Zero-shot information extraction from radiological reports using ChatGPT
INTERNATIONAL JOURNAL OF MEDICAL INFORMATICS(2024)
摘要
Introduction: Electronic health records contain an enormous amount of valuable information recorded in free text. Information extraction is the strategy to transform free text into structured data, but some of its components require annotated data to tune, which has become a bottleneck. Large language models achieve good performances on various downstream NLP tasks without parameter tuning, becoming a possible way to extract information in a zero-shot manner. Methods: In this study, we aim to explore whether the most popular large language model, ChatGPT, can extract information from the radiological reports. We first design the prompt template for the interested information in the CT reports. Then, we generate the prompts by combining the prompt template with the CT reports as the inputs of ChatGPT to obtain the responses. A post-processing module is developed to transform the responses into structured extraction results. Besides, we add prior medical knowledge to the prompt template to reduce wrong extraction results. We also explore the consistency of the extraction results. Results: We conducted the experiments with 847 real CT reports. The experimental results indicate that ChatGPT can achieve competitive performances for some extraction tasks like tumor location, tumor long and short diameters compared with the baseline information extraction system. By adding some prior medical knowledge to the prompt template, extraction tasks about tumor spiculations and lobulations obtain significant improvements but tasks about tumor density and lymph node status do not achieve better performances. Conclusion: ChatGPT can achieve competitive information extraction for radiological reports in a zero-shot manner. Adding prior medical knowledge as instructions can further improve performances for some extraction tasks but may lead to worse performances for some complex extraction tasks.
更多查看译文
关键词
Information extraction,Large language model,Question answering,Radiological report,Lung cancer
AI 理解论文
溯源树
样例
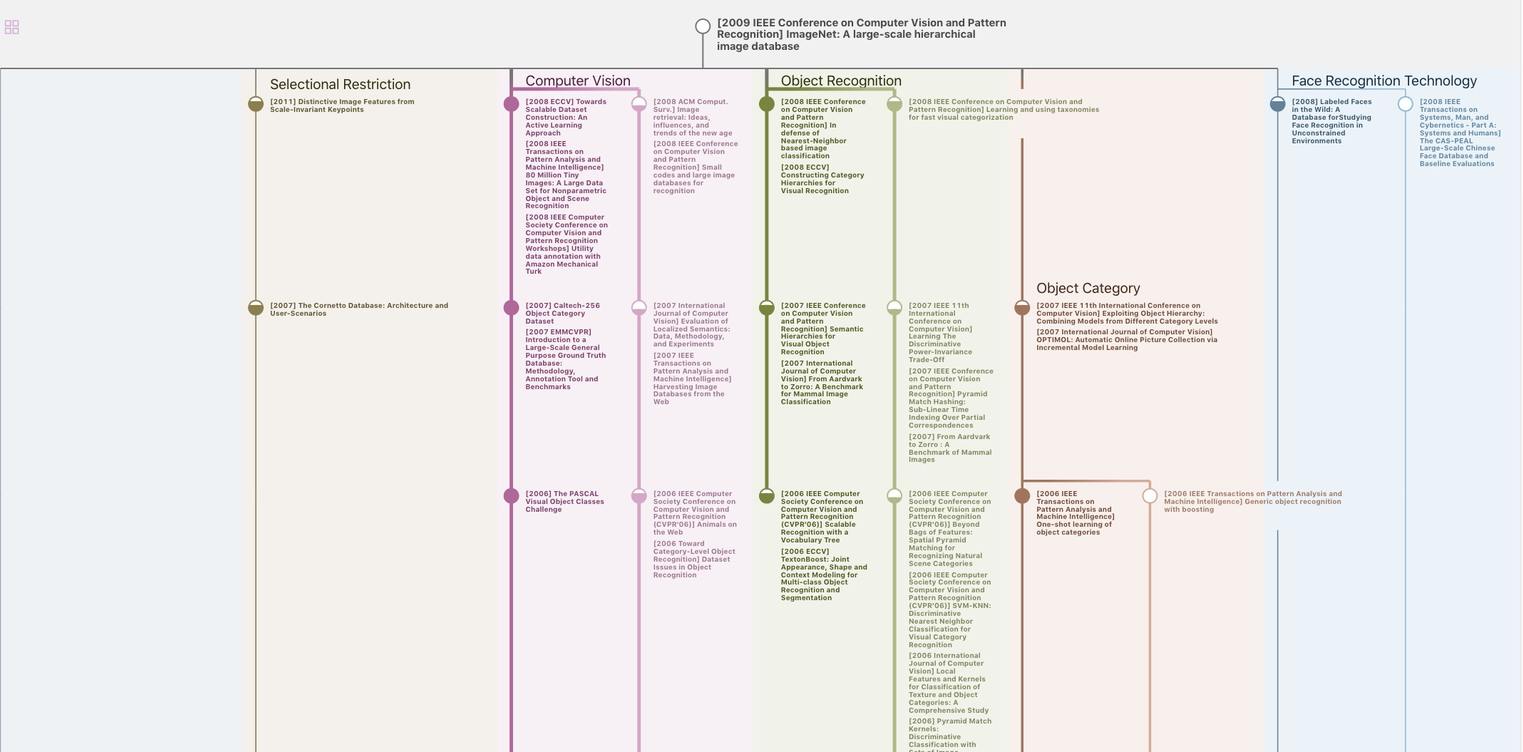
生成溯源树,研究论文发展脉络
Chat Paper
正在生成论文摘要