Empirical approximation of the gaussian distribution in $\mathbb{R}^d$
arXiv (Cornell University)(2023)
Abstract
Let $G_1,\dots,G_m$ be independent copies of the standard gaussian random vector in $\mathbb{R}^d$. We show that there is an absolute constant $c$ such that for any $A \subset S^{d-1}$, with probability at least $1-2\exp(-c\Delta m)$, for every $t\in\mathbb{R}$, \[ \sup_{x \in A} \left| \frac{1}{m}\sum_{i=1}^m 1_{ \{\langle G_i,x\rangle \leq t \}} - \mathbb{P}(\langle G,x\rangle \leq t) \right| \leq \Delta + \sigma(t) \sqrt\Delta. \] Here $\sigma(t) $ is the variance of $1_{\{\langle G,x\rangle\leq t\}}$ and $\Delta\geq \Delta_0$, where $\Delta_0$ is determined by an unexpected complexity parameter of $A$ that captures the set's geometry (Talagrand's $\gamma_1$ functional). The bound, the probability estimate, and the value of $\Delta_0$ are all (almost) optimal. We use this fact to show that if $\Gamma=\sum_{i=1}^m \langle G_i,x\rangle e_i$ is the random matrix that has $G_1,\dots,G_m$ as its rows, then the structure of $\Gamma(A)=\{\Gamma x: x\in A\}$ is far more rigid and well-prescribed than was previously expected.
MoreTranslated text
Key words
empirical approximation,gaussian distribution
AI Read Science
Must-Reading Tree
Example
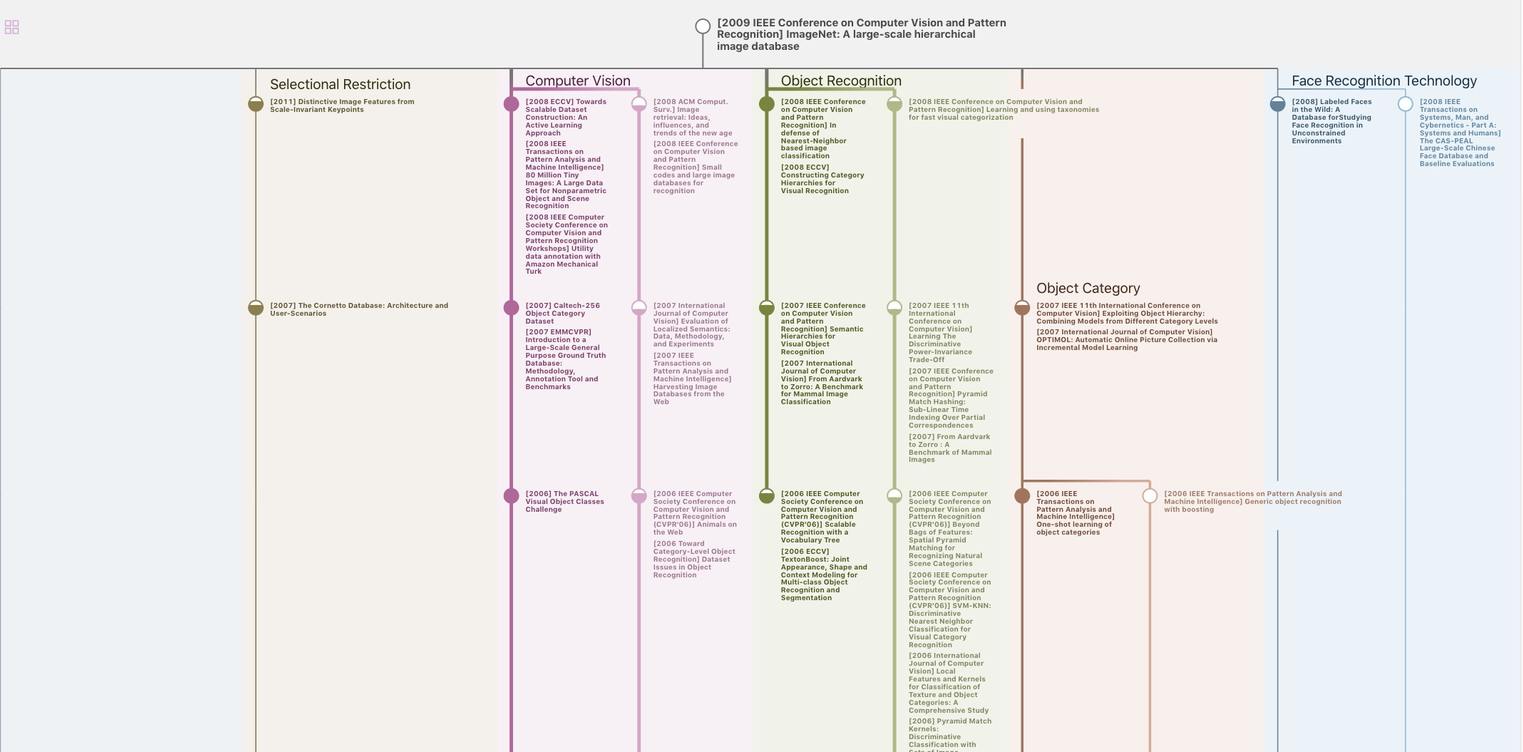
Generate MRT to find the research sequence of this paper
Chat Paper
Summary is being generated by the instructions you defined