A Deep Neural Network Architecture for Reliable 3D Position and Size Determination for Lagrangian Particle Tracking Using a Single Camera
MEASUREMENT SCIENCE AND TECHNOLOGY(2023)
摘要
Microfluidic flows feature typically fully three-dimensional velocity fields. However, often the optical access for measurements is limited. Astigmatism or defocus particle tracking velocimetry is a technique that enables the 3D position determination of individual particles by the analysis of astigmatic/defocused particle images. The classification and position determination of particles is a task well suited to deep neural networks (DNNs). In this work, two DNNs are used to extract the class and in-plane position (object detection) as well as the depth position (regression). The performance of both DNNs is assessed by the position uncertainties as well as the precision of the size classes and the amount of recalled particles. The DNNs are evaluated on a synthetic dataset and establish a new benchmark of DNNs in defocus tracking applications. The recall is higher than compared to classic methods and the in-plane errors are always subpixel accurate. The relative uncertainty in the depth position is below 1% for all examined particle seeding concentrations. Additionally, the performance on experimental images, using four different particle sizes, ranging from 1.14 mu m to 5.03 mu m is analyzed. The particle images are systematically rearranged to produce comprehensive datasets of varying particle seeding concentrations. The distinction between particles of similar size is more challenging but the DNNs still show very good results. A precision above 96% is reached with a high recall above 95%. The error in the depth position remains below 1% and the in-plane errors are subpixel accurate with respect to the labels. The work shows that first, DNNs can be trained with artificially rearranged data sets based on individual experimental images and are therefore easily adaptable to various experimental setups and applicable by non-experts. Second, the DNNs can be successfully adapted to determine additional variables as in this case the size of the suspended particles.
更多查看译文
关键词
astigmatism particle tracking velocimetry,Lagrangian particle tracking,size recognition,deep neural network,microfluidics,size classification
AI 理解论文
溯源树
样例
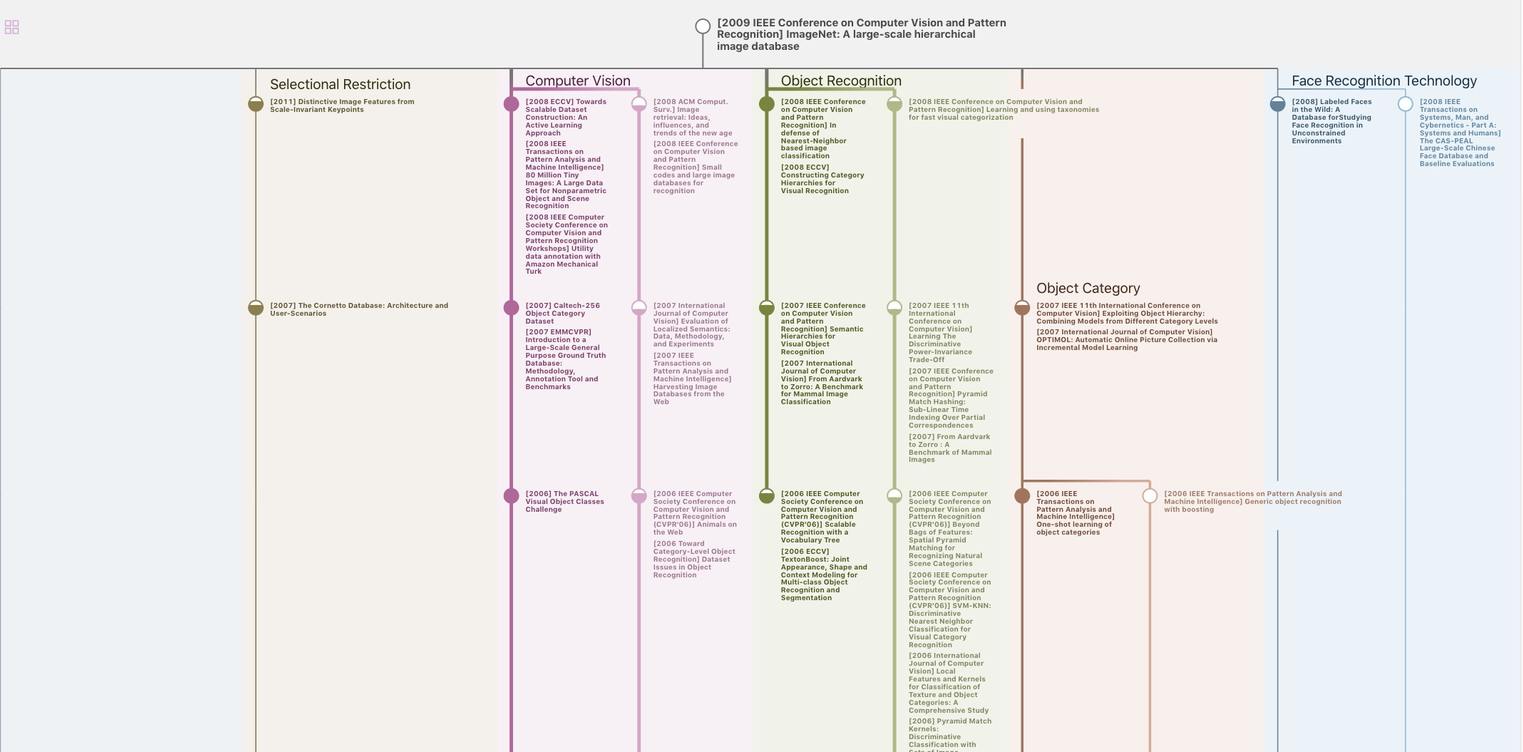
生成溯源树,研究论文发展脉络
Chat Paper
正在生成论文摘要