Advancing the Lower Bounds: an Accelerated, Stochastic, Second-Order Method with Optimal Adaptation to Inexactness
ICLR(2024)
摘要
We present a new accelerated stochastic second-order method that is robust to both gradient and Hessian inexactness, typical in machine learning. We establish theoretical lower bounds and prove that our algorithm achieves optimal convergence in both gradient and Hessian inexactness in this key setting. We further introduce a tensor generalization for stochastic higher-order derivatives. When the oracles are non-stochastic, the proposed tensor algorithm matches the global convergence of Nesterov Accelerated Tensor method. Both algorithms allow for approximate solutions of their auxiliary subproblems with verifiable conditions on the accuracy of the solution.
更多查看译文
关键词
Second-order methods,convex optimization,stochastic optimization,Cubic Newton Method,high-order methods,tensor methods,lower bounds
AI 理解论文
溯源树
样例
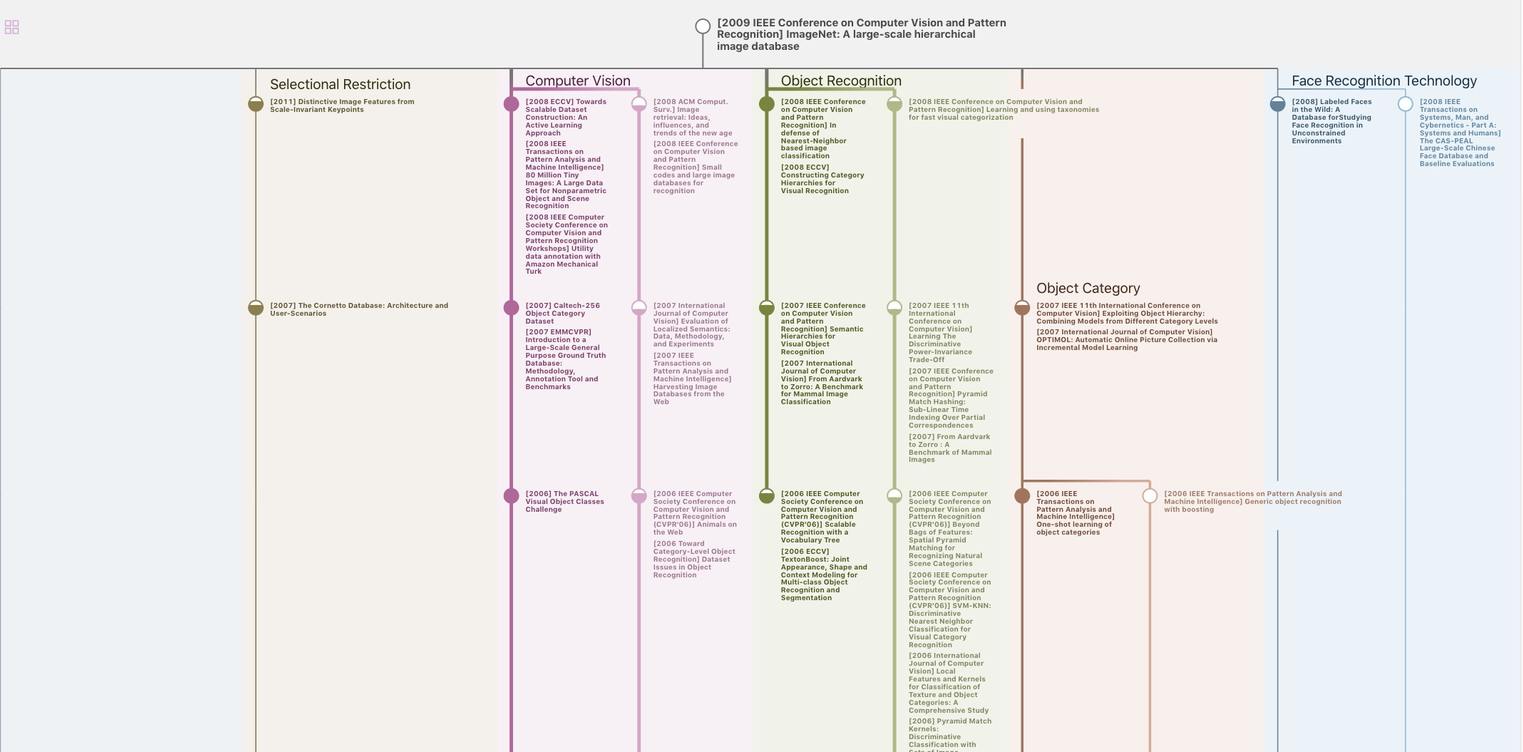
生成溯源树,研究论文发展脉络
Chat Paper
正在生成论文摘要