High-Definition Image Formation Using Multi-stage Cycle Generative Adversarial Network with Applications in Image Forensic
Arabian journal for science and engineering(2023)
摘要
In the modern world, human safety and crime control are daunting tasks. Each year the number of street crime cases has been increasing. In many cases, the culprit is unknown, in which the challenging task is to identify the right culprit possibly among hundreds of options in contexts of densely populated public spaces like target killing, vehicle snatching, etc. Often, in these situations, a sketch artist, working for the police forensic department, produces a drawing of the culprit’s face based on guidelines from the victims or witnesses to the crime. However, hand-drawn sketches can be an inefficient means for matching facial photographs of the culprit, particularly in cases where the software is designed around using images of real faces. In this research work, a novel technique is proposed to generate hyperreal high-definition (H-HD) face images of the culprit from a single hand-drawn face sketch. ’Hyperreal’ is used here in the way it is used in the Arts, as in making the image, albeit based on a person’s thoughts, truer to reality through a deep understanding of how the real subject would appear. To produce this image translation from sketch to H-HD face, two techniques are presented in this article, namely cycle generative adversarial network (CGAN) and multistage cycle generative adversarial network (MS-CGAN). MS-CGAN has multiple layers as stages and produces minimum cycle consistency and generative adversarial losses. CGAN uses paired data for training whereas MS-CGAN uses unpaired data. The training results show that the MSE loss of the proposed technique is found to be less than CGANs. GANs can be evaluated in three ways, namely qualitative, quantitative, and observational. In this paper, a quantitative comparison is made by the evaluation of CGAN and MS-CGAN based on the pixel-to-pixel comparison. An observational analysis is performed on the feedback from the observers. According to the evaluations, 54% of participants voted for the MS-CGAN whereas 46% rated CGAN to be the better performer. Two types of pixel-to-pixel comparisons are performed: color-to-color comparison and sketch-to-sketch comparison in terms of mean square error (MSE) and root mean square error (RMSE). For color-to-color image comparison, CGAN achieved an MSE of 2.312 and RMSE of 1.521 whereas MS-CGAN achieved an MSE of 2.232 and RMSE of 1.494. For sketch-to-sketch pixel comparison, CGAN achieved an MSE of 1.901 and RMSE of 1.379 whereas MS-CGAN achieved an MSE of 1.81 and 1.345 RMSE. The development of MS-CGAN and the research of this article are aimed at the police forensic department to generate a true-to-life H-HD face of a culprit and thereby contribute toward the overarching goal of maintaining a peaceful society.
更多查看译文
关键词
Generative adversarial network,Multistage cycle generative adversarial network,Face sketch,Image forensic,Image translation,Smart cities
AI 理解论文
溯源树
样例
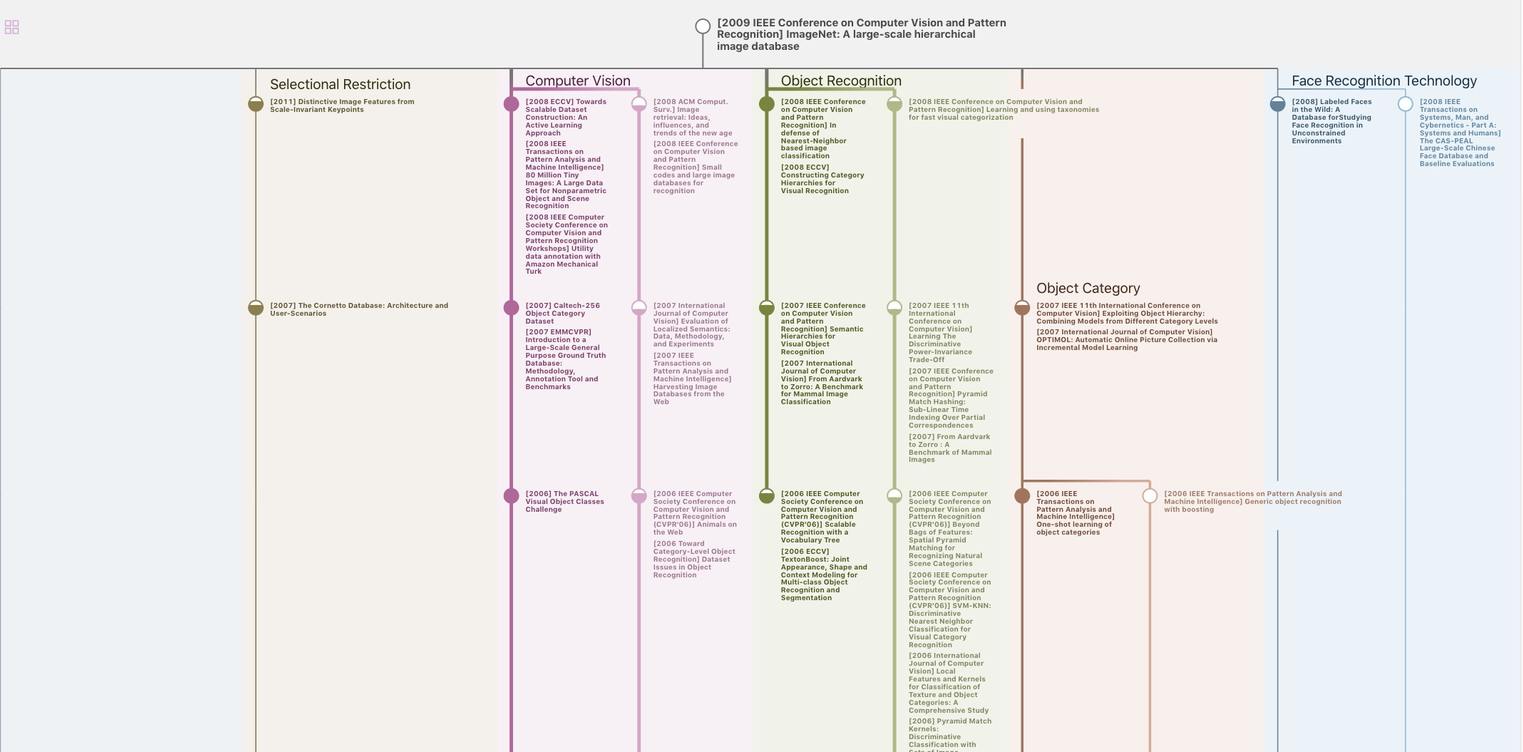
生成溯源树,研究论文发展脉络
Chat Paper
正在生成论文摘要