Background Debiased SAR Target Recognition via Causal Interventional Regularizer
CoRR(2023)
摘要
Recent studies have utilized deep learning (DL) techniques to automatically extract features from synthetic aperture radar (SAR) images, which shows great promise for enhancing the performance of SAR automatic target recognition (ATR). However, our research reveals a previously overlooked issue: SAR images to be recognized include not only the foreground (i.e., the target), but also a certain size of the background area. When a DL-model is trained exclusively on foreground data, its recognition performance is significantly superior to a model trained on original data that includes both foreground and background. This suggests that the presence of background impedes the ability of the DL-model to learn additional semantic information about the target. To address this issue, we construct a structural causal model (SCM) that incorporates the background as a confounder. Based on the constructed SCM, we propose a causal intervention based regularization method to eliminate the negative impact of background on feature semantic learning and achieve background debiased SAR-ATR. The proposed causal interventional regularizer can be integrated into any existing DL-based SAR-ATR models to mitigate the impact of background interference on the feature extraction and recognition accuracy. Experimental results on the Moving and Stationary Target Acquisition and Recognition (MSTAR) dataset indicate that the proposed method can enhance the efficiency of existing DL-based methods in a plug-and-play manner.
更多查看译文
关键词
causal interventional
AI 理解论文
溯源树
样例
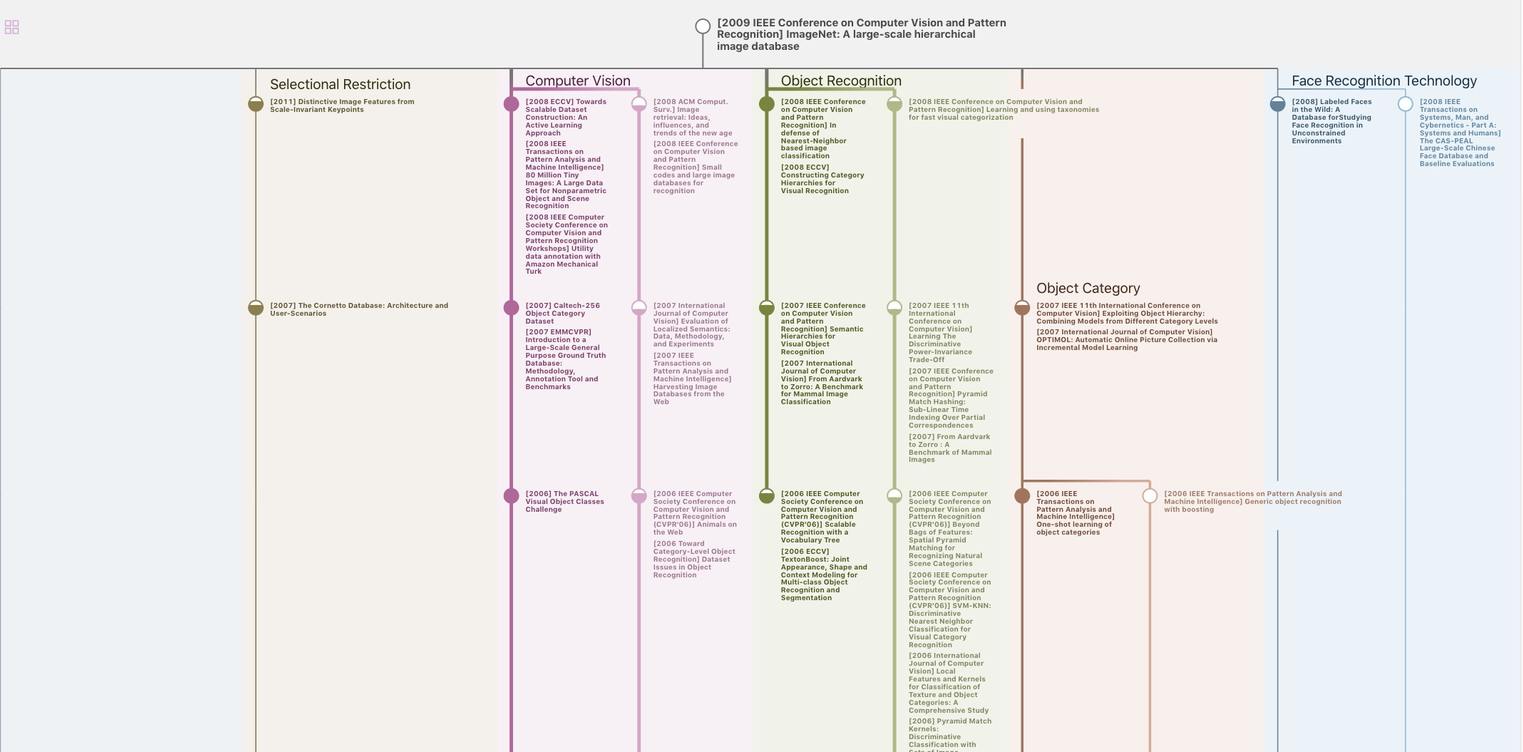
生成溯源树,研究论文发展脉络
Chat Paper
正在生成论文摘要