Boosting Aspect Sentiment Quad Prediction by Data Augmentation and Self-Training
IJCNN(2023)
摘要
Aspect Sentiment Quad Prediction (ASQP), which aims to predict the (aspect category, aspect term, opinion term, sentiment polarity) quadruple in a sentence, is an important subtask of Aspect-based Sentiment Analysis (ABSA) and has also gained much research attention. Recently, a number of works adopt generative models to do ASQP and achieve promising performance. Nevertheless, these approaches are limited by data scarcity, due to the challenge of labeling fine-grained quadruple annotations. Even data augmentation techniques do not help much because it is difficult to maintain the quality of augmented samples. Furthermore, the proposed templates of generative models are undesirable in extracting semantic and structural information, thus cannot fully exploit the knowledge of the language models. In this paper, to boost the performance of ASQP, we propose a novel method called DAST (the abbreviation of Data Augmentation and Self-Training). Specifically, DAST first generates large amounts of unlabeled data from the original training data. Then, an iterative self-training mechanism is proposed to fully utilize the unlabeled data to train the ASQP model. In each iteration, we employ a base ASQP model and a discriminator to obtain high-quality samples with pseudo labels, and further enhance the two models with the augmented data. In our method, both the potential of data augmentation and the ASQP model are fully explored thanks to the iterative mechanism. Finally, we advance the ASQP base model via a novel tree-like template that improves the representations of the relations between different sentiment elements, and a double-check mechanism that leverages multi-task learning to detect incorrect predictions. Extensive experiments on two benchmark datasets demonstrate the superiority of our method over the existing ones.
更多查看译文
关键词
Aspect-based sentiment analysis, Aspect sentiment quad prediction, Data augmentation, Self-training
AI 理解论文
溯源树
样例
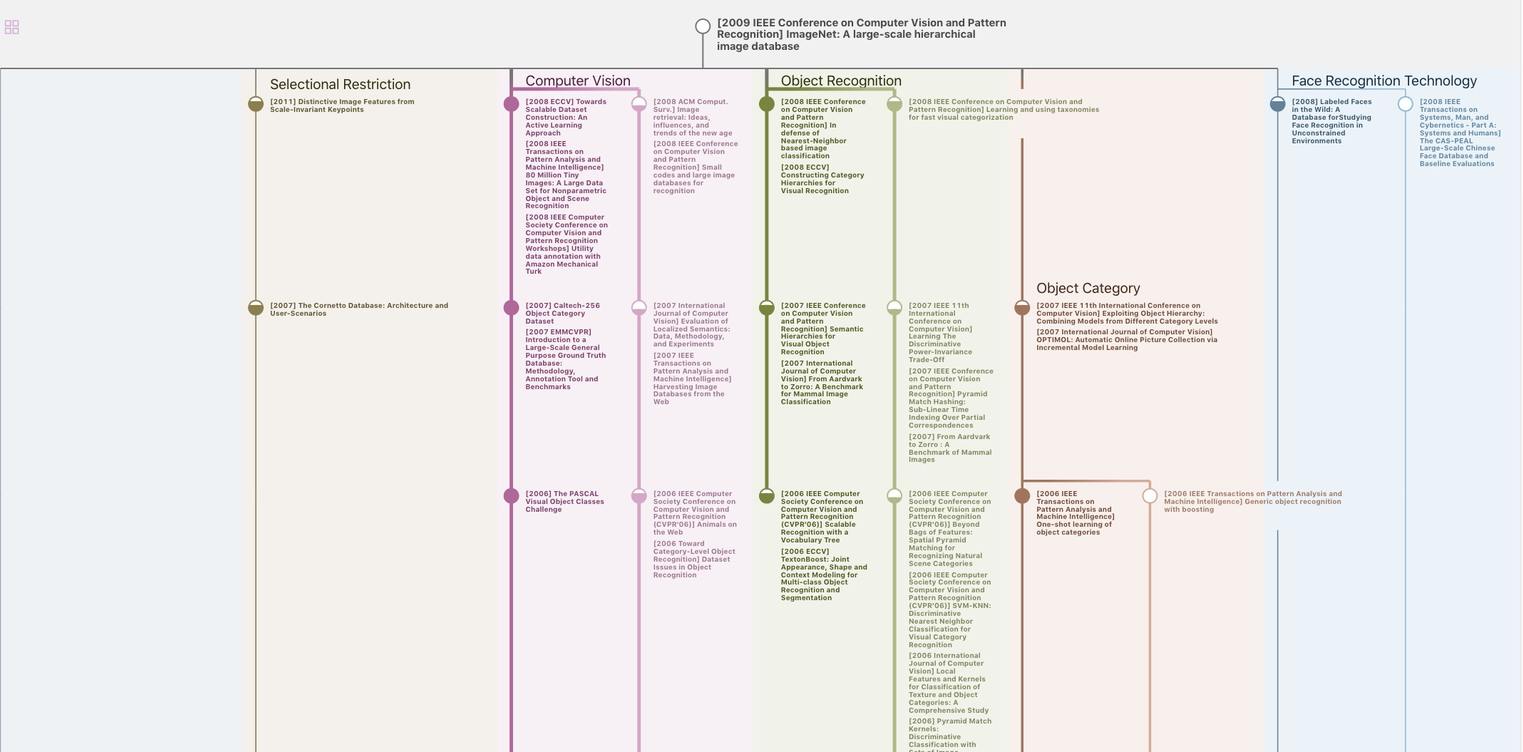
生成溯源树,研究论文发展脉络
Chat Paper
正在生成论文摘要