FastSpanNER: Speeding Up SpanNER by Named Entity Head Prediction
2023 5th International Conference on Natural Language Processing (ICNLP)(2023)
摘要
Named Entity Recognition (NER) is one of the most fundamental tasks in natural language processing (NLP). Different from the widely-used sequence labeling framework in NER, span prediction based methods are more naturally suitable for the nested NER problem and have received a lot of attention recently. However, classifying the samples generated by traversing all sub-sequences is computational expensive during training and very ineffective at inference. In this paper, we propose the FastSpanNER approach to reduce the computation of both training and inferring. We introduce a task of Named Entity Head (NEH) prediction for each word in given sequence, and perform multi-task learning together with the task of span classification, which uses no more than half of the samples in SpanNER. In the inference phase, only the words predicted as NEHs are used to generate candidate spans for named entity classification. Experimental results on the four standard benchmark datasets (CoNLL2003, MSRA, CNERTA and GENIA) show that our FastSpanNER method not only greatly reduces the computation of training and inferring but also achieves better F1 scores compared with the SpanNER method.
更多查看译文
关键词
FastSpanNER,SpanNER,Named Entity Head Prediction,Span Classification,Multi-task Learning
AI 理解论文
溯源树
样例
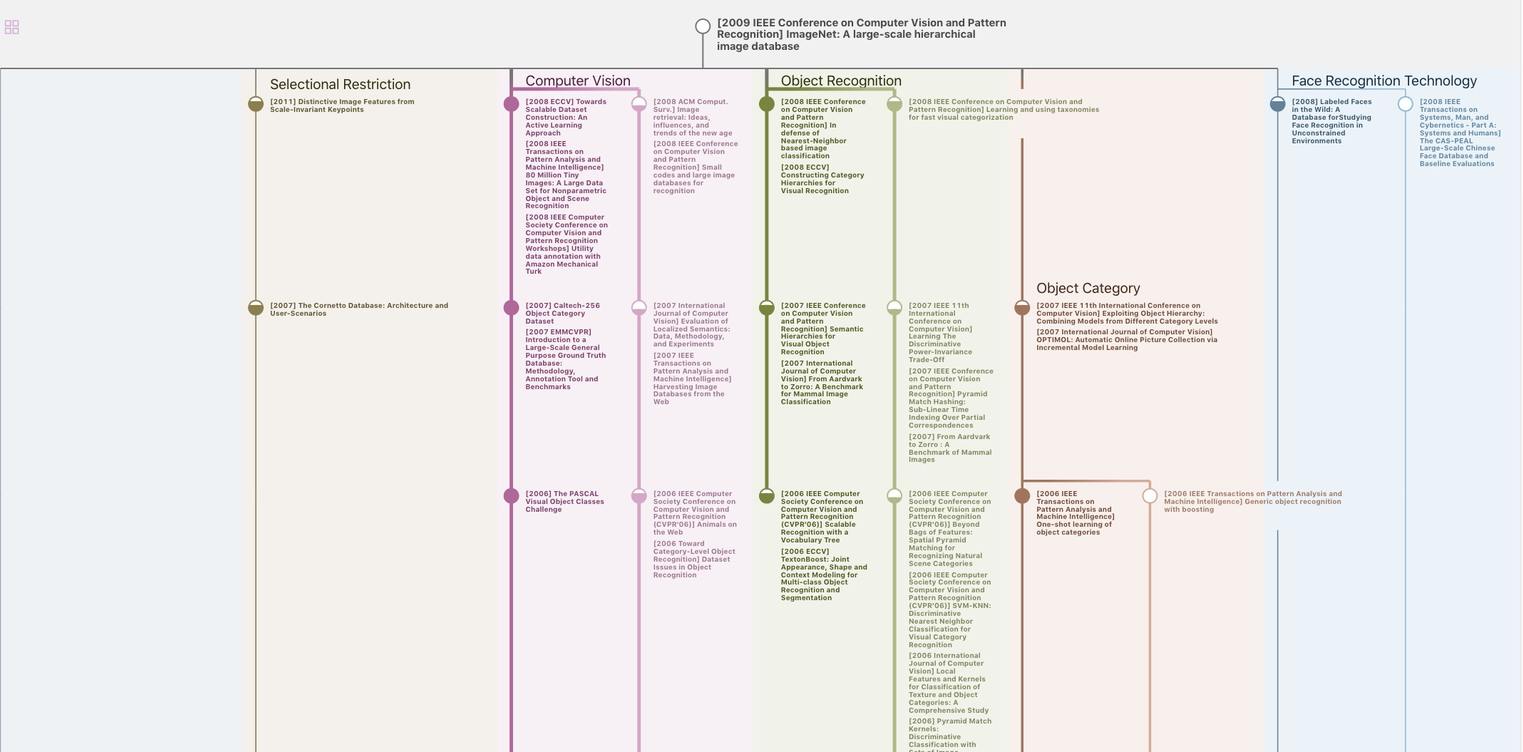
生成溯源树,研究论文发展脉络
Chat Paper
正在生成论文摘要