Multiple Surgical Instruments Tracking-By-Prediction with Graph Hierarchy.
2023 IEEE International Conference on Robotics and Automation (ICRA)(2023)
摘要
Current research strive has tremendously changed the horizon of computer vision tasks in multiple agents tracking. Nevertheless, in the research of robotic assisted surgery, reliable surgical instrument tracking imposes challenge due to the high complexity in state modeling for the hierarchical structure of the instrument versus de-coupling the spatial-temporal correlations naturally embedded in the task. In this paper, we present a new tracking paradigm integrating the trajectory prediction to reduce the data association error that is propagated from the false detection. As a key component in the system, a proposed predictor disentangles the hierarchical modeling and agent kinematic learning by introducing inductive attention mechanism in spatial-temporal graph network. Experiments on real anatomical datasets show that our tracking-by-prediction scheme improves overall localization accuracy over the frames by up to 81%, in comparison to the generic pipelines of tracking, even with transductive graph representation learning, with a large margin of gain in terms of precise localization.
更多查看译文
关键词
agent kinematic learning,computer vision,data association error,graph hierarchy,hierarchical structure,multiple surgical instruments tracking-by-prediction,robotic assisted surgery,spatial-temporal correlations,spatial-temporal graph network,state modeling,tracking paradigm,trajectory prediction,transductive graph representation learning
AI 理解论文
溯源树
样例
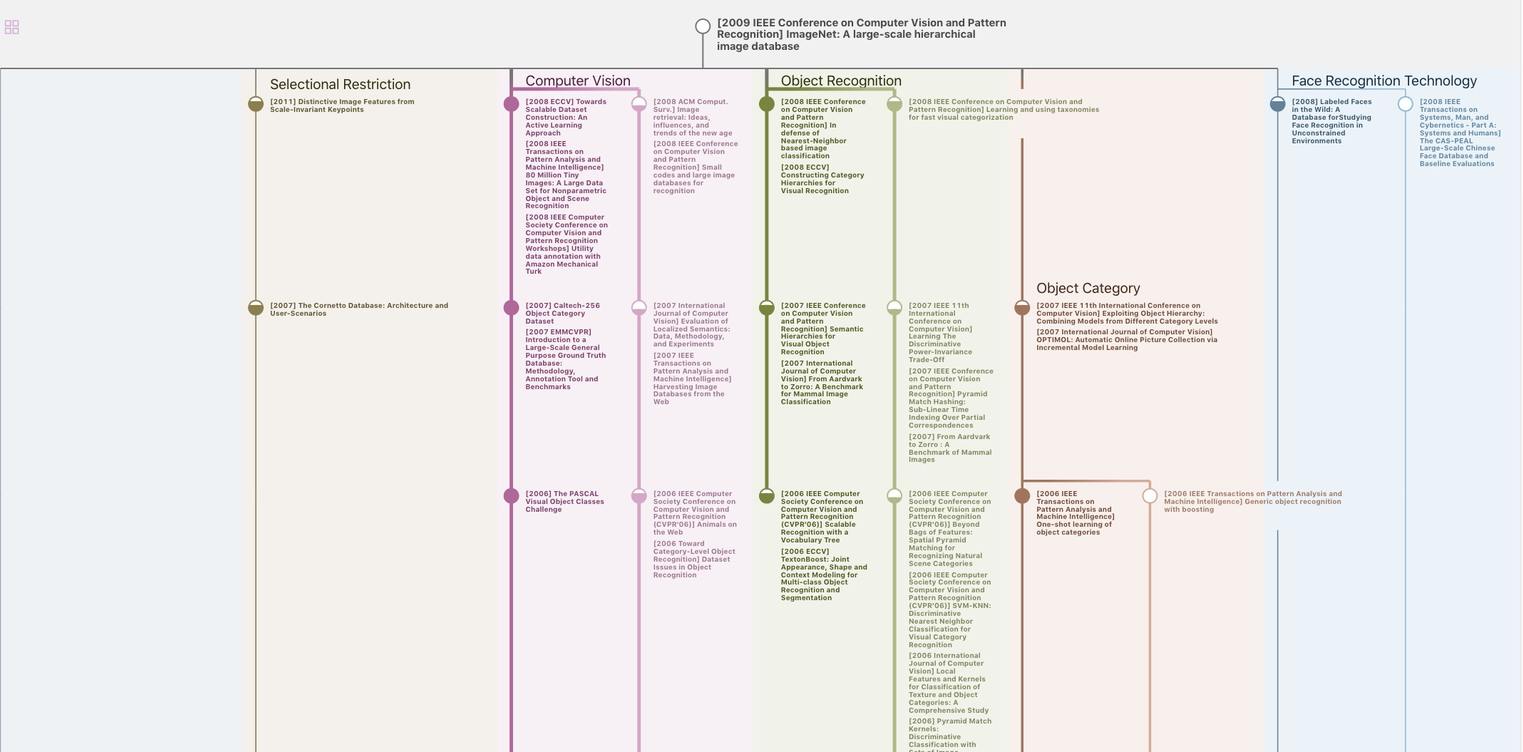
生成溯源树,研究论文发展脉络
Chat Paper
正在生成论文摘要