Automated Evaluation of the Left-Sided Heart Volumes Using Machine Learning During Stress Echocardiography: a Multicenter Stress Echo 2030 Study
European heart journal Cardiovascular imaging(2023)
摘要
Funding Acknowledgements Type of funding sources: Public grant(s) – EU funding. Main funding source(s): HORIZON. Introduction Stress echocardiography (SE) is a recognized method for risk assessment and detection of coronary artery disease. Although left atrial (LA) and left ventricle (LV) volumes provide valuable information during SE, the time-consuming manual tracings of these structures and operator-dependent accuracy remain significant limitations in analyzing and interpreting SE that might be overcome by automated evaluation. Purpose To assess the potential of an automated machine learning (ML) system for the volumetric evaluation of left-sided heart volumes during SE. Methods The study enrolled 218 participants from five recruiting centers who underwent SE (in most cases, dipyridamole SE). Imaging data were acquired in DICOM format and anonymized for further processing. The studies were evaluated by an expert cardiologist trained in SE evaluation who selected apical four-chamber (4Ch) and two-chamber (2Ch) view images during stress and rest phases an marked end-systolic (ES) and end-diastolic (ED) frames and traced endocardial borders for LA (in ES) and LV (in ED and ES). The same frames were then processed by a transformer neural network trained on a separate set of images. LA volume (LAV), LV end-diastolic volume (LVEDV), LV end-systolic volume (LVESV), and LV ejection fraction (LVEF) was calculated in 4Ch and 2Ch views during rest and at stress phases. Each evaluator (cardiologist and ML model) was blinded from each other's measurements. All of the tracings and automated measurements were performed on the same platform. Results Comparison between evaluators by Student's paired t-test showed the best agreement between LAV (4Ch) and LVESV (2Ch) during the rest phase and LVEDV (4Ch and 2Ch) during the stress phase (p>0.05). Other volumetric measurements tended to be slightly higher (except LVEDVs at rest) and LVEF lower assessed by ML than by cardiologists. Pearson's correlation analysis demonstrated strong correlations between the ML model and cardiologist for LAVs (4Ch LAV r=0.94, p<0.001 and r=0.93, p<0.001, 2Ch LAV r=0.91, p<0.001 and r=0.88, p<0.001 at rest and stress, respectively), and LV volumes (4Ch LVEDV r=0.86, p<0.001 and r=0.84, p<0.001, 2Ch LVEDV r=0.82, p<0.001 and r=0.93, p<0.001, 4Ch LVESV r=0.82, p<0.001 and r=0.83, p<0.001, 2Ch LVESV r=0.86, p<0.001 and r=0.82, p<0.001 at rest and stress, respectively), and moderate correlations for LVEF measurements during rest and stress phases. The strongest correlations between raters and the smallest root mean squared errors (RMSEs) were for LAV measurements. Conclusions This multicenter study showed the effectiveness of using ML to produce left-sided heart measurements during SE. There was a tendency for higher volumes assessed by ML, demonstrating the need for additional standardization of SE evaluation for more precise accuracy. However, correlations between the ML model and cardiologists' measurements for left-sided heart volumes were strong during the rest and the stress phases.
更多查看译文
AI 理解论文
溯源树
样例
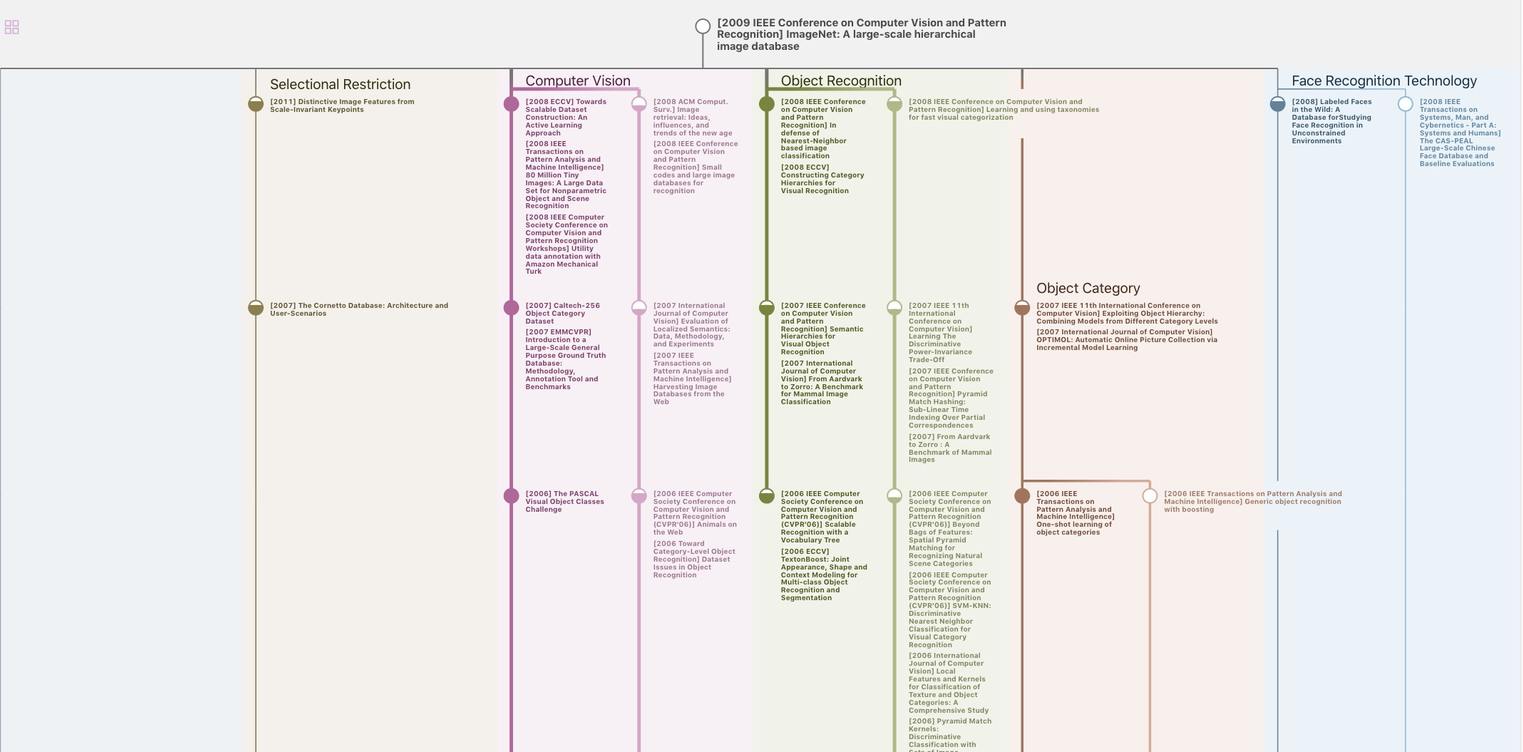
生成溯源树,研究论文发展脉络
Chat Paper
正在生成论文摘要