EMO2-DETR: Efficient-Matching Oriented Object Detection With Transformers.
IEEE Trans. Geosci. Remote. Sens.(2023)
摘要
Object detection in remote sensing is a challenging task due to the arbitrary orientations of objects and the vast variation in the number of objects within a single image. For instance, one image may contain hundreds of small vehicles, while another may only have a single football field. Recently, DEtection TRansformer (DETR) and its variants have achieved great success in object detection by setting a fixed number of object queries and using bipartite graph matching for one-to-one label assignment. However, we have observed that bipartite graph matching can result in relative redundancy of object queries when the number of objects changes dramatically in an image. This relative redundancy can cause two problems: slower convergence during training and redundant bounding boxes during inference. To analyze the aforementioned problems, we proposed a metric, redundancy of object query (ROQ), to quantitatively analyze the redundancy. Through experiments, we discovered that the reason for the two issues is the difficulty in distinguishing between high-quality negative samples and positive samples. In this article, we proposed efficient-matching oriented object detection with transformers (EMO2-DETR) consisting of three dedicated components to address the aforementioned issues. Specifically, reassign bipartite graph matching (RBGM) is proposed to extract high-quality negative samples from the negative samples. And ignored sample predicted head (ISPH) is proposed to predict high-quality negative samples. Then, reassigned Hungarian loss is used to better involve high-quality negative samples in the update of model parameters. Extensive experiments on DOTAv1 and DOTAv1.5 datasets demonstrated that our proposed method achieves the competitive results.
更多查看译文
关键词
Detection transformer (DETR), high-quality negative samples, object detection, relative redundancy of object query, remote sensing
AI 理解论文
溯源树
样例
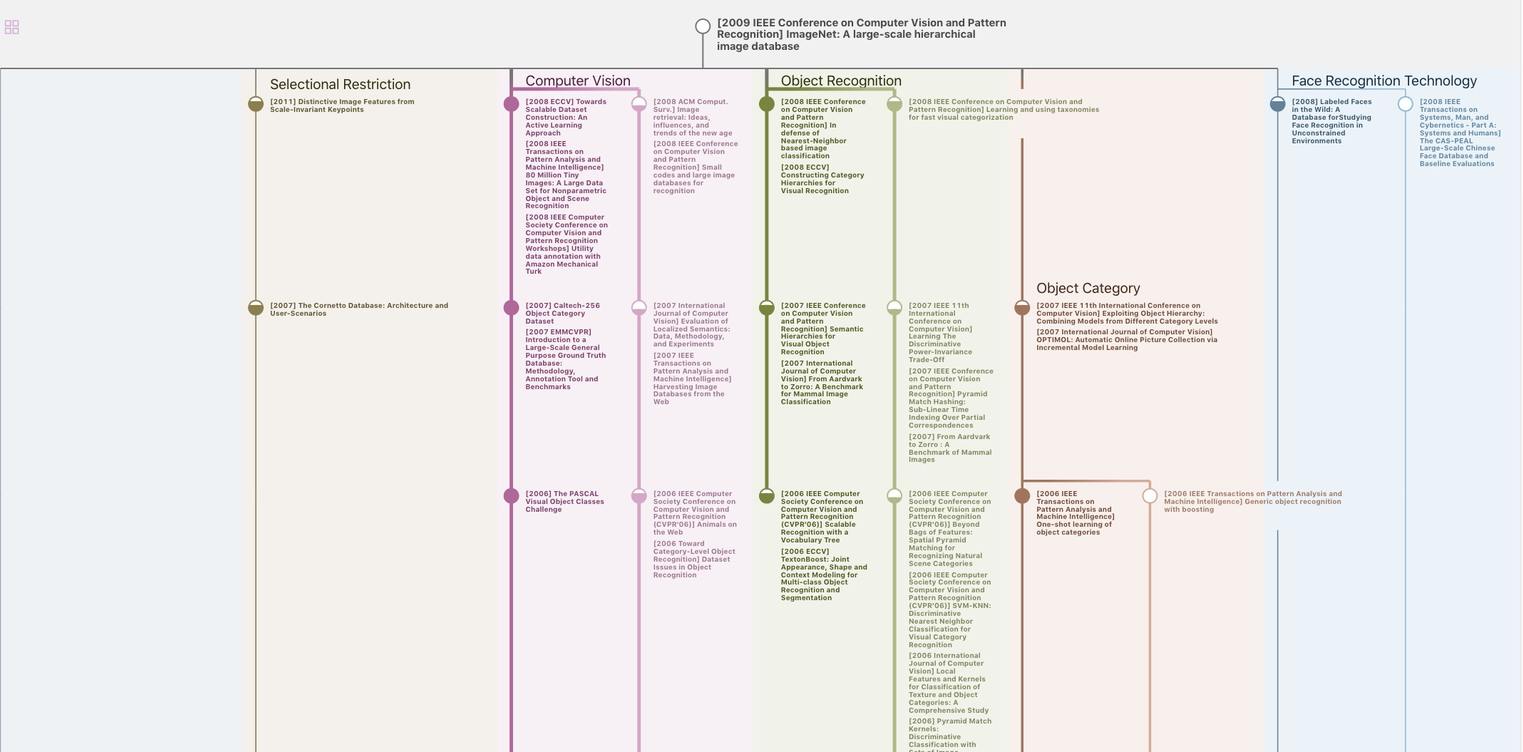
生成溯源树,研究论文发展脉络
Chat Paper
正在生成论文摘要