A Hybrid Neuro-Fuzzy Approach for Heterogeneous Patch Encoding in ViTs Using Contrastive Embeddings and Deep Knowledge Dispersion.
IEEE access(2023)
摘要
Vision Transformers (ViT) are commonly utilized in image recognition and related applications. It delivers impressive results when it is pre-trained using massive volumes of data and then employed in mid-sized or small-scale image recognition evaluations such as ImageNet and CIFAR-100. Basically, it converts images into patches, and then the patch encoding is used to produce latent embeddings (linear projection and positional embedding). In this work, the patch encoding module is modified to produce heterogeneous embedding by using new types of weighted encoding. A traditional transformer uses two embeddings including linear projection and positional embedding. The proposed model replaces this with weighted combination of linear projection embedding, positional embedding and three additional embeddings called Spatial Gated, Fourier Token Mixing and Multi-layer perceptron Mixture embedding. Secondly, a Divergent Knowledge Dispersion (DKD) mechanism is proposed to propagate the previous latent information far in the transformer network. It ensures the latent knowledge to be used in multi headed attention for efficient patch encoding. Four benchmark datasets (MNIST, Fashion-MNIST, CIFAR-10 and CIFAR-100) are used for comparative performance evaluation. The proposed model is named as SWEKP-based ViT, where the term SWEKP stands for Stochastic Weighted Composition of Contrastive Embeddings & Divergent Knowledge Dispersion (DKD) for Heterogeneous Patch Encoding. The experimental results show that adding extra embeddings in transformer and integrating DKD mechanism increases performance for benchmark datasets. The ViT has been trained separately with combination of these embeddings for encoding. Conclusively, the spatial gated embedding with default embeddings outperforms Fourier Token Mixing and MLP-Mixture embeddings.
更多查看译文
关键词
Vision transformer,patch encoding,spatial gated unit,Fourier token mixing,MLP-mixture embedding,computer vision
AI 理解论文
溯源树
样例
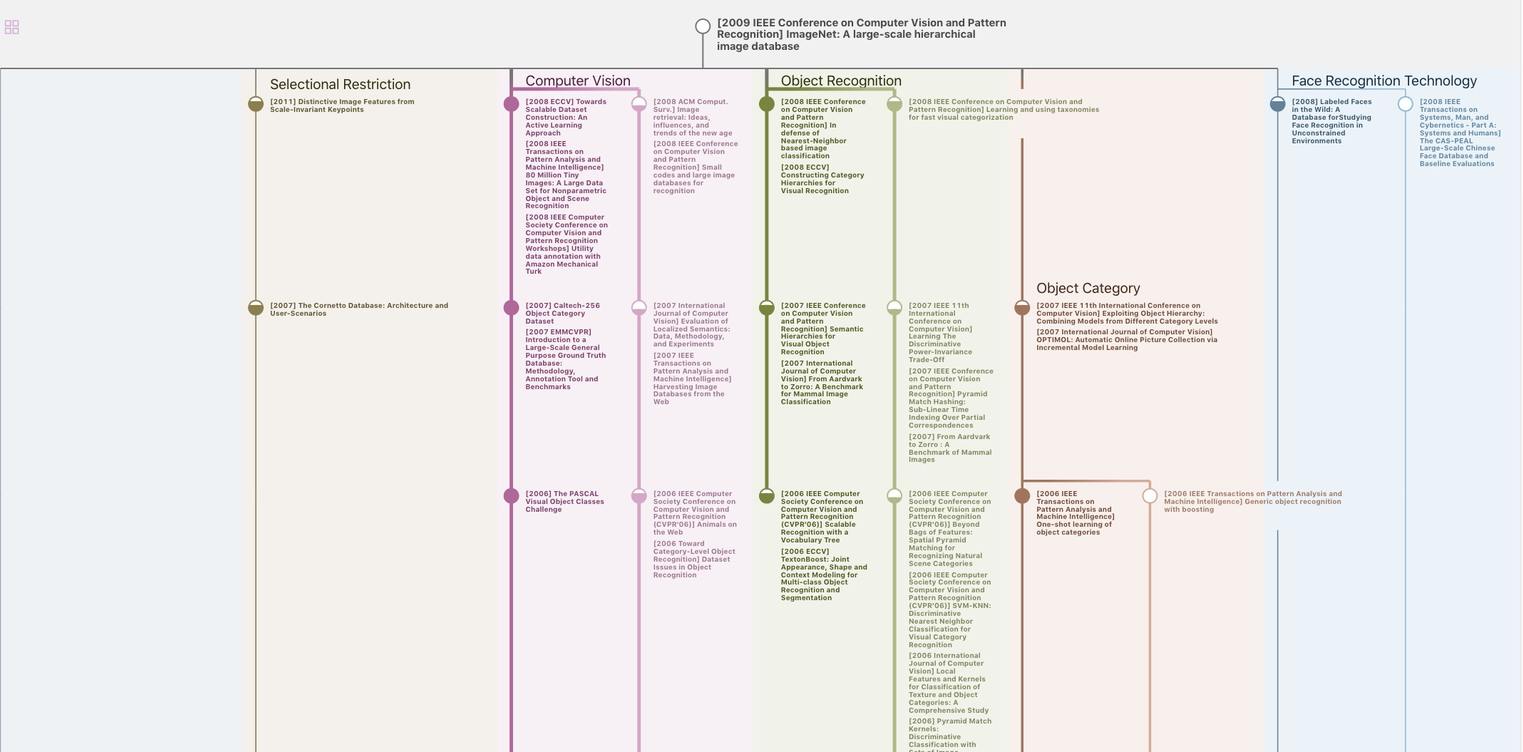
生成溯源树,研究论文发展脉络
Chat Paper
正在生成论文摘要