TDRConv: Exploring the Trade-off Between Feature Diversity and Redundancy for a Compact CNN Module.
ICIC (4)(2023)
Abstract
Rich or even redundant features without losing diversity of feature maps can undoubtedly help to improve network performance. In this work, we propose a compact CNN module by exploring the trade-off between feature diversity and redundancy, namely TDRConv, to retain and generate features with moderate redundancy and rich diversity but require less computation. Specifically, the input features are split into the main part and the expansion part by certain proportion, where the main part can extract intrinsic and diverse features in different ways, while the expansion part can enhance the extraction ability of diverse information. Finally, a series of experiments are conducted to verify the effectiveness of the proposed TDRConv on CIFAR10 and ImageNet. The results show the network models equipped with TDRConv can all outperform the state-of-the-art methods in terms of accuracy, but with significantly lower FLOPs and parameters. More importantly, the proposed TDRConv can readily replace the existing convolution modules as a plug-and-play component, and it is promising to further extend CNNs to wider scenarios.
MoreTranslated text
Key words
compact cnn module,feature diversity,redundancy,trade-off
AI Read Science
Must-Reading Tree
Example
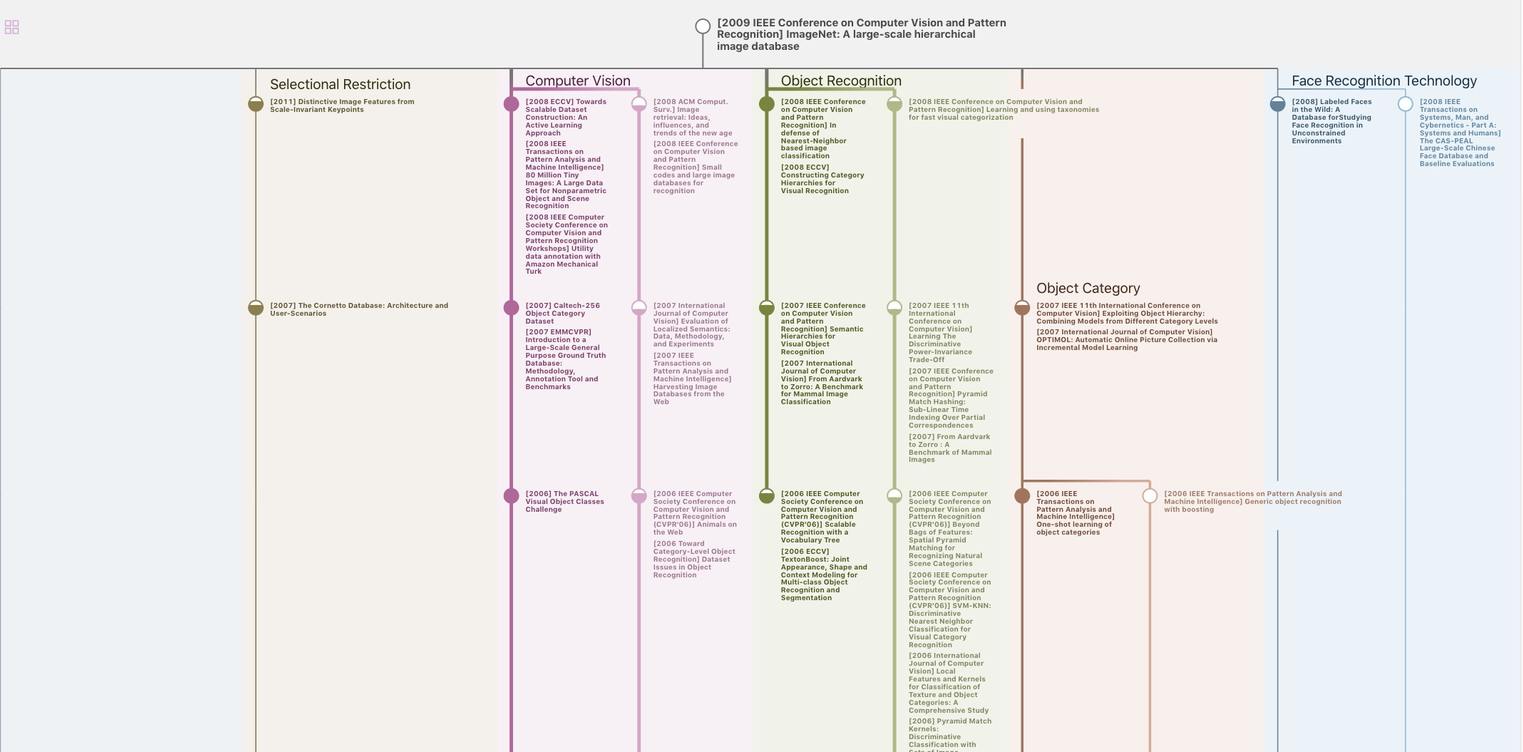
Generate MRT to find the research sequence of this paper
Chat Paper
Summary is being generated by the instructions you defined