A Current-Mode Implementation of A Nearest Neighbor STDP Synapse
NEWCAS(2023)
Abstract
The Artificial Intelligence (AI) disruption continues unabated, albeit at extreme compute requirements. Neuromorphic circuits and systems offer a panacea for this extravagance. To this effect, event-based learning such as spike-timing-dependent plasticity (STDP) in spiking neural networks (SNNs) is an active area of research. Hebbian learning in SNNs fundamentally involves synaptic weight updates based on temporal correlations between pre- and post- synaptic neural activities. While there are broadly two approaches of realizing STDP, i.e. All-to-All versus Nearest Neighbor (NN), there exist strong arguments favoring the NN approach on the biologically plausibility front. In this paper, we present a novel current-mode implementation of a postsynaptic event-based NN STDP-based synapse. We leverage transistor subthreshold dynamics to generate exponential STDP traces using repurposed log-domain low-pass filter circuits. Synaptic weight operations involving addition and multiplications are achieved by the Kirchoff current law and the translinear principle respectively. Simulation results from the NCSU TSMC 180 nm technology are presented. Finally, the ideas presented here hold implications for engineering efficient hardware to meet the growing AI training and inference demands.
MoreTranslated text
Key words
analog computation,STDP,learning,local synaptic plasticity,three-factor,neuromorphic
AI Read Science
Must-Reading Tree
Example
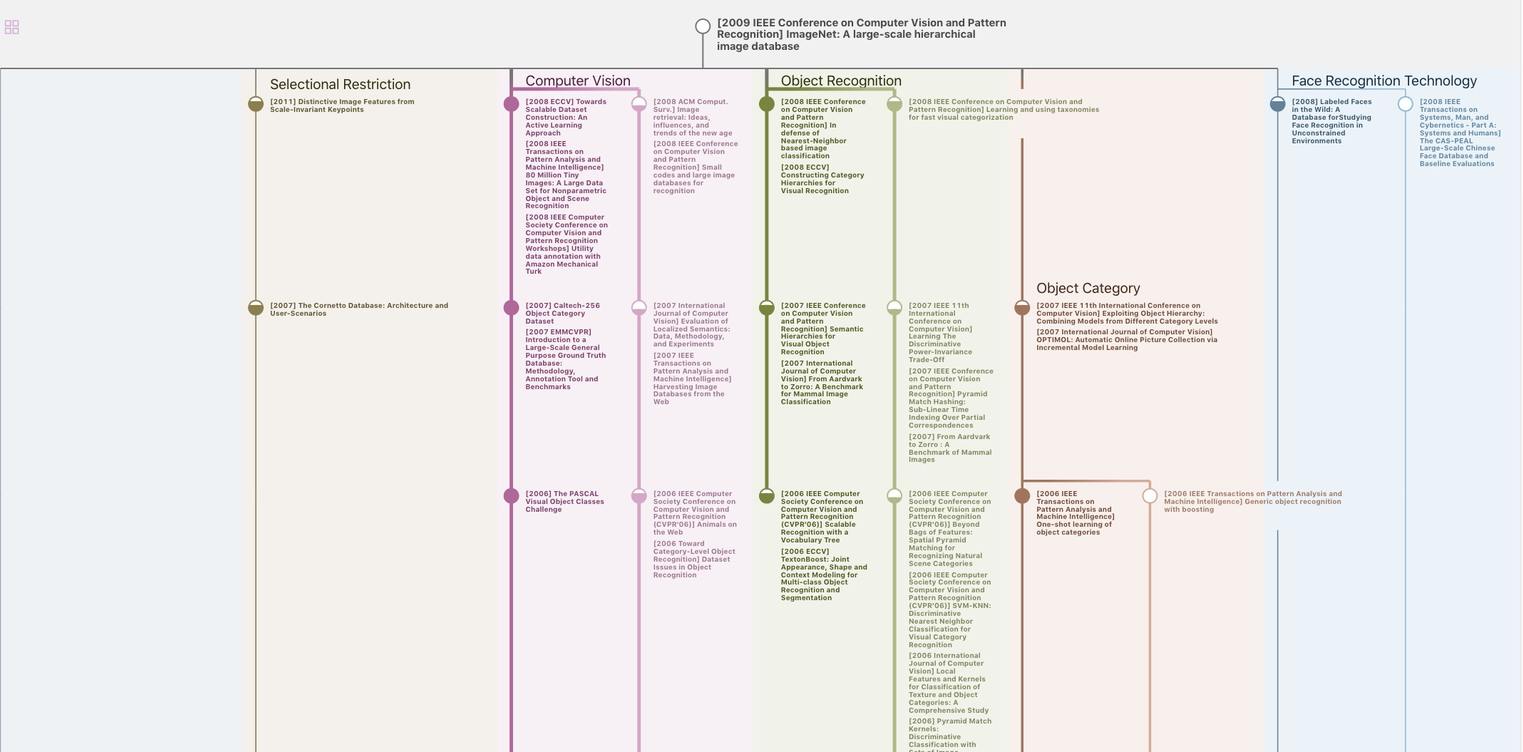
Generate MRT to find the research sequence of this paper
Chat Paper
Summary is being generated by the instructions you defined