An adaptive selective ensemble algorithm for fault classification
MEASUREMENT SCIENCE AND TECHNOLOGY(2023)
摘要
With the aim of improving the classification performance of a single extreme learning machine (ELM) for fault diagnosis, an adaptive selective ensemble classification algorithm is proposed based on the idea of ensemble learning. The proposed algorithm achieves better classification performance than a single model and the selected ELM subnetworks are selected adaptively and the ensemble weights of selected ELM subnetworks are calculated adaptively, which avoids the complex process of manually selecting subnetworks and calculating ensemble weights. First, a regularized error weight adjustment ELM is constructed by introducing regularization parameters and error weight adjustment matrix to a standard ELM, where the error weight adjustment matrix is obtained by the method of adaptive Gaussian kernel density estimation. Then, discrepancy subnetworks are constructed using six different activation functionsand the ensemble weights of subnetworks are obtained adaptively according to the normalized ratio of mean and variance of subnetwork F-scores to achieve the ensemble of subnetworks. Finally, the adaptive selective ensemble classification algorithm is validated using the UCI dataset and experimental acoustic emission signals of gearbox faults. The results show that the adaptive selective ensemble method can improve the stability and accuracy of classification algorithms and the achieved classification accuracy for experimental acoustic emission was 0.9773.
更多查看译文
关键词
fault diagnosis,adaptive Gaussian kernel density estimation,adaptive selective ensemble,ELM
AI 理解论文
溯源树
样例
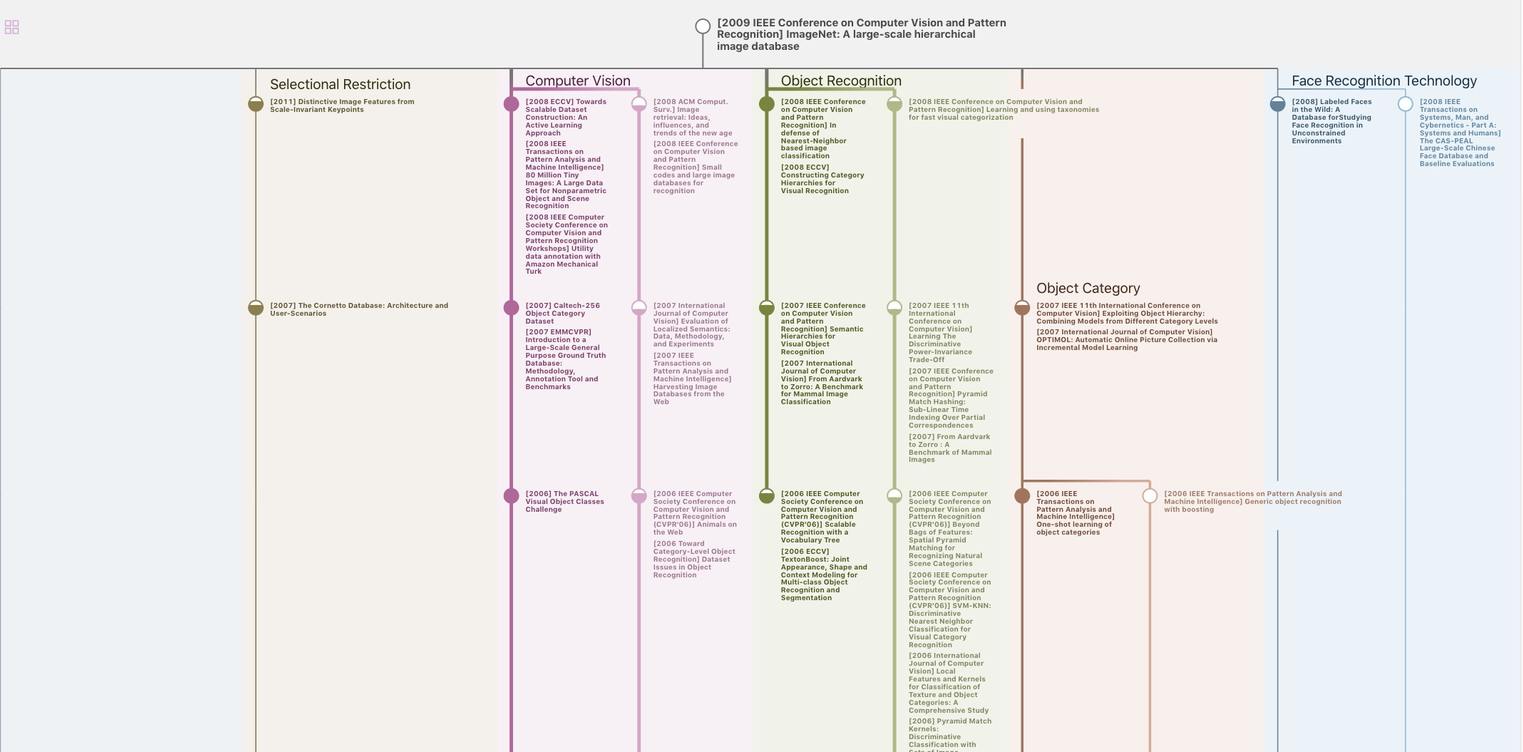
生成溯源树,研究论文发展脉络
Chat Paper
正在生成论文摘要