A Scalable GPT-2 Inference Hardware Architecture on FPGA
IJCNN(2023)
Abstract
Transformer-based architectures using attention mechanisms are a class of learning architectures for sequence processing tasks. These include architectures such as the generative pretrained transformer (GPT) and the bidirectional encoder representations from transformers (BERT). GPT-2 is a popular sequence learning architecture that uses transformer architecture. GPT-2 is trained on text prediction, and the network parameters obtained during this training process can be used in various other tasks like text classification and premise-hypothesis testing. Edge computing is an recent trend in which training is done on cloud or server with multiple GPUs, but inference is done on edge devices like mobile phones to reduce latency and improve privacy. This necessitates a study of GPT-2 performance and complexity to distill hardware-based architectures for their usability on edge devices. In this paper, a single layer of GPT-2 based inference architecture is implemented on Virtex-7 xc7vx485tffg1761-2 FPGA board. The inference engine has model dimensionality of 128 and latency of 1.637 ms while operating at 142.44 MHz, consuming 85.6K flip-flops and 96.8K lookup tables, achieving 1.73x speedup compared to previously reported work on transformer-based architecture. The approach proposed in this paper is scalable to models of higher dimensionality.
MoreTranslated text
Key words
GPT,transformer,neural networks,hardware architecture
AI Read Science
Must-Reading Tree
Example
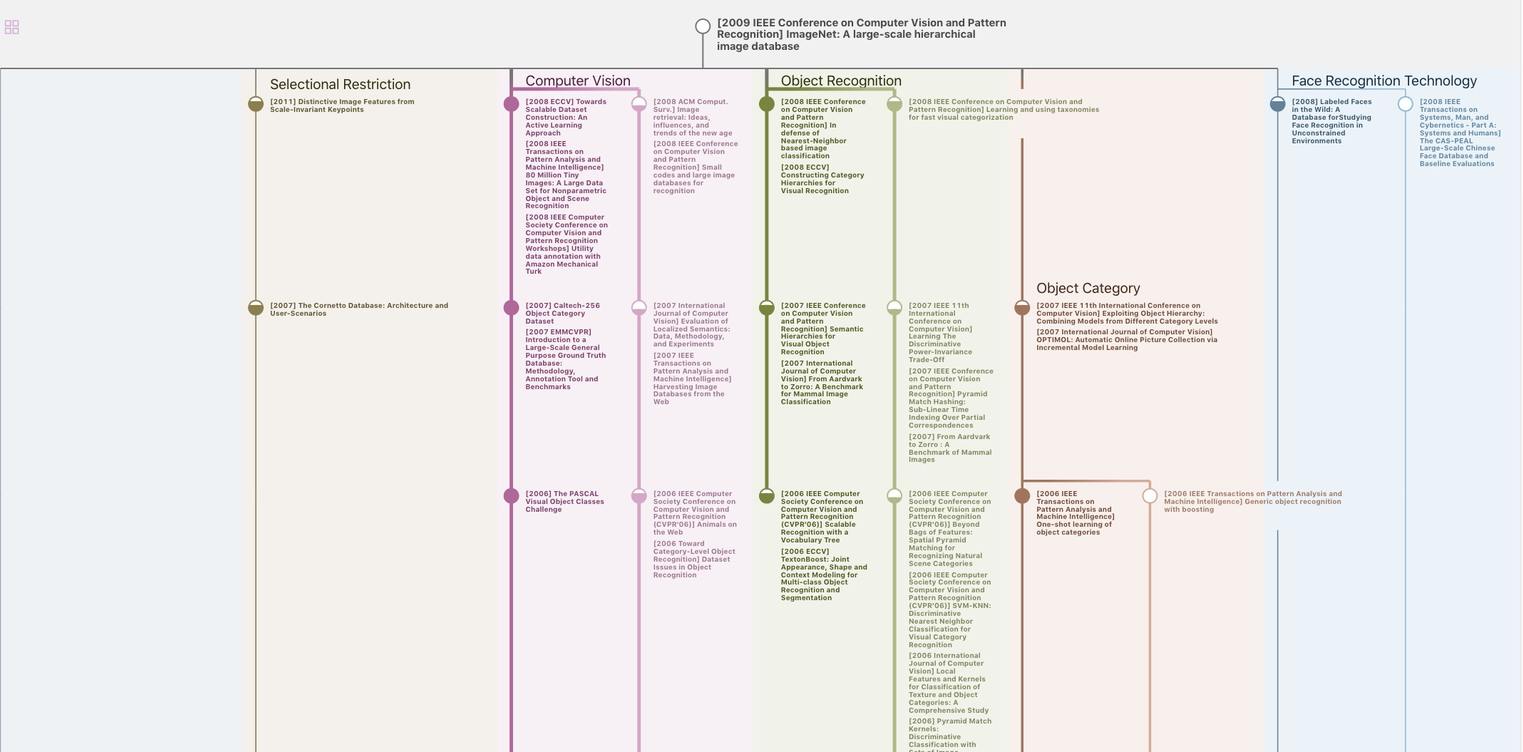
Generate MRT to find the research sequence of this paper
Chat Paper
Summary is being generated by the instructions you defined