A decision-making tool for first-line treatment in advanced non-small-cell lung cancer based on plasma proteome biomarkers
JOURNAL OF CLINICAL ONCOLOGY(2023)
摘要
9122 Background: Initial treatment selection for advanced NSCLC patients without driver mutations is mainly based on evaluating PD-L1 expression levels in the tumor tissue. However, PD-L1 assays are only moderately predictive. In addition, the guidelines for the PD-L1≥50% subpopulation are not definitive, enabling the usage of immune checkpoint inhibitors (ICI) either as a monotherapy or combined with chemotherapy. Here we aim to provide a decision-making tool for the first-line treatment of advanced NSCLC patients based on plasma derived biomarkers obtained before treatment initiation. Methods: Pre-treatment plasma samples were collected from 545 NSCLC patients receiving ICI-based therapy. Clinical benefit was evaluated based on progression-free survival (PFS) at 12 months as a threshold. Deep plasma proteomic profiling was performed using aptamer technology. Based on the proteomic profiles, a computational model, termed PROphet, was developed and evaluated in a blinded manner on the validation subset (n = 272). The model output, clinical benefit probability, was used to stratify the patients into two groups, PROphet-positive or -negative. Results: The model displayed good performance with a high correlation between the predicted clinical benefit and the observed clinical benefit rate (R 2 = 0.97), outperforming a PD-L1-based prediction model (R 2 = 0.35). Patients classified as PROphet-positive achieved significantly longer overall survival (OS) than PROphet-negative patients, with a median OS of 25.9 versus 10.8 months (hazard ratio, HR = 0.51; 95% confidence interval, CI = 0.37-0.70; p-value < 0.001). Next, we examined the clinical utility of combining the PROphet output with PD-L1 levels by comparing different treatment modalities. Focusing on PD-L1≥50%, patients with PROphet-positive results displayed similar OS and PFS when receiving ICI monotherapy or ICI-chemotherapy (OS HR = 0.77; CI = 0.42-1.43; p-value = 0.4096), suggesting that these patients may consider monotherapy, thus avoiding chemotherapy-related toxicity. Conversely, PD-L1≥50% patients with a PROphet-negative result displayed a significantly longer OS and PFS when receiving ICI-chemotherapy in comparison to ICI monotherapy, with a median OS that was not reached versus 11.10 months in the combination therapy and monotherapy groups, respectively (HR = 0.29; CI = 0.14-0.59; p-value < 0.001). These patients should consider combination therapy, avoiding a treatment that is suboptimal for them. Results for PD-L1 1-49% and < 1% provide additional clinical information for each subgroup. Conclusions: Plasma proteomic profiling can provide biomarkers that accurately predict the benefit of ICI-based treatment. The model output complements tissue PD-L1 expression levels as a tool to assist therapeutic decisions.
更多查看译文
关键词
plasma proteome biomarkers,lung cancer,decision-making,first-line,small-cell
AI 理解论文
溯源树
样例
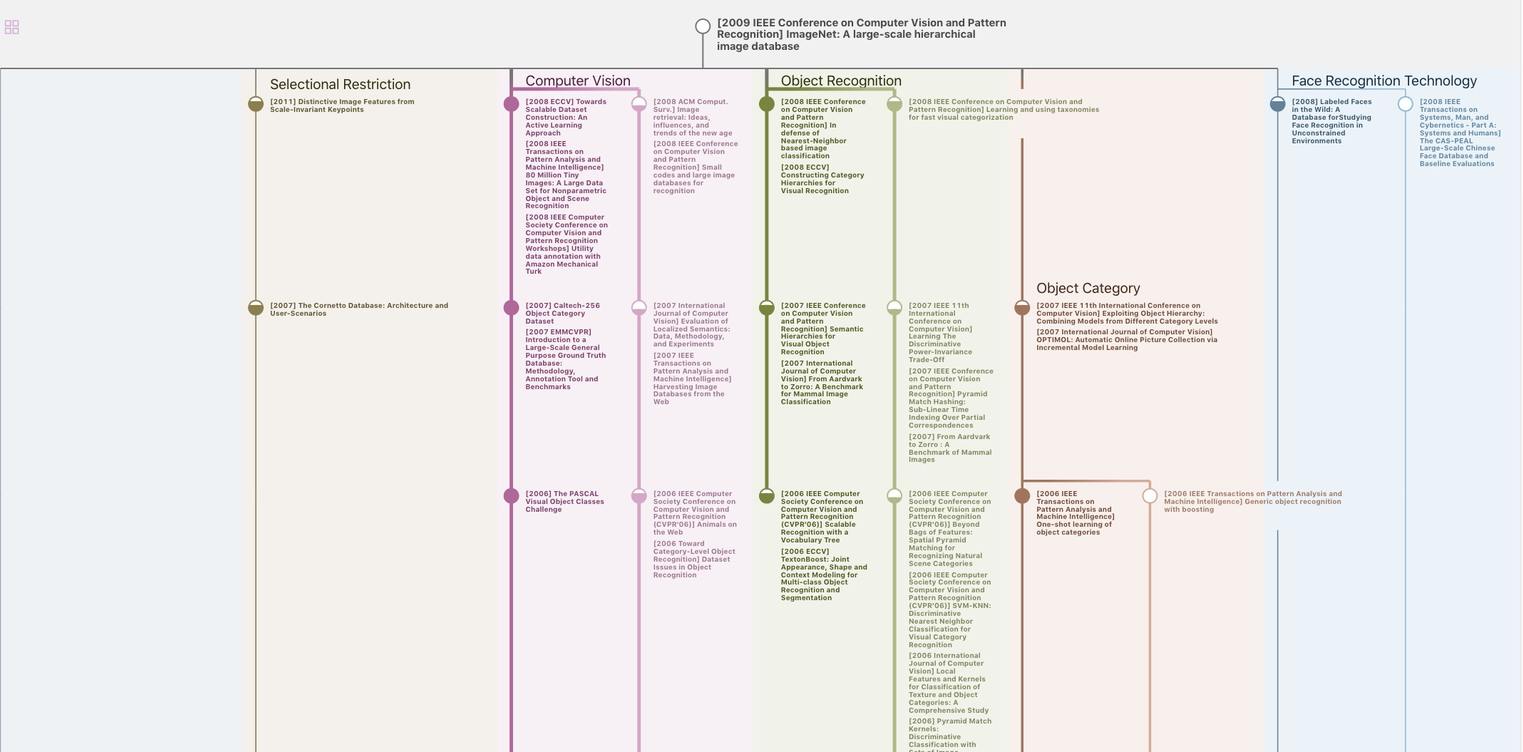
生成溯源树,研究论文发展脉络
Chat Paper
正在生成论文摘要