On Learning Agent-Based Models from Data
SCIENTIFIC REPORTS(2023)
摘要
Agent-Based Models (ABMs) are used in several fields to study the evolution of complex systems from micro-level assumptions. However, a significant drawback of ABMs is their inability to estimate agent-specific (or "micro") variables, which hinders their ability to make accurate predictions using micro-level data. In this paper, we propose a protocol to learn the latent micro-variables of an ABM from data. We begin by translating an ABM into a probabilistic model characterized by a computationally tractable likelihood. Next, we use a gradient-based expectation maximization algorithm to maximize the likelihood of the latent variables. We showcase the efficacy of our protocol on an ABM of the housing market, where agents with different incomes bid higher prices to live in high-income neighborhoods. Our protocol produces accurate estimates of the latent variables while preserving the general behavior of the ABM. Moreover, our estimates substantially improve the out-of-sample forecasting capabilities of the ABM compared to simpler heuristics. Our protocol encourages modelers to articulate assumptions, consider the inferential process, and spot potential identification problems, thus making it a useful alternative to black-box data assimilation methods.
更多查看译文
关键词
Agent-Based Modeling
AI 理解论文
溯源树
样例
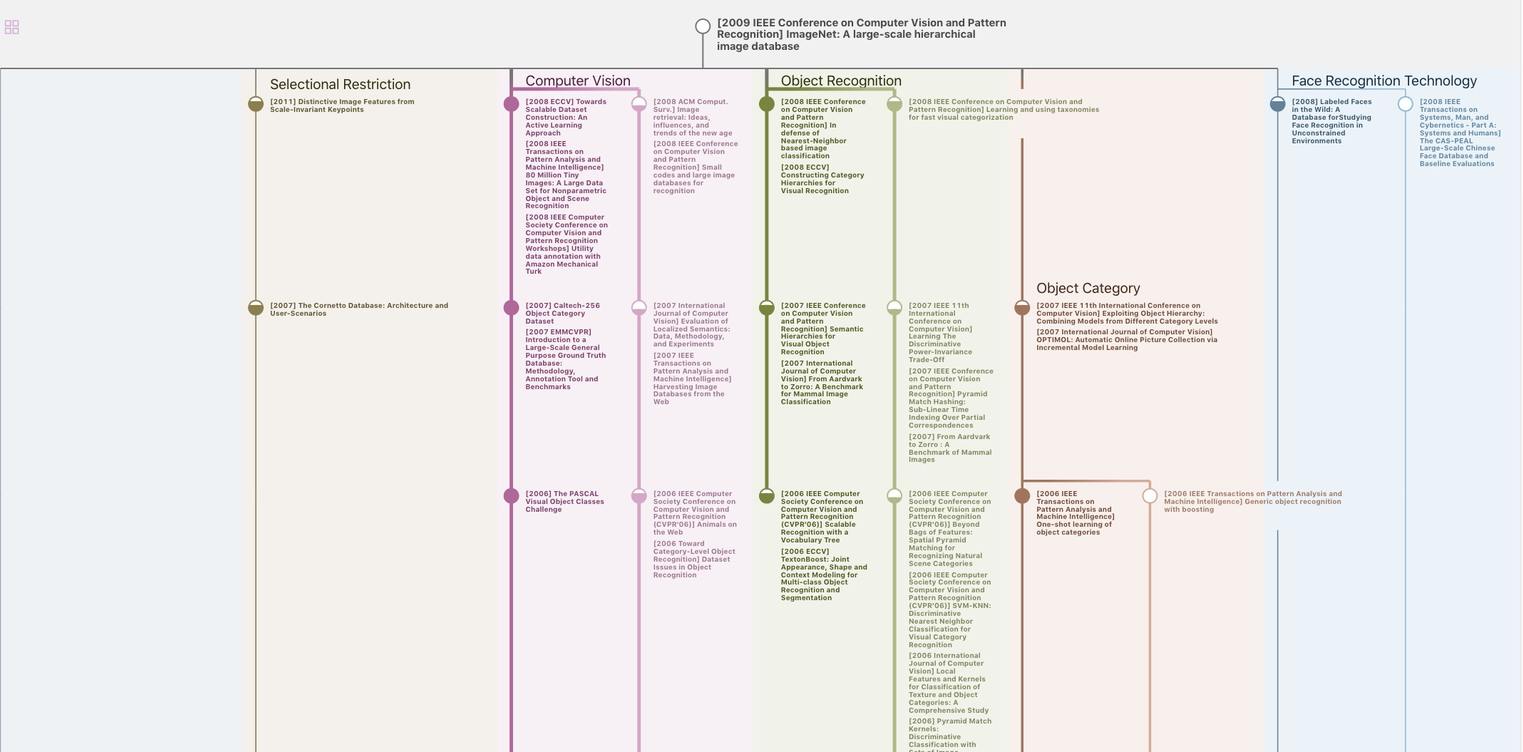
生成溯源树,研究论文发展脉络
Chat Paper
正在生成论文摘要