm1
求助PDF
上传PDF
View via Publisher
AI Read Science
AI Summary
AI Summary is the key point extracted automatically understanding the full text of the paper, including the background, methods, results, conclusions, icons and other key content, so that you can get the outline of the paper at a glance.
Example
Background
Key content
Introduction
Methods
Results
Related work
Fund
Key content
- Pretraining has recently greatly promoted the development of natural language processing (NLP)
- We show that M6 outperforms the baselines in multimodal downstream tasks, and the large M6 with 10 parameters can reach a better performance
- We propose a method called M6 that is able to process information of multiple modalities and perform both single-modal and cross-modal understanding and generation
- The model is scaled to large model with 10 billion parameters with sophisticated deployment, and the 10 -parameter M6-large is the largest pretrained model in Chinese
- Experimental results show that our proposed M6 outperforms the baseline in a number of downstream tasks concerning both single modality and multiple modalities We will continue the pretraining of extremely large models by increasing data to explore the limit of its performance
Upload PDF to Generate Summary
Must-Reading Tree
Example
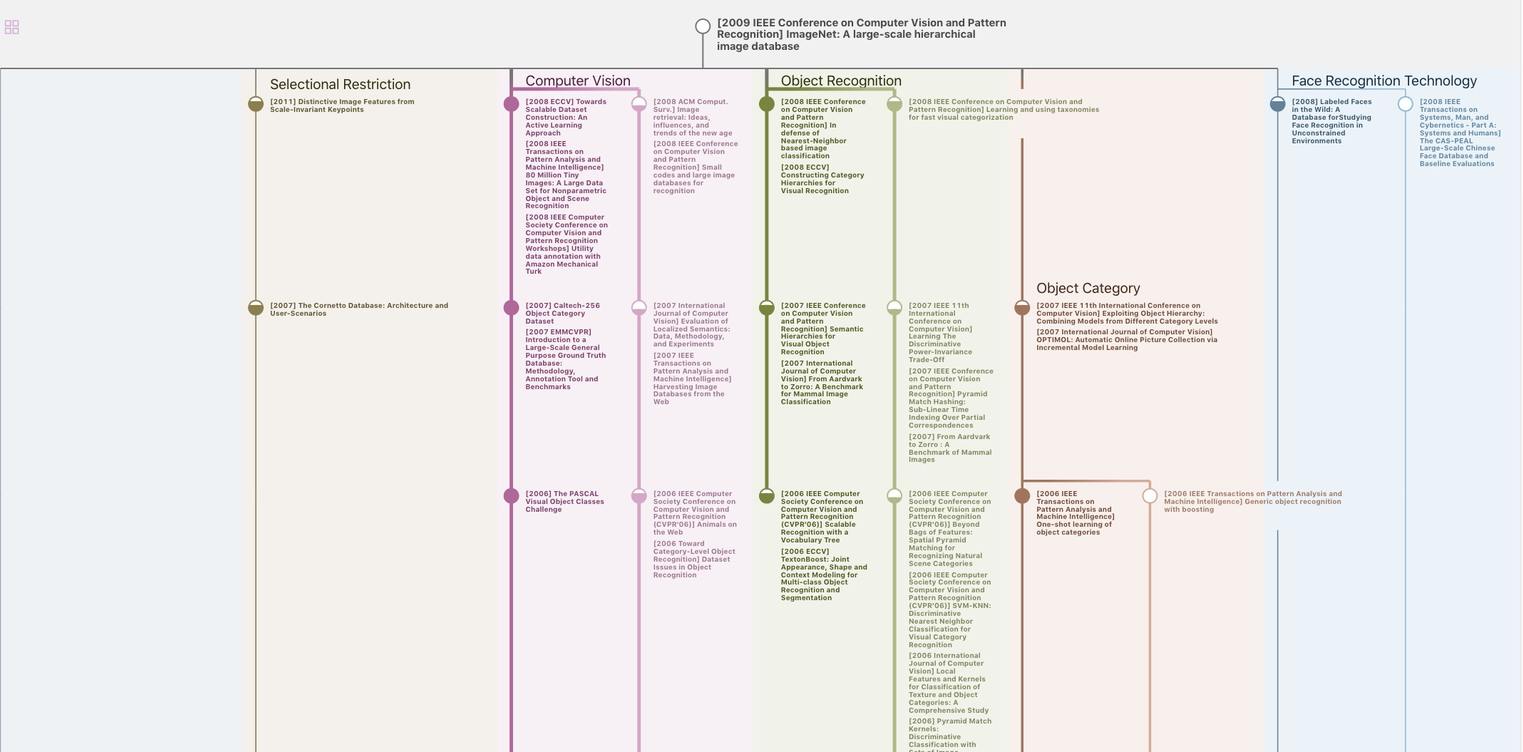
Generate MRT to find the research sequence of this paper
Related Papers
Data Disclaimer
The page data are from open Internet sources, cooperative publishers and automatic analysis results through AI technology. We do not make any commitments and guarantees for the validity, accuracy, correctness, reliability, completeness and timeliness of the page data. If you have any questions, please contact us by email: report@aminer.cn
Chat Paper