Decoupling Visual-Semantic Features Learning with Dual Masked Autoencoder for Self-Supervised Scene Text Recognition.
ICDAR (2)(2023)
摘要
Self-supervised text recognition has attracted more and more attention since it provides an effective way to utilize unlabeled real text images. Nowadays, Masked Image Modeling (MIM) shows superiority in visual representation learning, and several works introduce it into text recognition. In this paper, we take a further step and design a method for text-recognition-friendly self-supervised feature learning. Specifically, we propose to decouple visual and semantic feature learning with different masking strategies. For the visual features, intra-window random masking is proposed where the reconstruction is applied on a local image region with random masking, which prevents the model from the help of much context information. In the meanwhile, semantic feature learning is based on a window random masking, which removes more visual clues and boosts the sequence modeling of the model. Based on this idea, we first propose a siamese network that aligns dual features with each other, then we explore the dual distillation with a co-teacher framework. Our proposed method shows the effectiveness of self-supervised scene text recognition with state-of-the-art performances on most benchmarks.
更多查看译文
关键词
dual masked autoencoder,recognition,text,visual-semantic,self-supervised
AI 理解论文
溯源树
样例
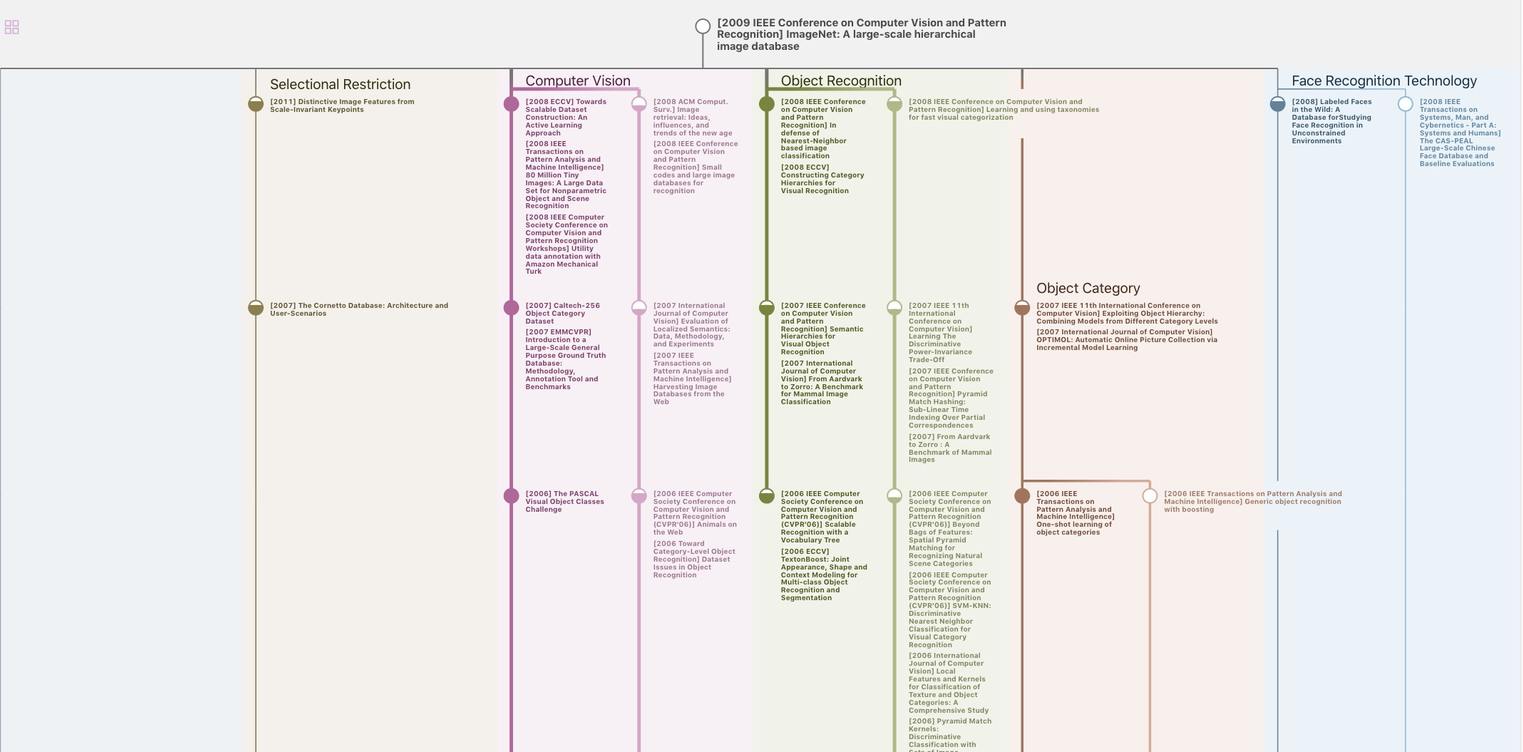
生成溯源树,研究论文发展脉络
Chat Paper
正在生成论文摘要