TPatch: A Triggered Physical Adversarial Patch
PROCEEDINGS OF THE 32ND USENIX SECURITY SYMPOSIUM(2023)
摘要
Autonomous vehicles increasingly utilize the vision-based perception module to acquire information about driving environments and detect obstacles. Correct detection and classification are important to ensure safe driving decisions. Existing works have demonstrated the feasibility of fooling the perception models such as object detectors and image classifiers with printed adversarial patches. However, most of them are indiscriminately offensive to every passing autonomous vehicle. In this paper, we propose TPatch, a physical adversarial patch triggered by acoustic signals. Unlike other adversarial patches, TPatch remains benign under normal circumstances but can be triggered to launch a hiding, creating or altering attack by a designed distortion introduced by signal injection attacks towards cameras. To avoid the suspicion of human drivers and make the attack practical and robust in the real world, we propose a content-based camouflage method and an attack robustness enhancement method to strengthen it. Evaluations with three object detectors, YOLO V3/V5 and Faster R-CNN, and eight image classifiers demonstrate the effectiveness of TPatch in both the simulation and the real world. We also discuss possible defenses at the sensor, algorithm, and system levels.
更多查看译文
AI 理解论文
溯源树
样例
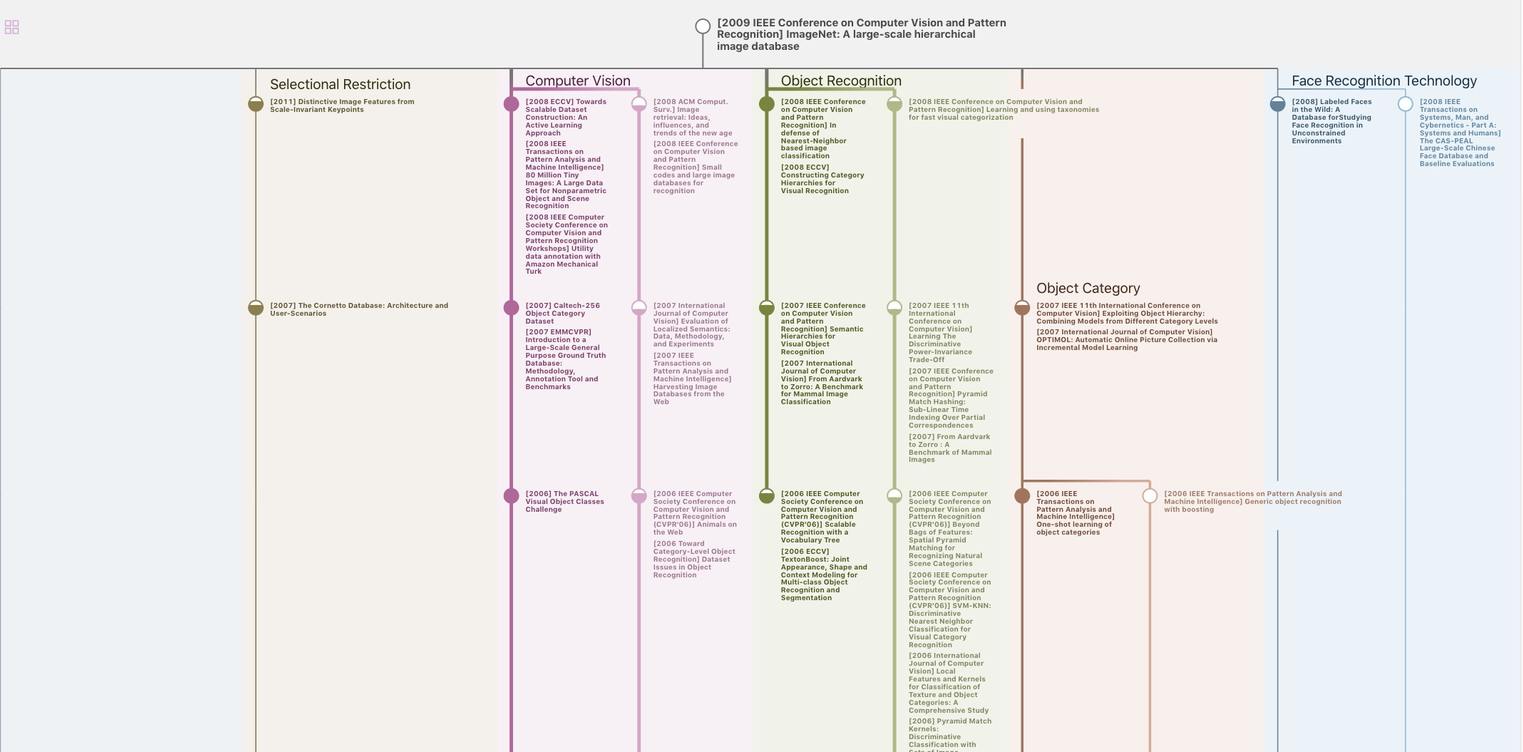
生成溯源树,研究论文发展脉络
Chat Paper
正在生成论文摘要