Deep Learning-based Demodulator for Magnitude Modulated Signals
IEEE Vehicular Technology Conference(2023)
摘要
The current applications’ requirement for higher data rates typically results in an increase in the signal’s Peak-to-Average Power Ratio (PAPR), which harms the transmitter’s Power Amplifier (PA) efficiency. The Magnitude Modulation (MM) technique was developed to address this issue in bandlimited single-carrier (SC) communications, leaving a trade-off between the attainable PAPR reduction and an increase in the bit error rate (BER) upon MM signal demodulation. Because Deep Learning (DL) algorithms are proving to be applicable in the majority of fields, two models based on current state-of-the-art Artificial Neural Networks (ANNs) are proposed in this paper to replace conventional demodulators. It is also shown that DL demodulators can leverage on the symbols’ memory that is embedded within the transmitted MM signal, caused by the MM procedure. Both systems’ BER performance is analyzed and compared, demonstrating that it is possible to mitigate the MM effect on demodulation.
更多查看译文
关键词
Deep Learning,Neural Networks,Magnitude Modulation,Demodulator
AI 理解论文
溯源树
样例
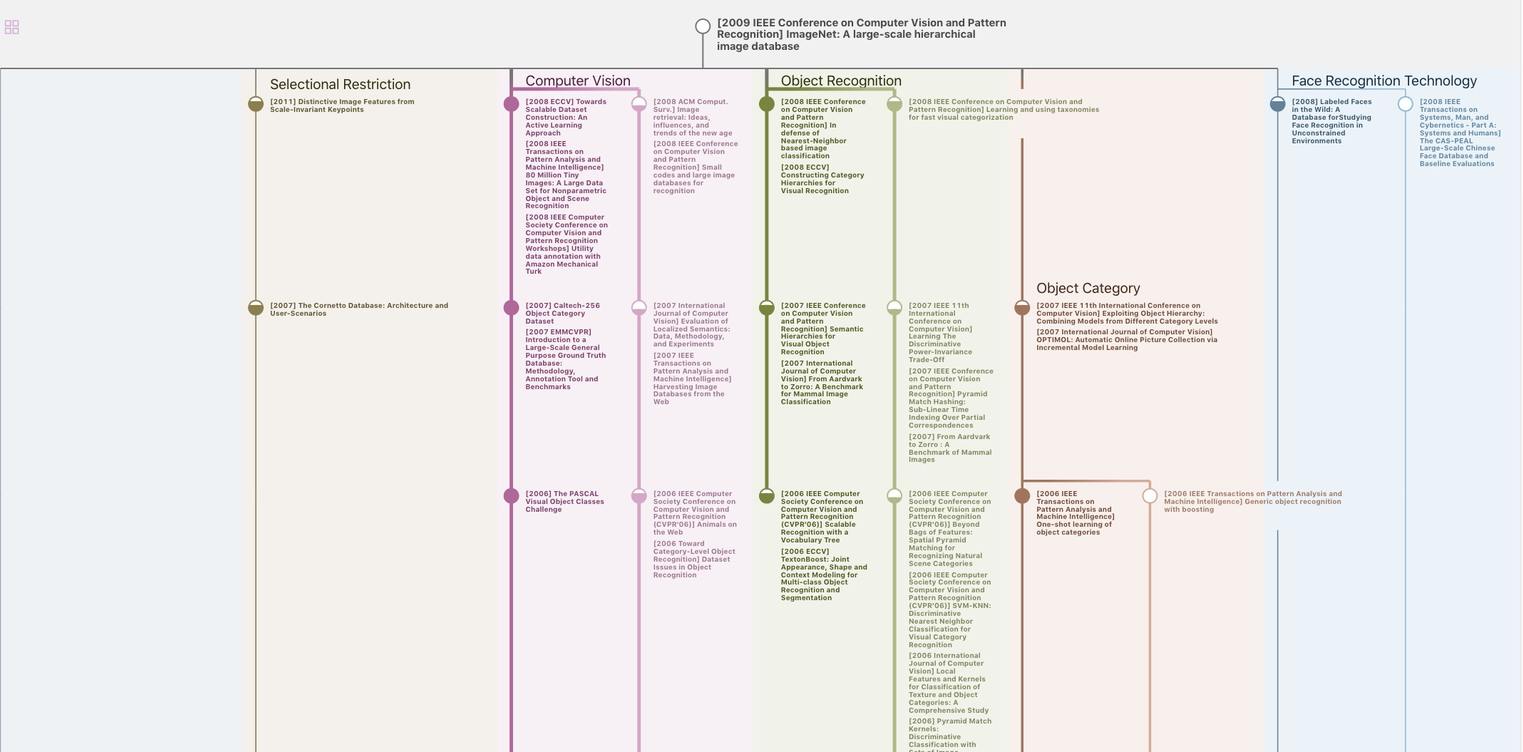
生成溯源树,研究论文发展脉络
Chat Paper
正在生成论文摘要