Negligible Magnetic Losses at Low Temperatures in Liquid Phase Epitaxy Grown Y3Fe5O12 Films
PHYSICAL REVIEW MATERIALS(2023)
Northeastern Univ | Cornell Univ | Oak Ridge Natl Lab | Natl Inst Stand & Technol | INNOVENT e V Technologieentwicklung | Sandia Natl Labs
Abstract
Yttrium iron garnet (Y3Fe5O12; YIG) has a unique combination of low magnetic damping, high spin-wave conductivity, and insulating properties that make it a highly attractive material for a variety of applications in the fields of magnetics and spintronics. While the room-temperature magnetization dynamics of YIG have been extensively studied, there are limited reports correlating the low-temperature magnetization dynamics to the material structure or growth method. Here we investigate liquid phase epitaxy grown YIG films and their magnetization dynamics at temperatures down to 10 K. We show there is a negligible increase in the ferromagnetic resonance linewidth down to 10 K, which is unique when compared with YIG films grown by other deposition methods. From the broadband ferromagnetic resonance measurements, polarized neutron reflectivity, and scanning transmission electron microscopy, we conclude that these liquid phase epitaxy grown films have negligible rare-earth impurities present, specifically the suppression of Gd diffusion from the Gd3Ga5O12 (GGG) substrate into the Y3Fe5O12 film, and therefore negligible magnetic losses attributed to the slow-relaxation mechanism. Overall, liquid phase epitaxy YIG films have a YIG/GGG interface that is five times sharper and have ten times lower ferromagnetic resonance linewidths below 50 K than comparable YIG films by other deposition methods. Thus, liquid phase epitaxy grown YIG films are ideal for low-temperature experiments/applications that require low magnetic losses, such as quantum transduction and manipulation via magnon coupling.
MoreTranslated text
Key words
Yttrium Iron Garnet
求助PDF
上传PDF
View via Publisher
AI Read Science
AI Summary
AI Summary is the key point extracted automatically understanding the full text of the paper, including the background, methods, results, conclusions, icons and other key content, so that you can get the outline of the paper at a glance.
Example
Background
Key content
Introduction
Methods
Results
Related work
Fund
Key content
- Pretraining has recently greatly promoted the development of natural language processing (NLP)
- We show that M6 outperforms the baselines in multimodal downstream tasks, and the large M6 with 10 parameters can reach a better performance
- We propose a method called M6 that is able to process information of multiple modalities and perform both single-modal and cross-modal understanding and generation
- The model is scaled to large model with 10 billion parameters with sophisticated deployment, and the 10 -parameter M6-large is the largest pretrained model in Chinese
- Experimental results show that our proposed M6 outperforms the baseline in a number of downstream tasks concerning both single modality and multiple modalities We will continue the pretraining of extremely large models by increasing data to explore the limit of its performance
Upload PDF to Generate Summary
Must-Reading Tree
Example
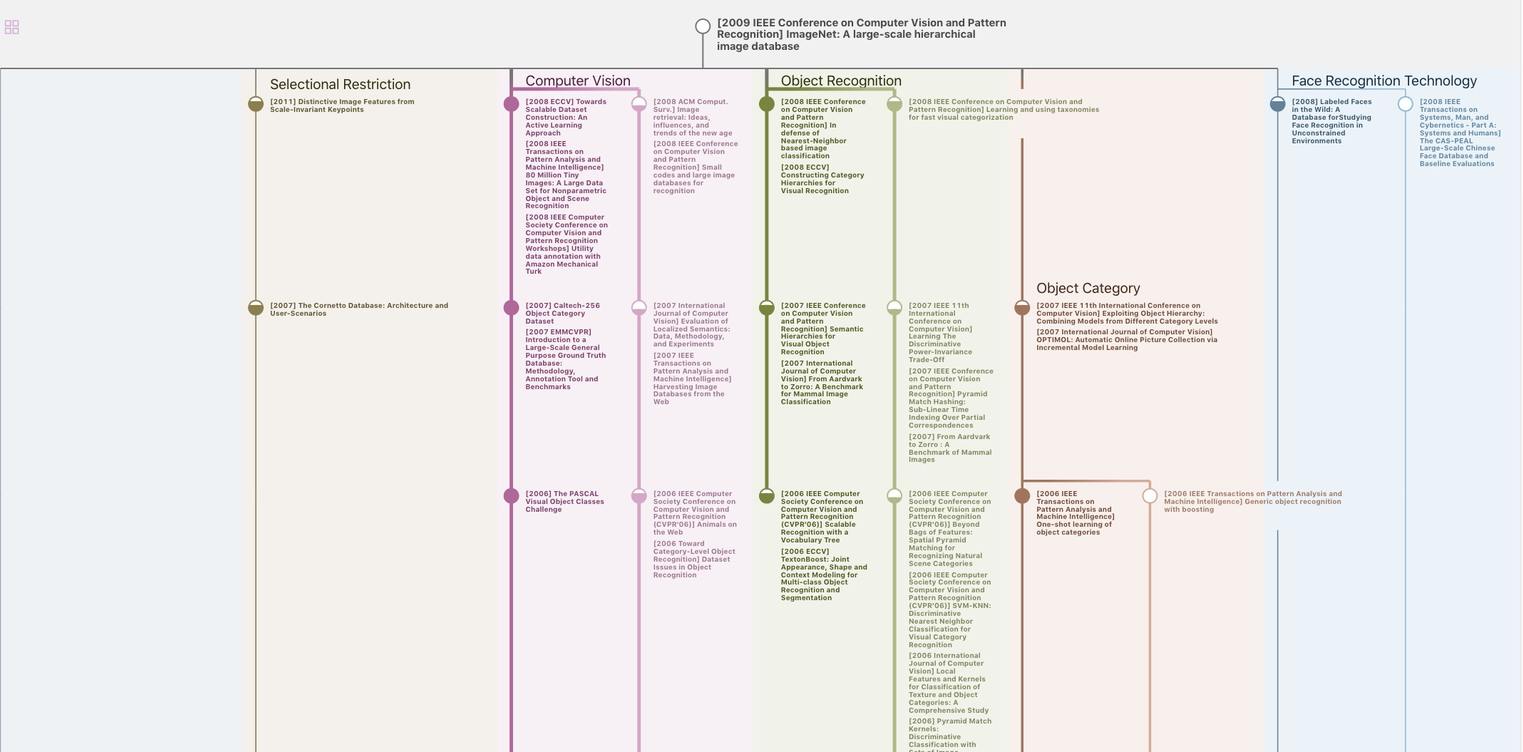
Generate MRT to find the research sequence of this paper
Related Papers
2010
被引用1829 | 浏览
2002
被引用16 | 浏览
1976
被引用25 | 浏览
2014
被引用193 | 浏览
2014
被引用151 | 浏览
2013
被引用95 | 浏览
2012
被引用291 | 浏览
2016
被引用48 | 浏览
2016
被引用29 | 浏览
2017
被引用46 | 浏览
2018
被引用35 | 浏览
2018
被引用46 | 浏览
2019
被引用15 | 浏览
Data Disclaimer
The page data are from open Internet sources, cooperative publishers and automatic analysis results through AI technology. We do not make any commitments and guarantees for the validity, accuracy, correctness, reliability, completeness and timeliness of the page data. If you have any questions, please contact us by email: report@aminer.cn
Chat Paper
GPU is busy, summary generation fails
Rerequest