ConsE: Consistency Exploitation for Semi-Supervised Anomaly Detection in Graphs
IJCNN(2023)
摘要
Graph anomaly detection has attracted considerable interest due to the wide use of graph structure data. Several GNN-based anomaly detection methods discover anomalies through the powerful node representation ability of GNNs. However, real-world graphs are typically rarely labeled, which leads these deep learning methods to face the challenge of under-fitting. A fundamental question here is: can anomalies in graphs be detected with few annotations? In this paper, we propose a novel semi-supervised anomaly detection method in graphs based on Consistency Exploitation (ConsE). First, ConsE adopts a consistency-based neighbor sampler, which ensures the consistency of a central node and its neighbors on attributes and categories during the aggregation process through attribute similarity and soft pseudo-labels. Afterward, ConsE encourages the consistency of node representations generated by a dedicated neighbor sampler and a generic neighbor sampler to improve its robustness in complex neighborhoods. Experimental results on real-world datasets demonstrate that our model significantly outperforms several state-of-the-art baseline methods.
更多查看译文
关键词
Anomaly Detection, Semi-supervised Learning, Consistency Regularization
AI 理解论文
溯源树
样例
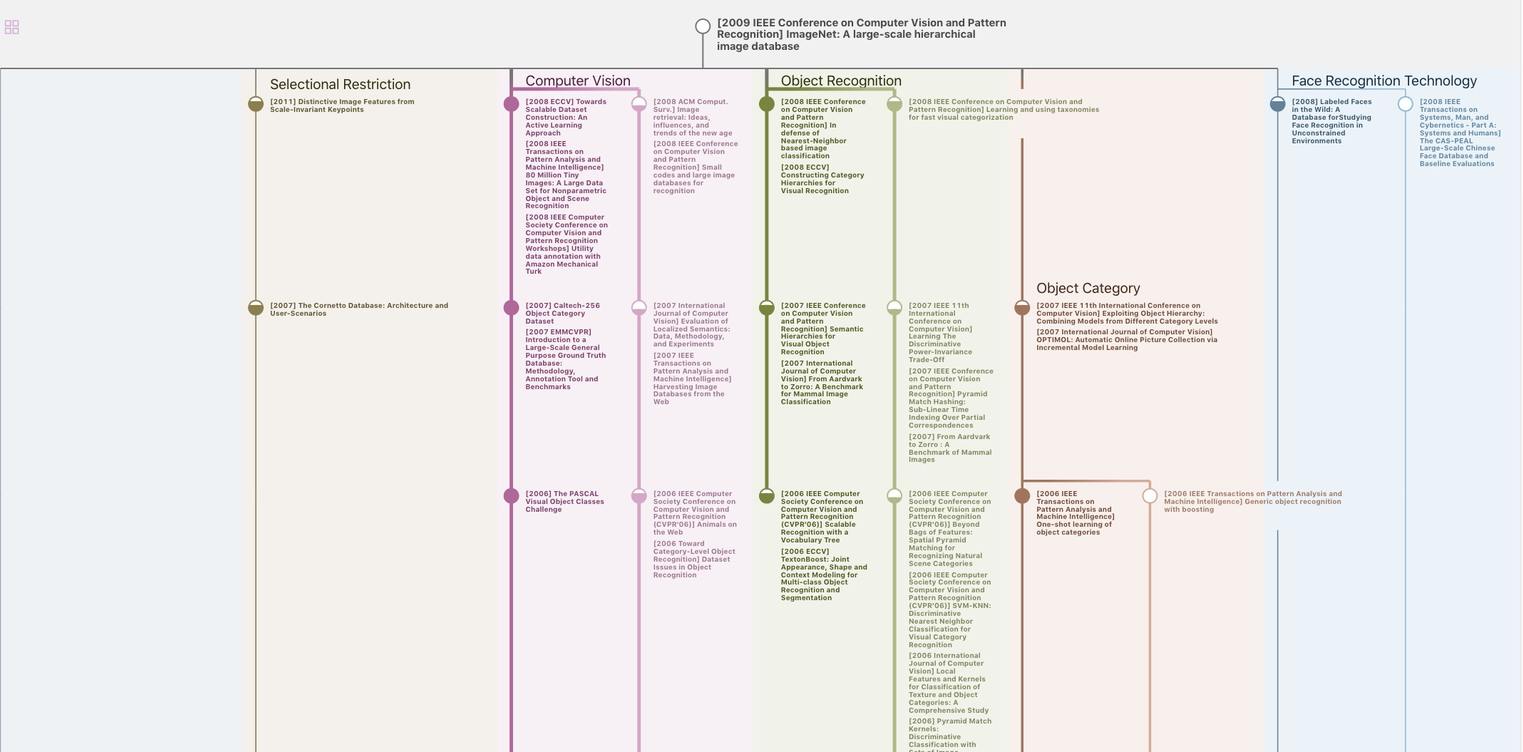
生成溯源树,研究论文发展脉络
Chat Paper
正在生成论文摘要