Adaptive Priority Reweighing for Generalizing Fairness Improvement.
IJCNN(2023)
摘要
With the increasing penetration of Machine-Learning (ML) applications in critical decision-making areas, calls for algorithmic fairness are more prominent. Though there have been diverse modalities to improve algorithmic fairness through training the algorithms with fairness constraints, their performance does not generalize well at the test set. A performance-promising fair algorithm with better generalizability is needed. This paper proposes a novel adaptive reweighing method to eliminate the impact of the distribution shifts between training and test data on model generalizability. Specifically, instead of assigning a unified weight for each (sub)group as most previous reweighing methods propose, we granularly model the distance from the sample predictions to the decision boundary and assign higher individual weight to the samples closer to the decision boundary in each (sub)group. Our adaptive reweighing method prioritizes the samples closer to the decision boundary and assigns a higher weight to improve the generalizability of fair classifiers. We design extensive experiments to evaluate the generalizability of our adaptive priority reweighing method for accuracy and fairness measures (i.e., equal opportunity, equalized odds, and demographic parity.) in tabular benchmarks across Adult, COMPAS, and IPUMS. We further highlight the performance of our method in improving the fairness of language and vision models. We believe that our method shows promising results in improving the fairness of any pre-trained models simply via fine-tuning.
更多查看译文
关键词
adaptive priority reweighing method,algorithmic fairness,COMPAS,critical decision-making areas,decision boundary,diverse modalities,fair classifiers,fairness constraints,fairness improvement generalization,IPUMS,machine-learning applications,model generalizability,performance-promising fair algorithm,sample predictions,tabular benchmarks,unified weight
AI 理解论文
溯源树
样例
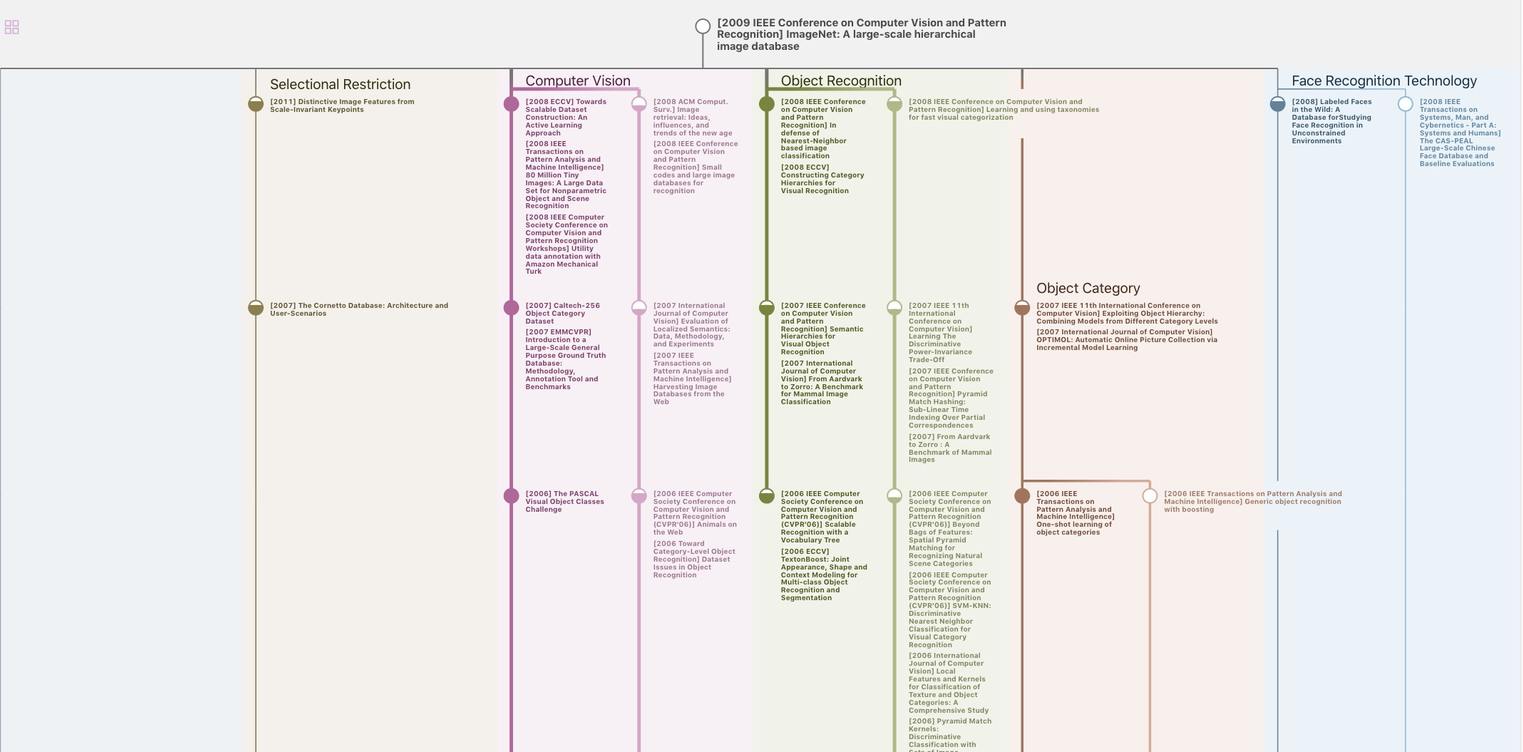
生成溯源树,研究论文发展脉络
Chat Paper
正在生成论文摘要