A discriminative multiple-manifold network for image set classification
APPLIED INTELLIGENCE(2023)
摘要
Because the distinct advantages of manifold-learning methods for feature extraction, Riemannian manifolds have been used extensively in image recognition tasks in recent years. However, large intra-class variation is a major problem for researchers. Although recent studies have alleviated this problem by extending nonlinear learning to Riemannian manifolds, important classification information may be lost when describing image sets with a single manifold. To address this problem, we design a novel discriminative multiple-manifold network (DMMNet) for image recognition. The Grassmann and symmetric positive definite manifold are first used simultaneously to model each image set. Then, the symmetric positive definite manifold and Grassmann manifold nonlinear learning blocks are devised to transform the data into low-dimensional manifold matrices to enhance their discriminability. A feature fusion layer is designed to fuse the complementary features of different manifolds, and a point-to-point hidden layer is devised to focus on more important feature maps. To acquire the importance of the manifold feature maps, a feature selection method based on manifold feature importance is designed to learn the corresponding weight coefficients. Finally, an output layer is used to map the optimal feature subset into the Euclidean space, such that the kernel discriminant analysis and K-nearest neighbor algorithm are applied. The proposed DMMNet is compared with state-of-the-art techniques on four datasets. Extensive experimental results demonstrate the effectiveness of the proposed DMMNet.
更多查看译文
关键词
classification,multiple-manifold
AI 理解论文
溯源树
样例
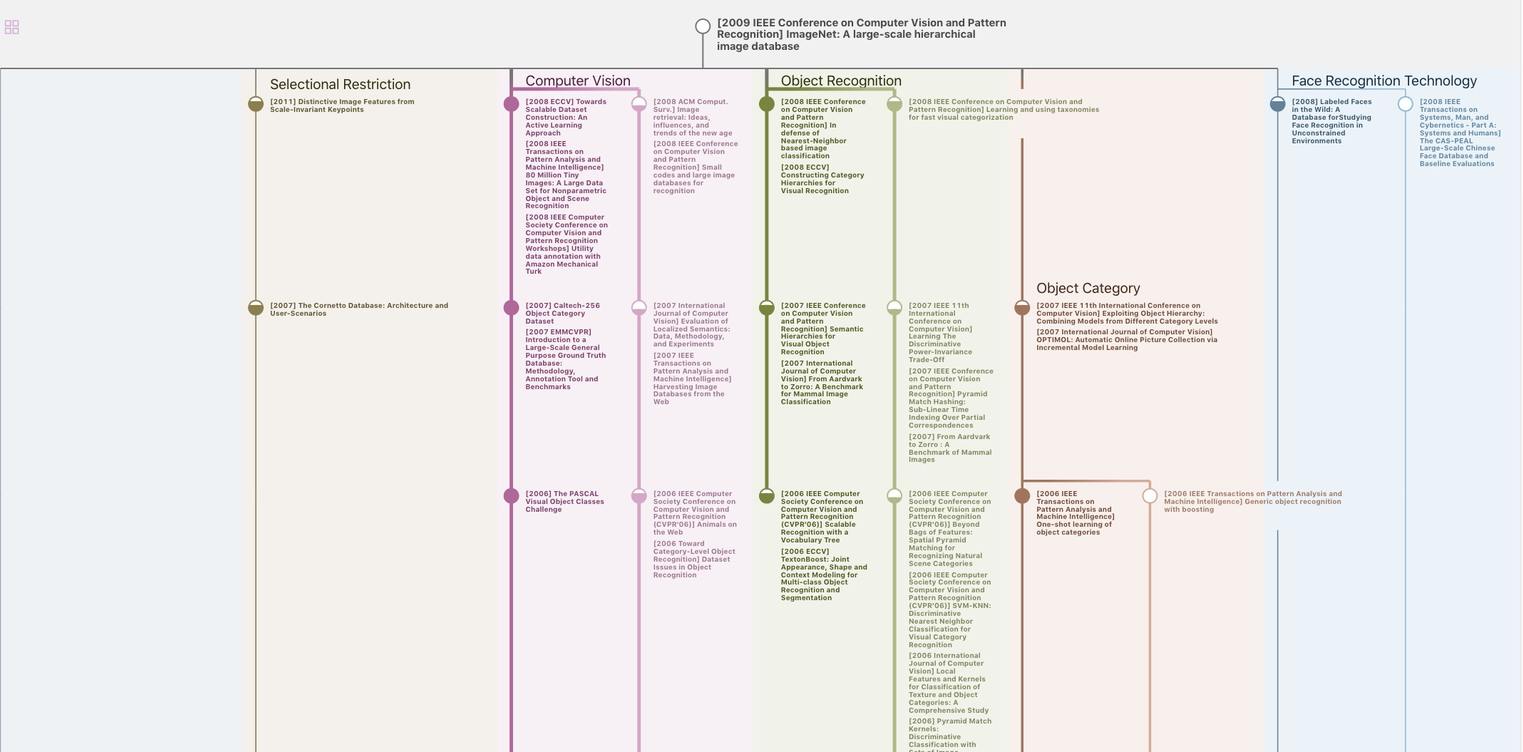
生成溯源树,研究论文发展脉络
Chat Paper
正在生成论文摘要