Simple Yet Effective Joint Guidance Learning for Few-Shot Semantic Segmentation
Applied intelligence(2023)
摘要
Fully-supervised semantic segmentation methods are difficult to generalize to novel objects, and their fine-tuning often requires a sufficient number of fully-labeled images. Few-shot semantic segmentation (FSS) has recently attracted lots of attention due to its excellent capability for segmenting the novel object with only a few labeled images. Most of recent approaches follow the prototype learning paradigm and have made a significant improvement in segmentation performance. However, there exist two critical bottleneck problems to be solved. (1) Previous methods mainly focus on mining the foreground information of the target object, and class-specific prototypes are generated by solely leveraging average operation on the whole support image, which may lead to information loss, underutilization, or semantic confusion of the object. (2) Most existing methods unilaterally guide the object segmentation in the query image with support images, which may result in semantic misalignment due to the diversity of objects in the support and query sets. To alleviate the above challenging problems, we propose a simple yet effective joint guidance learning architecture to generate and align more compact and robust prototypes from two aspects. (1) We propose a coarse-to-fine prototype generation module to generate coarse-grained foreground prototypes and fine-grained background prototypes. (2) We design a joint guidance learning module for the prototype evaluation and optimization on both support and query images. Extensive experiments show that the proposed method can achieve superior segmentation results on PASCAL-5 ^i and COCO-20 ^i datasets.
更多查看译文
关键词
Few-shot semantic segmentation,Joint guidance learning,Coarse-to-fine prototype generation,Prototype learning
AI 理解论文
溯源树
样例
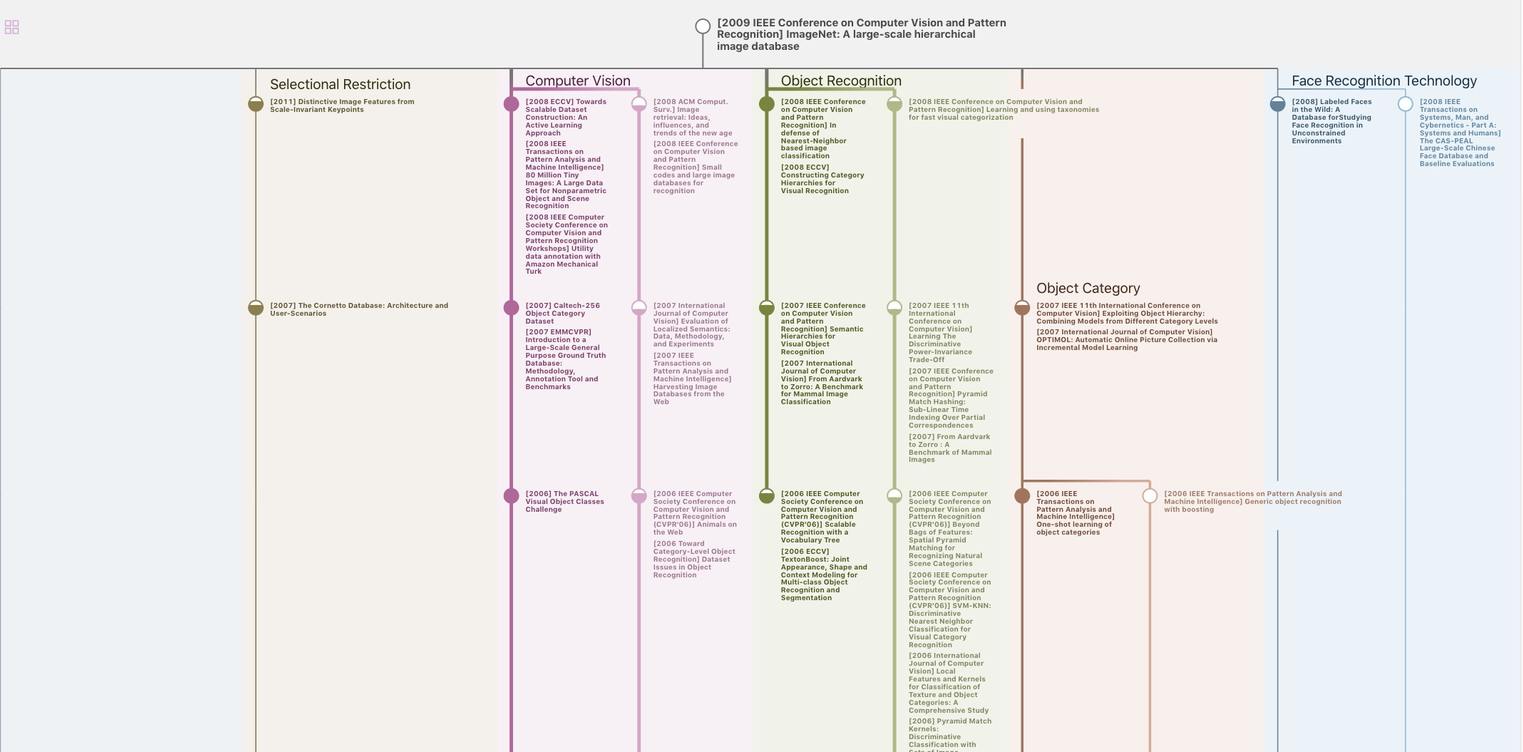
生成溯源树,研究论文发展脉络
Chat Paper
正在生成论文摘要